Orthogonal Convolutional Neural Networks
CVPR(2020)
摘要
The instability and feature redundancy in CNNs hinders further performance improvement. Using orthogonality as a regularizer has shown success in alleviating these issues. Previous works however only considered the kernel orthogonality in the convolution layers of CNNs, which is a necessary but not sufficient condition for orthogonal convolutions in general. We propose orthogonal convolutions as regularizations in CNNs and benchmark its effect on various tasks. We observe up to 3% gain for CIFAR100 and up to 1% gain for ImageNet classification. Our experiments also demonstrate improved performance on image retrieval, inpainting and generation, which suggests orthogonal convolution improves the feature expressiveness. Empirically, we show that the uniform spectrum and reduced feature redundancy may account for the gain in performance and robustness under adversarial attacks.
更多查看译文
关键词
convolutional layer,doubly block-Toeplitz matrix representation,convolutional kernel,common kernel orthogonality approach,orthogonal convolution,kernel orthogonality alternative,orthogonal convolutional neural networks,training instability,feature redundancy,convolutional filters,filter orthogonality
AI 理解论文
溯源树
样例
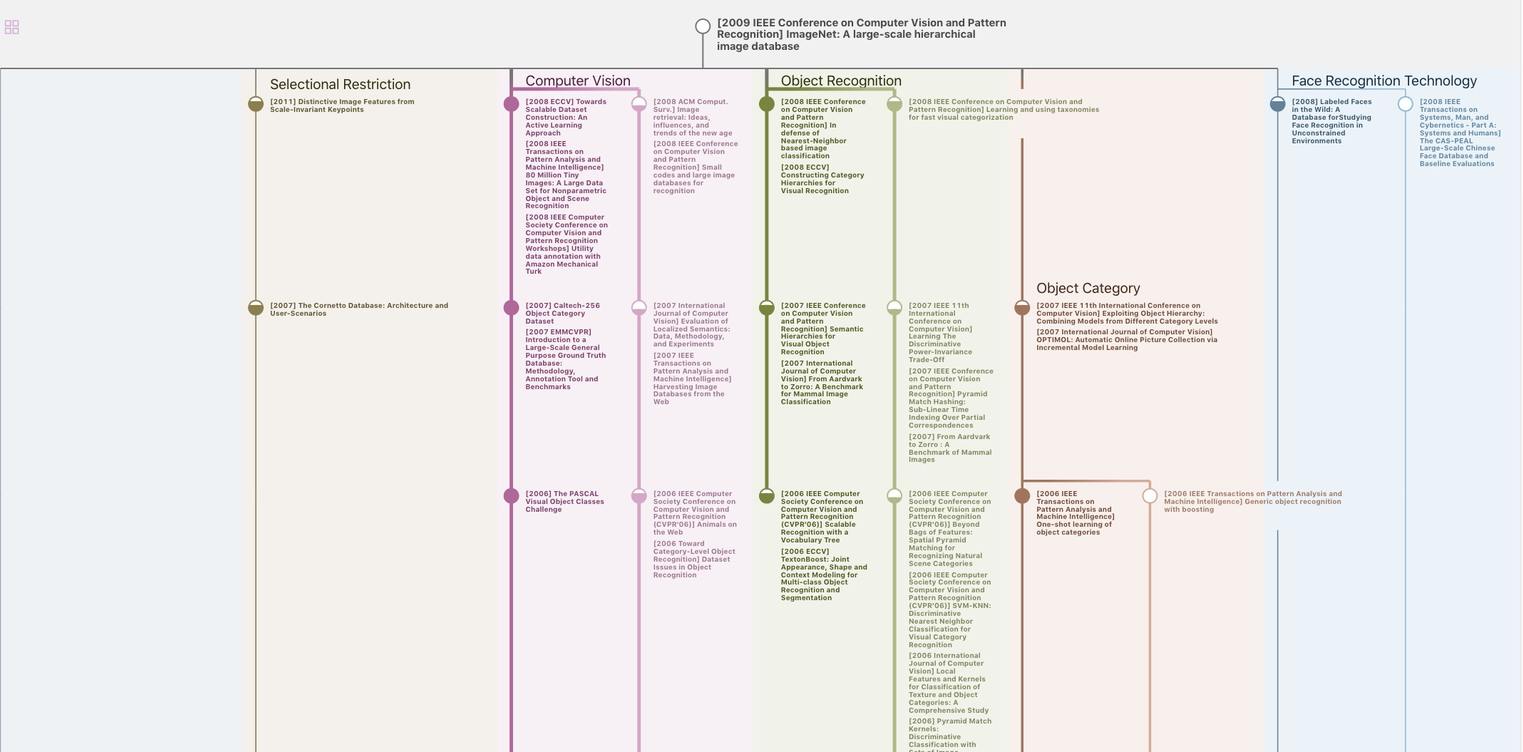
生成溯源树,研究论文发展脉络
Chat Paper
正在生成论文摘要