Deep Reinforcement Learning For Multi-Driver Vehicle Dispatching And Repositioning Problem
CoRR(2019)
摘要
Order dispatching and driver repositioning (also known as fleet management) in the face of spatially and temporally varying supply and demand are central to a ride-sharing platform marketplace. Hand-crafting heuristic solutions that account for the dynamics in these resource allocation problems is difficult, and may be better handled by an end-to-end machine learning method. Previous works have explored machine learning methods to the problem from a high-level perspective, where the learning method is responsible for either repositioning the drivers or dispatching orders, and as a further simplification, the drivers are considered independent agents maximizing their own reward functions. In this paper we present a deep reinforcement learning approach for tackling the full fleet management and dispatching problems. In addition to treating the drivers as individual agents, we consider the problem from a system-centric perspective, where a central fleet management agent is responsible for decision-making for all drivers.
更多查看译文
关键词
reinforcement learning, ride-sharing, fleet management, order dispatching
AI 理解论文
溯源树
样例
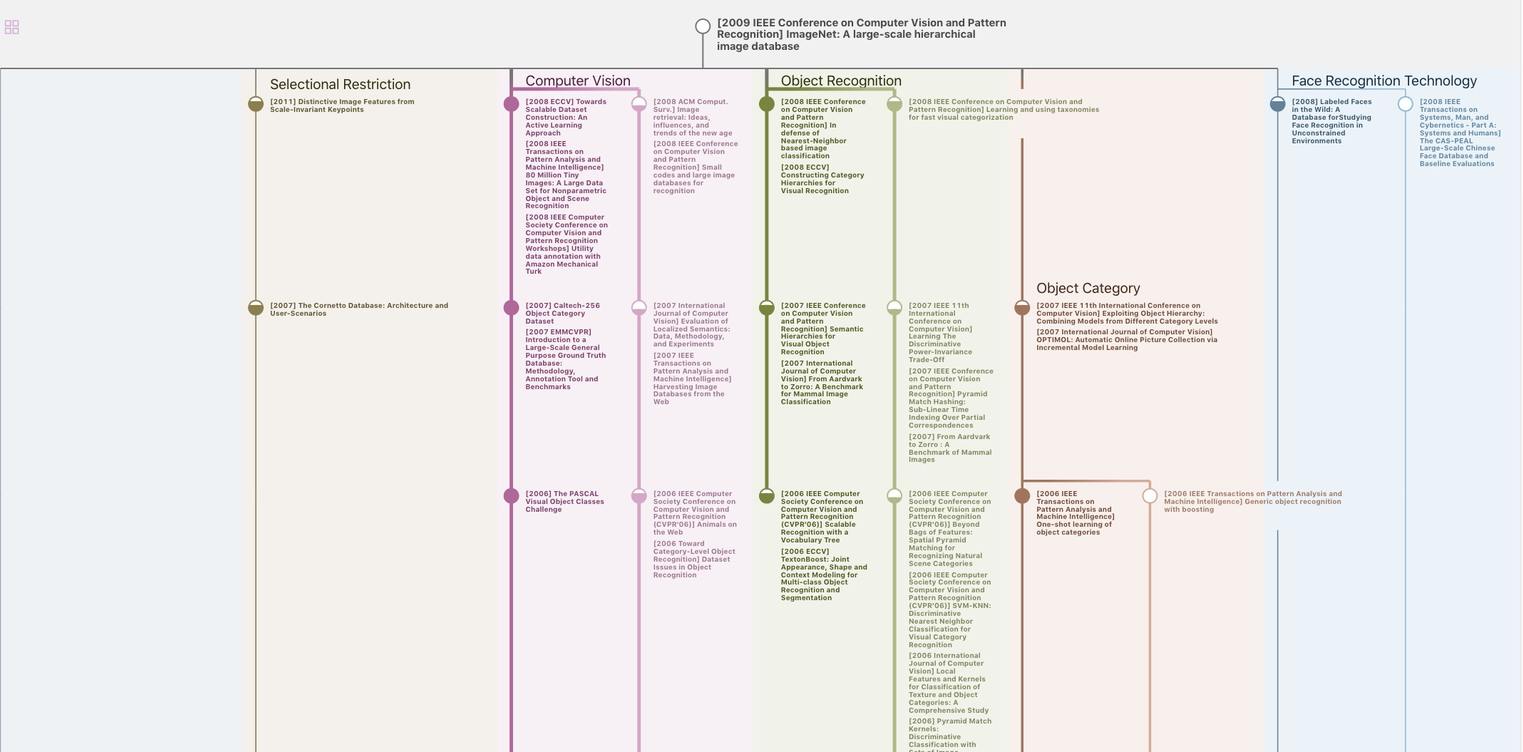
生成溯源树,研究论文发展脉络
Chat Paper
正在生成论文摘要