One Man’s Trash Is Another Man’s Treasure: Resisting Adversarial Examples by Adversarial Examples
2020 IEEE/CVF Conference on Computer Vision and Pattern Recognition (CVPR)(2020)
摘要
Modern image classification systems are often built on deep neural networks, which suffer from adversarial examples--images with deliberately crafted, imperceptible noise to mislead the network's classification. To defend against adversarial examples, a plausible idea is to obfuscate the network's gradient with respect to the input image. This general idea has inspired a long line of defense methods. Yet, almost all of them have proven vulnerable. We revisit this seemingly flawed idea from a radically different perspective. We embrace the omnipresence of adversarial examples and the numerical procedure of crafting them, and turn this harmful attacking process into a useful defense mechanism. Our defense method is conceptually simple: before feeding an input image for classification, transform it by finding an adversarial example on a pre-trained external model. We evaluate our method against a wide range of possible attacks. On both CIFAR-10 and Tiny ImageNet datasets, our method is significantly more robust than state-of-the-art methods. Particularly, in comparison to adversarial training, our method offers lower training cost as well as stronger robustness.
更多查看译文
关键词
Tiny ImageNet datasets,CIFAR-10 datasets,modern image classification systems,adversarial training,input image,defense method
AI 理解论文
溯源树
样例
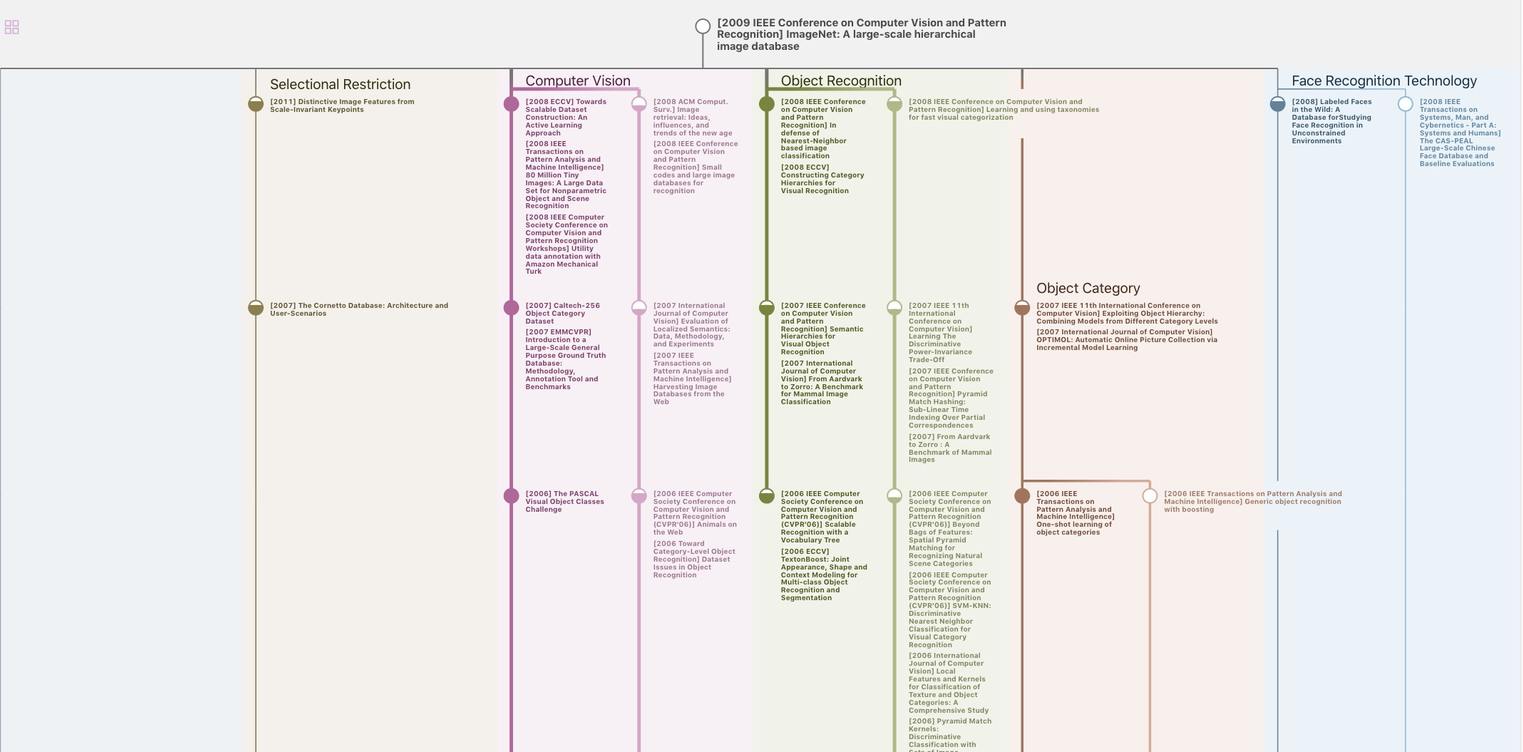
生成溯源树,研究论文发展脉络
Chat Paper
正在生成论文摘要