Implementation and performance evaluation of a communication-avoiding GMRES method for stencil-based code on GPU cluster
The Journal of Supercomputing(2019)
摘要
In this study, a communication-avoiding generalized minimum residual method (CA-GMRES) is implemented on a hybrid CPU–GPU cluster, targeted for the performance acceleration of iterative linear system solver in the gyrokinetic toroidal five-dimensional Eulerian code (GT5D). In the GT5D, its sparse matrix-vector multiplication operation (SpMV) is performed as a 17-point stencil-based computation. The specialized part for the GT5D is only in the SpMV, and the other parts are usable also for other application program codes. In addition to the CA-GMRES, we implement and evaluate a modified variant of CA-GMRES (M-CA-GMRES) proposed in the previous study Idomura et al. (in: Proceedings of the 8th workshop on latest advances in scalable algorithms for large-scale systems (ScalA ’17), 2017. https://doi.org/10.1145/3148226.3148234 ) to reduce the amount of floating-point calculations. This study demonstrates that beneficial features of the CA-GMRES are in its minimum number of collective communications and its highly efficient calculations based on dense matrix–matrix operations. The performance evaluation is conducted on the Reedbush-L GPU cluster, which contains four NVIDIA Tesla P100 (Pascal GP100) GPUs per compute node. The evaluation results show that the M-CA-GMRES or CA-GMRES for the GT5D is advantageous over the GMRES or the generalized conjugate residual method (GCR) on GPU clusters, especially when the problem size (vector length) is large so that the cost of the SpMV is less dominant. The M-CA-GMRES is 1.09 ×, 1.22 × and 1.50 × faster than the CA-GMRES, GCR and GMRES, respectively, when 64 GPUs are used.
更多查看译文
关键词
Communication-avoiding Krylov subspace method, GMRES, Tall-skinny QR factorization, Stencil-based computation, GPU cluster
AI 理解论文
溯源树
样例
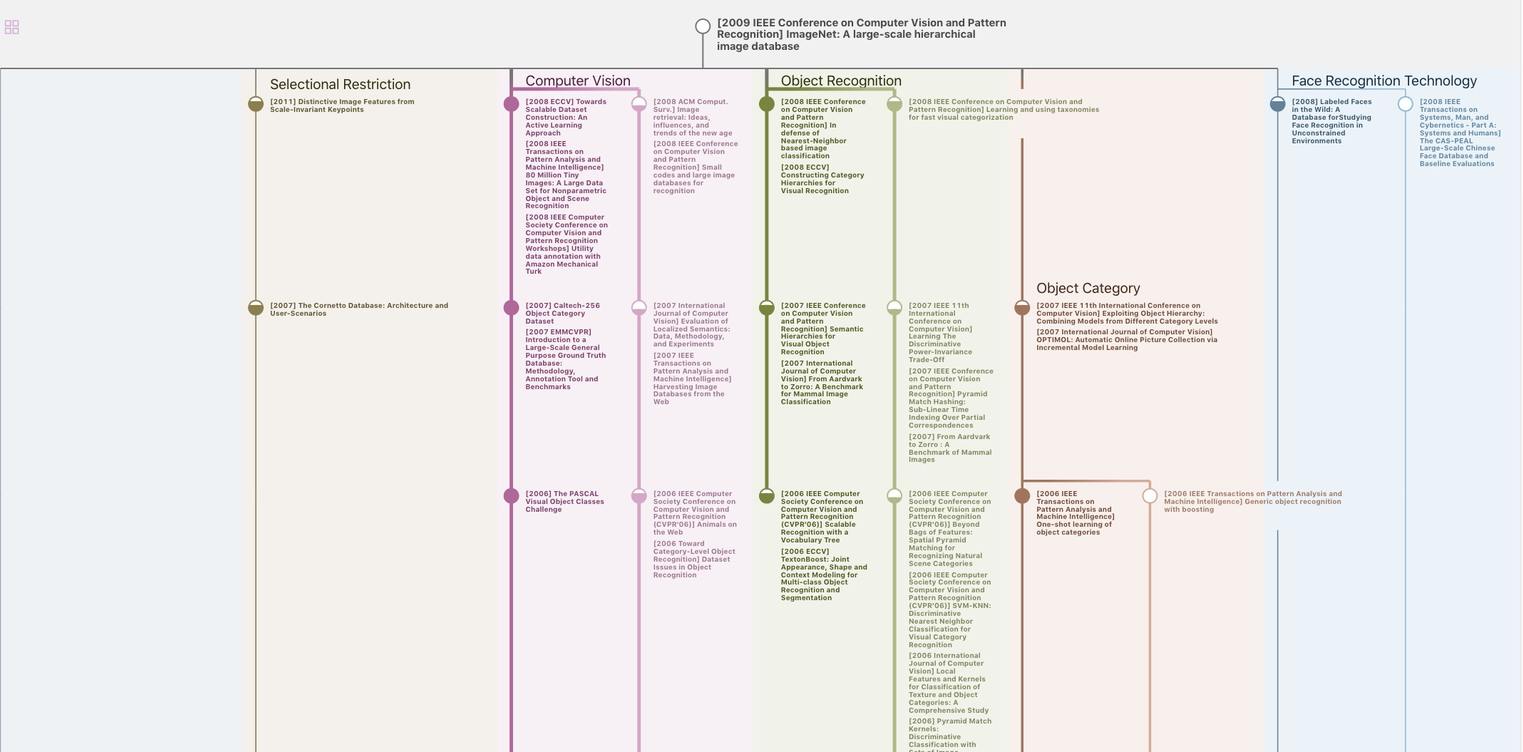
生成溯源树,研究论文发展脉络
Chat Paper
正在生成论文摘要