From Speech to Facial Activity: Towards Cross-modal Sequence-to-Sequence Attention Networks
2019 IEEE 21st International Workshop on Multimedia Signal Processing (MMSP)(2019)
摘要
Multimodal data sources offer the possibility to capture and model interactions between modalities, leading to an improved understanding of underlying relationships. In this regard, the work presented in this paper explores the relationship between facial muscle movements and speech signals. Specifically, we explore the efficacy of different sequence-to-sequence neural network architectures for the task of predicting Facial Action Coding System Action Units (AUs) from one of two acoustic feature representations extracted from speech signals, namely the extended Geneva Minimalistic Acoustic Parameter Set (eGeMAPs) or the Interspeech Computational Paralinguistics Challenge features set (ComParE). Furthermore, these architectures were enhanced by two different attention mechanisms (intra- and inter-attention) and various state-of-the-art network settings to improve prediction performance. Results indicate that a sequence-to-sequence model with inter-attention can achieve on average an Unweighted Average Recall (UAR) of 65.9 % for AU onset, 67.8 % for AU apex (both eGeMAPs), 79.7 % for AU offset and 65.3 % for AU occurrence (both ComParE) detection over all AUs.
更多查看译文
关键词
attention networks,facial action units,sequence to sequence,paralingustics
AI 理解论文
溯源树
样例
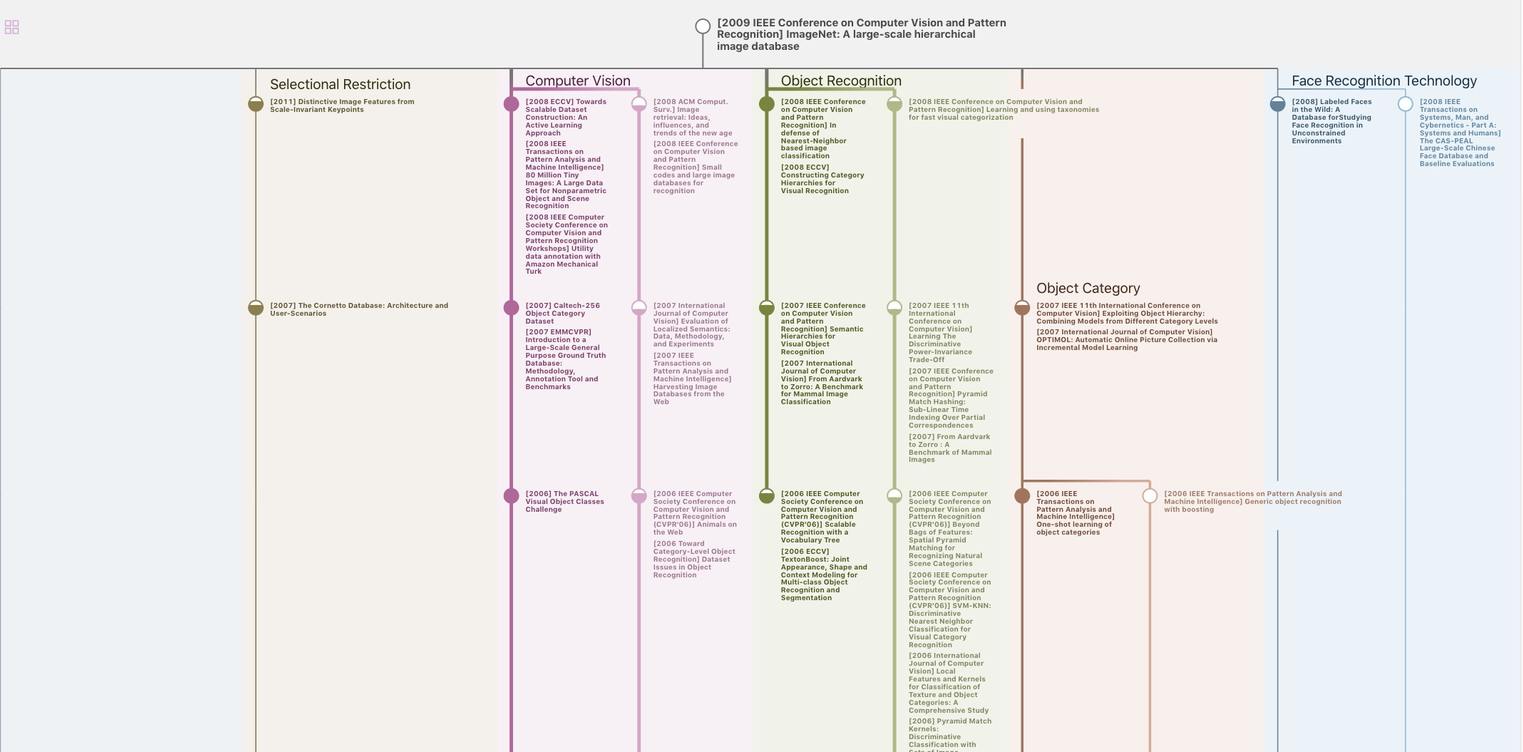
生成溯源树,研究论文发展脉络
Chat Paper
正在生成论文摘要