Aop: An Anti-Overfitting Pretreatment For Practical Image-Based Plant Diagnosis
2019 IEEE INTERNATIONAL CONFERENCE ON BIG DATA (BIG DATA)(2019)
摘要
In image-based plant diagnosis, clues related to diagnosis are often unclear, and the other factors such as image backgrounds often have a significant impact on the final decision. As a result, overfitting due to latent similarities in the dataset often occurs, and the diagnostic performance on real unseen data (e,g. images from other farms) is usually dropped significantly. However, this problem has not been sufficiently explored, since many systems have shown excellent diagnostic performance due to the bias caused by the similarities in the dataset. In this study, we investigate this problem with experiments using more than 50,000 images of cucumber leaves, and propose an anti-overfitting pretreatment (AOP) for realizing practical image-based plant diagnosis systems. The AOP detects the area of interest (leaf, fruit etc.) and performs brightness calibration as a preprocessing step. The experimental results demonstrate that our AOP can improve the accuracy of diagnosis for unknown test images from different farms by 12.2% in a practical setting.
更多查看译文
关键词
automated plant diagnosis, preprocessing, segmentation
AI 理解论文
溯源树
样例
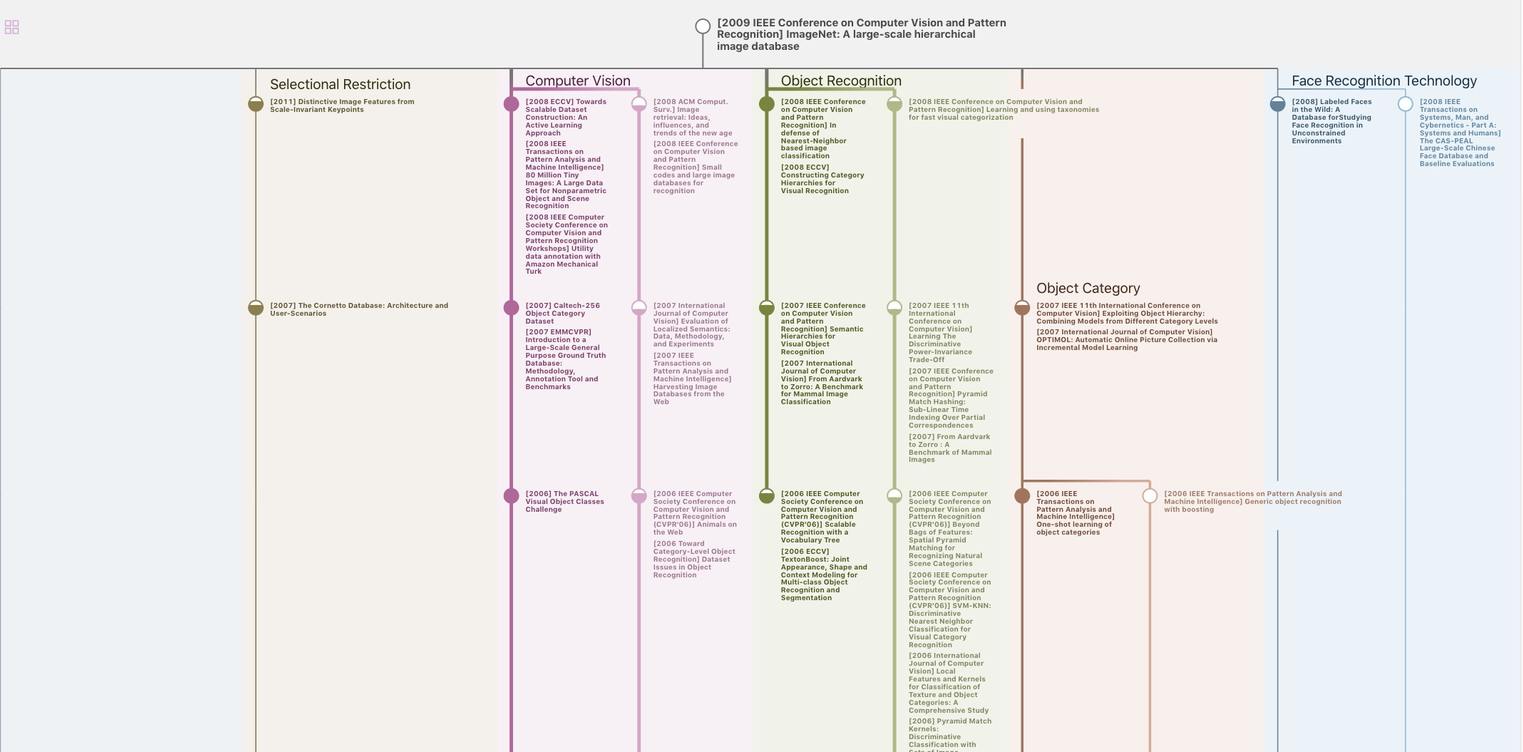
生成溯源树,研究论文发展脉络
Chat Paper
正在生成论文摘要