NAViDAd: A No-Reference Audio-Visual Quality Metric Based on a Deep Autoencoder
2019 27TH EUROPEAN SIGNAL PROCESSING CONFERENCE (EUSIPCO)(2020)
摘要
The development of models for quality prediction of both audio and video signals is a fairly mature field. But, although several multimodal models have been proposed, the area of audio-visual quality prediction is still an emerging area. In fact, despite the reasonable performance obtained by combination and parametric metrics, currently there is no reliable pixel-based audio-visual quality metric. The approach presented in this work is based on the assumption that autoencoders, fed with descriptive audio and video features, might produce a set of features that is able to describe the complex audio and video interactions. Based on this hypothesis, we propose a No-Reference Audio-Visual Quality Metric Based on a Deep Autoencoder (NAViDAd). The model visual features are natural scene statistics (NSS) and spatial-temporal measures of the video component. Meanwhile, the audio features are obtained by computing the spectrogram representation of the audio component. The model is formed by a 2-layer framework that includes a deep autoencoder layer and a classification layer. These two layers are stacked and trained to build the deep neural network model. The model is trained and tested using a large set of stimuli, containing representative audio and video artifacts. The model performed well when tested against the UnB-AV and the LiveNetflix-II databases. that this type of approach produces quality scores that are highly correlated to subjective quality scores.
更多查看译文
关键词
audio-visual, quality metrics, no-reference, distortions, autoencoder, NAViDAd
AI 理解论文
溯源树
样例
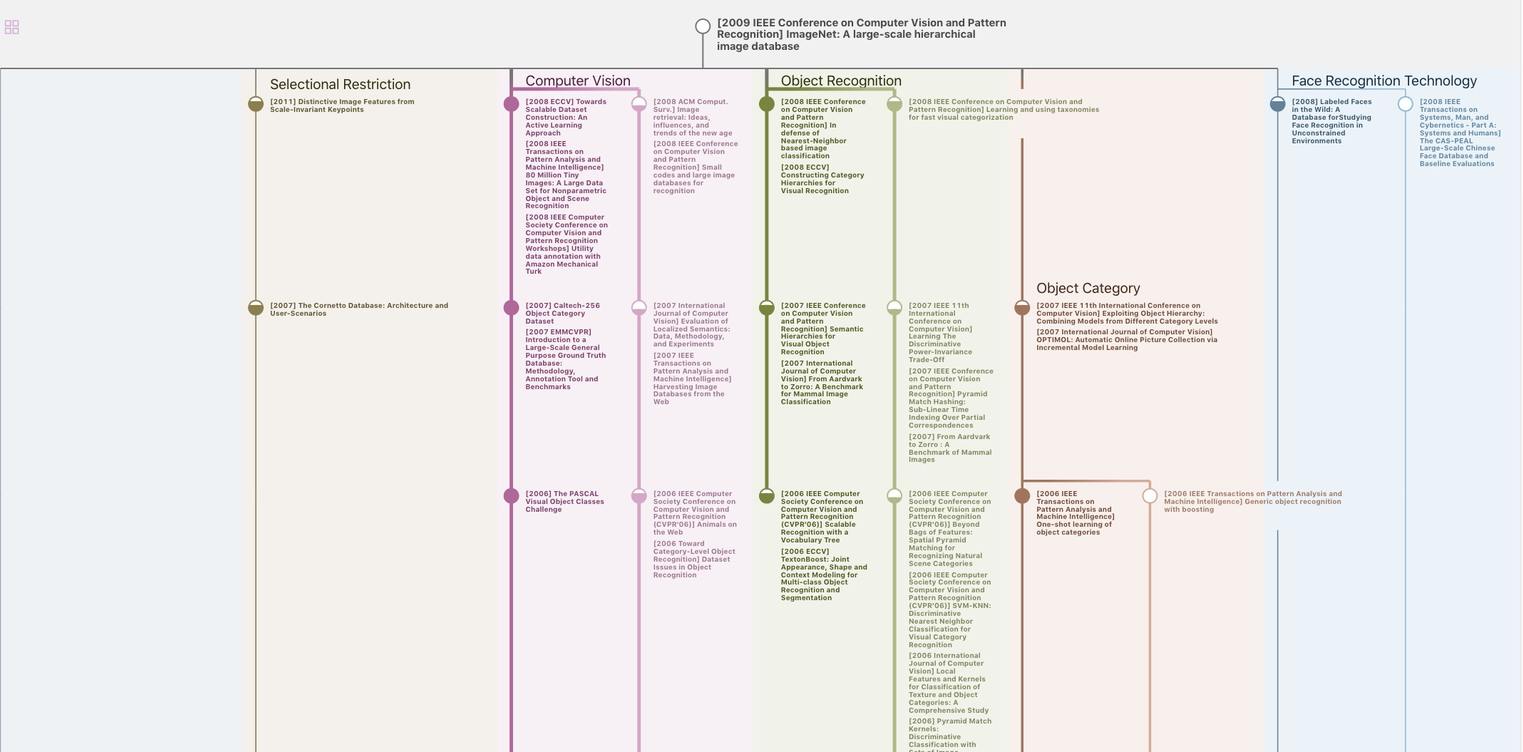
生成溯源树,研究论文发展脉络
Chat Paper
正在生成论文摘要