ViewAL: Active Learning with Viewpoint Entropy for Semantic Segmentation
CVPR(2020)
摘要
We propose ViewAL, a novel active learning strategy for semantic segmentation that exploits viewpoint consistency in multi-view datasets. Our core idea is that inconsistencies in model predictions across viewpoints provide a very reliable measure of uncertainty and encourage the model to perform well irrespective of the viewpoint under which objects are observed. To incorporate this uncertainty measure, we introduce a new viewpoint entropy formulation, which is the basis of our active learning strategy. In addition, we propose uncertainty computations on a superpixel level, which exploits inherently localized signal in the segmentation task, directly lowering the annotation costs. This combination of viewpoint entropy and the use of superpixels allows to efficiently select samples that are highly informative for improving the network. We demonstrate that our proposed active learning strategy not only yields the best-performing models for the same amount of required labeled data, but also significantly reduces labeling effort. For instance, our method achieves 95% of maximum achievable network performance using only 7%, 17%, and 24% labeled data on SceneNet-RGBD, ScanNet, and Matterport3D, respectively. On these datasets, the best state-of-the-art method achieves the same performance with 14%, 27% and 33% labeled data. Finally, we demonstrate that labeling using superpixels yields the same quality of ground-truth compared to labeling whole images, but requires 25% less time.
更多查看译文
关键词
multiview datasets,model predictions,uncertainty measure,viewpoint entropy formulation,active learning strategy,uncertainty computations,segmentation task,maximum achievable network performance,ViewAL,semantic segmentation,viewpoint consistency,SceneNet-RGBD,ScanNet,Matterport3D
AI 理解论文
溯源树
样例
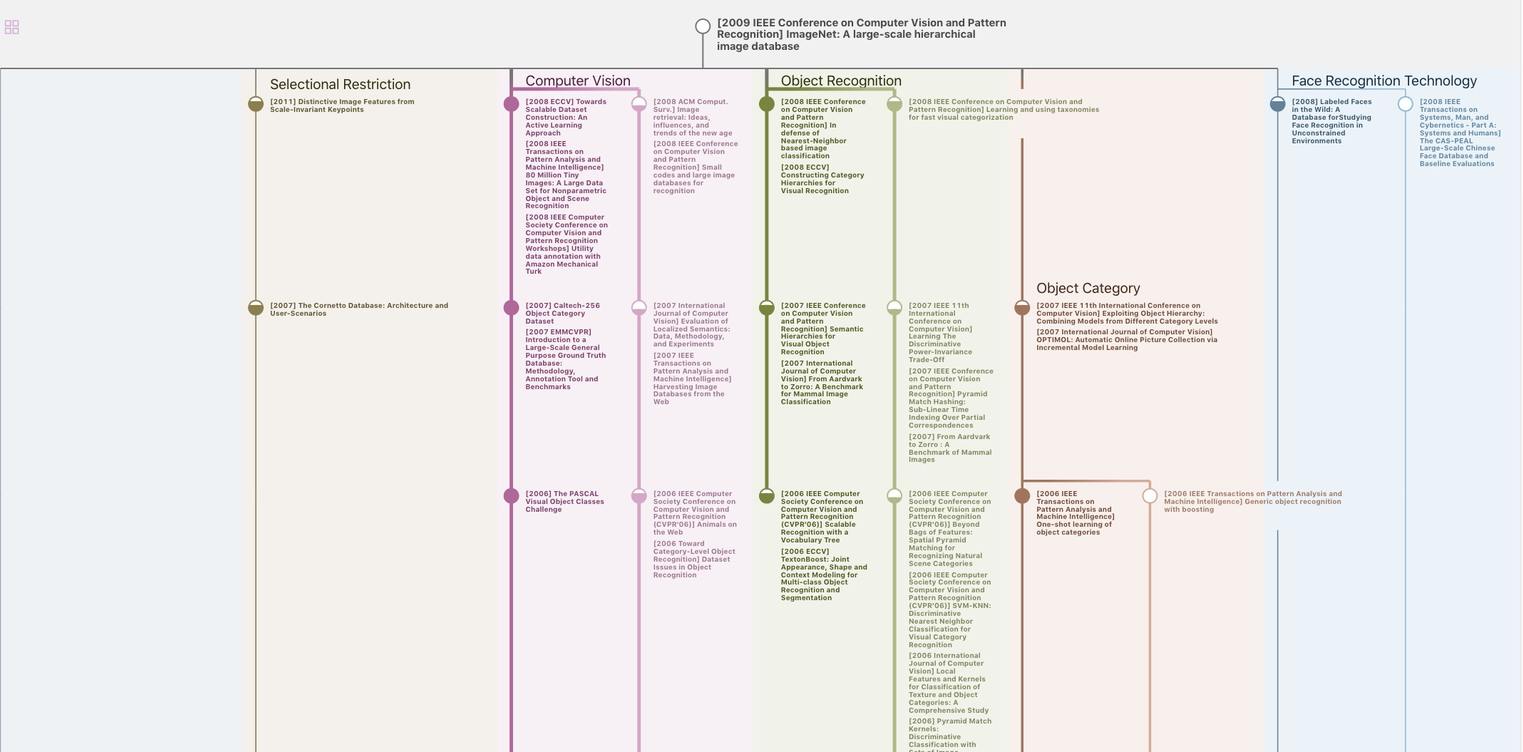
生成溯源树,研究论文发展脉络
Chat Paper
正在生成论文摘要