On the Minimal Set of Inputs Required for Efficient Neuro-Evolved Foraging
CoRR(2019)
摘要
In this paper, we perform an ablation study of \neatfa, a neuro-evolved foraging algorithm that has recently been shown to forage efficiently under different resource distributions. Through selective disabling of input signals, we identify a \emph{sufficiently} minimal set of input features that contribute the most towards determining search trajectories which favor high resource collection rates. Our experiments reveal that, independent of how the resources are distributed in the arena, the signals involved in imparting the controller the ability to switch from searching of resources to transporting them back to the nest are the most critical. Additionally, we find that pheromones play a key role in boosting performance of the controller by providing signals for informed locomotion in search for unforaged resources.
更多查看译文
AI 理解论文
溯源树
样例
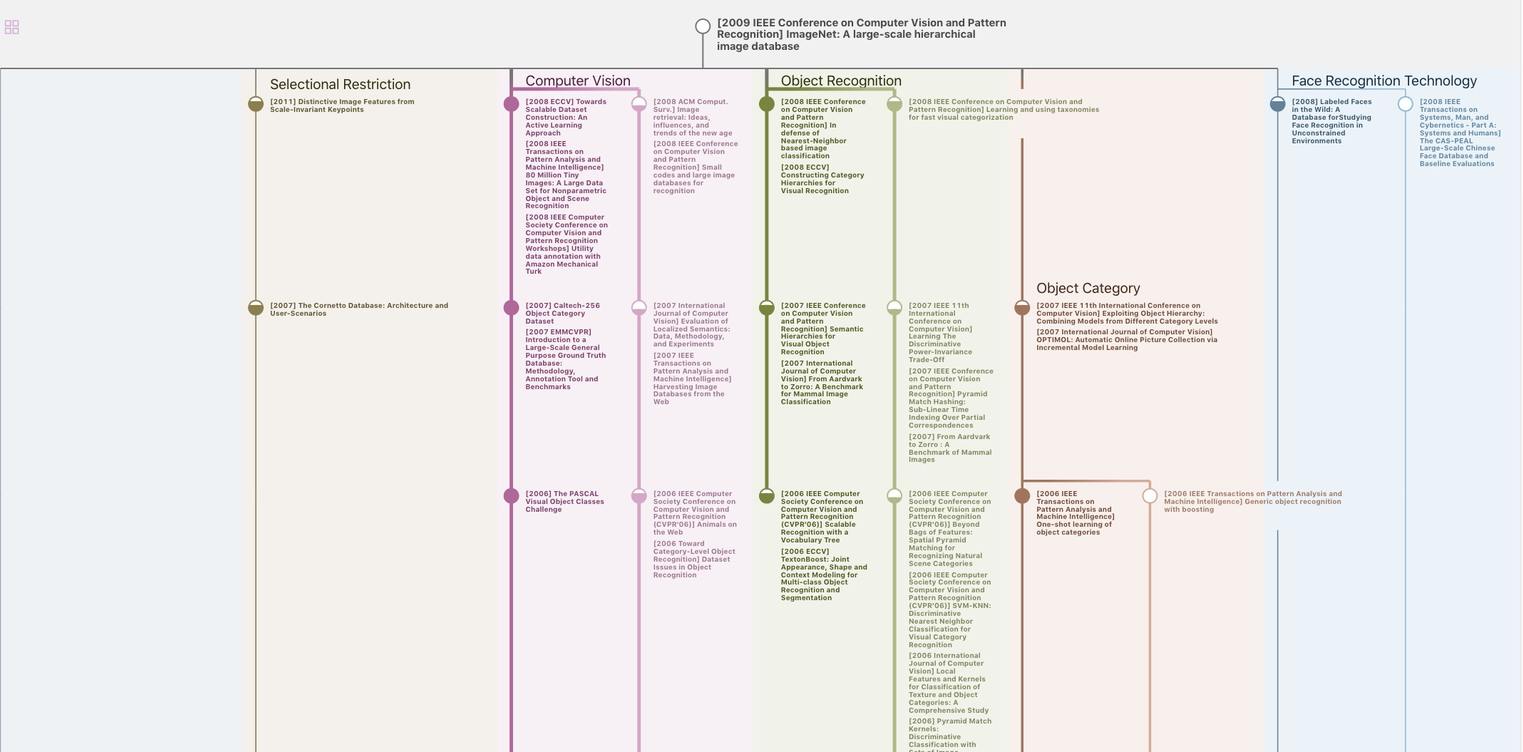
生成溯源树,研究论文发展脉络
Chat Paper
正在生成论文摘要