Effective image super resolution via hierarchical convolutional neural network.
Neurocomputing(2020)
摘要
An extensive amount of research work has been carried out in image super-resolution using convolutional neural networks. The focus of a significant number of reported approaches is primarily on either increasing the depth or the width of the networks to achieve improvements in performance. This paper proposes a novel hierarchical convolutional neural network (HCNN) for effective image super-resolution by learning features from different levels. More specifically, the proposed framework implements a 3-step hierarchical process, which consists of an edge extraction branch, an edge reinforcement branch, and an image reconstruction branch. Informative edges in an image are extracted and enhanced in the edge extraction and reinforcement branch, which are used as a guidance in the image reconstruction branch. Experimental results on several public datasets demonstrate that the proposed framework can restore high-frequency edges information and achieve superior performances over the state-of-the-art methods. Moreover, through a case study in facial expression recognition, we show that the enhanced images are beneficial in improving the recognition performance, paving the way for more practical applications.
更多查看译文
关键词
Image super-resolution,Edges extraction,Deep learning,Facial expression recognition
AI 理解论文
溯源树
样例
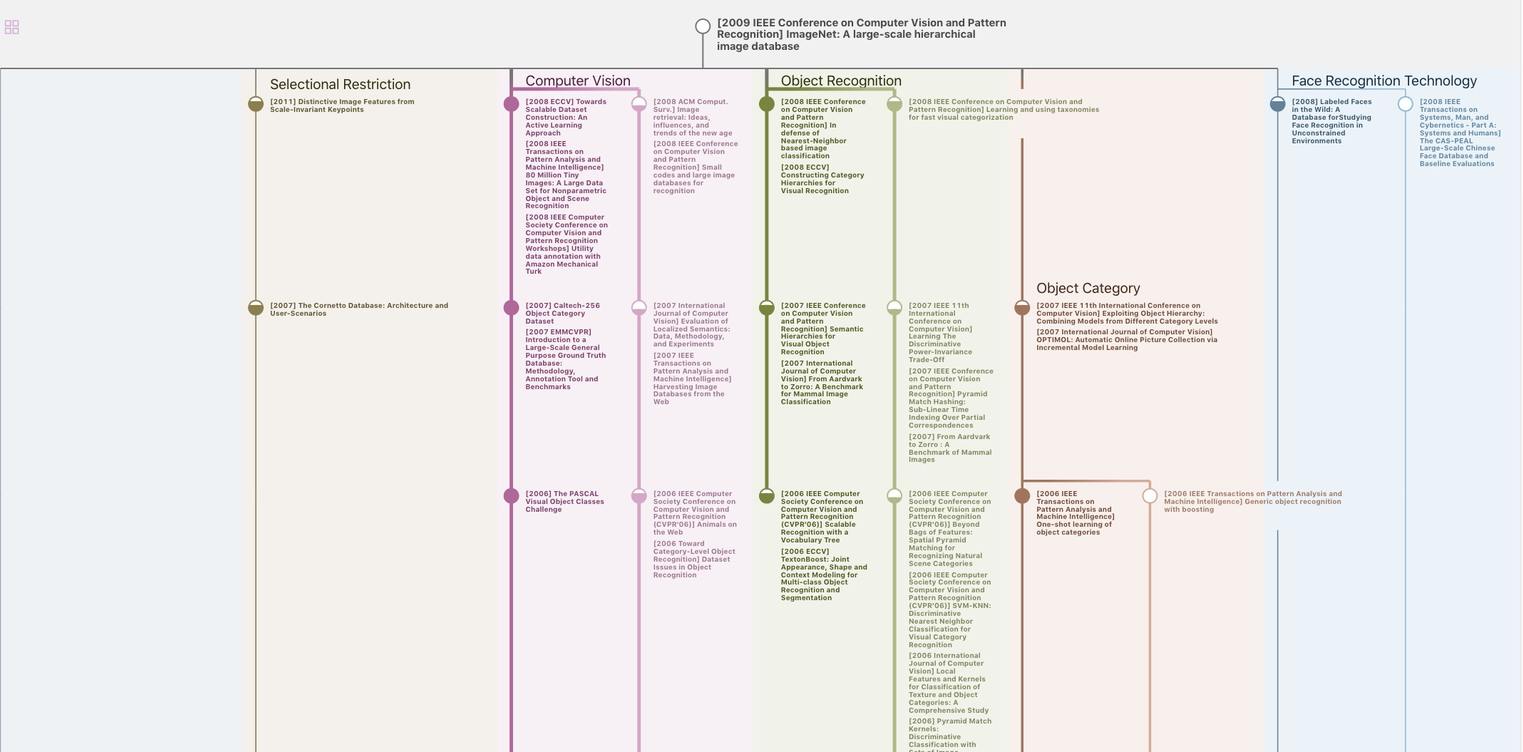
生成溯源树,研究论文发展脉络
Chat Paper
正在生成论文摘要