Shape Reconstruction By Learning Differentiable Surface Representations
2020 IEEE/CVF CONFERENCE ON COMPUTER VISION AND PATTERN RECOGNITION (CVPR)(2020)
摘要
Generative models that produce point clouds have emerged as a powerful tool to represent 3D surfaces, and the best current ones rely on learning an ensemble of parametric representations. Unfortunately, they offer no control over the deformations of the surface patches that form the ensemble and thus fail to prevent them from either overlapping or collapsing into single points or lines. As a consequence, computing shape properties such as surface normals and curvatures becomes difficult and unreliable.In this paper, we show that we can exploit the inherent differentiability of deep networks to leverage differential surface properties during training so as to prevent patch collapse and strongly reduce patch overlap. Furthermore, this lets us reliably compute quantities such as surface normals and curvatures. We will demonstrate on several tasks that this yields more accurate surface reconstructions than the state-of-the-art methods in terms of normals estimation and amount of collapsed and overlapped patches.
更多查看译文
关键词
patch overlap,surface normals,collapsed patches,overlapped patches,shape reconstruction,differentiable surface representations,generative models,point clouds,parametric representations,surface patches,shape properties,patch collapse,deep networks
AI 理解论文
溯源树
样例
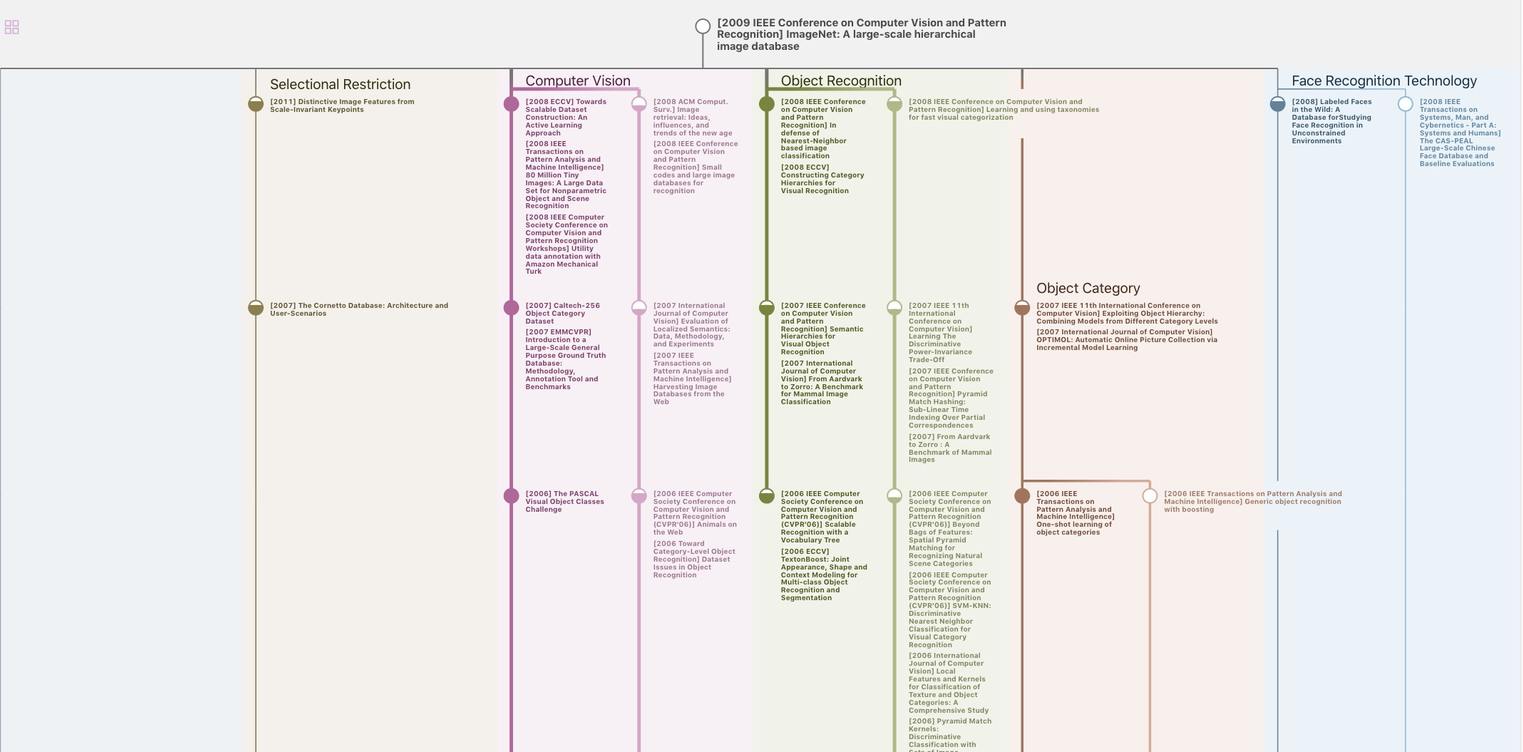
生成溯源树,研究论文发展脉络
Chat Paper
正在生成论文摘要