Estimating Quality of Service on Wi-Fi Stations Using Recurrent Neural Networks
2019 IEEE 30th Annual International Symposium on Personal, Indoor and Mobile Radio Communications (PIMRC)(2019)
摘要
Wireless networks are the most common way to access the Internet, with more than 10 billion Wi-Fi devices already sold. Wireless connections suffer from problems related to spectrum overuse, such as transmission errors and loss of information. Intelligent control systems can be used for network management and to improve Quality of Service (QoS). However, the first step towards such systems is a way to correlate current Wi-Fi readings to a QoS value. This work proposes a model using Recurrent Neural Networks (RNN) to infer such relation, based on real Wi-Fi data, and compares two RNN types: Gated Recurrent Unit (GRU) and Long Short Term Memory (LSTM). The model predicts four network metrics: throughput, loss, delay, and jitter, based only in traffic data obtained at the AP. The average Root Mean Square Error is of the order of 10
-2
for throughput, 10
-4
for delay, and 10
-5
for jitter and packet loss using both methods.
更多查看译文
关键词
Wi-Fi data,Gated Recurrent Unit,network metrics,average Root Mean Square Error,Wi-Fi Stations,Recurrent Neural Networks,wireless networks,Wi-Fi devices,wireless connections,spectrum overuse,transmission errors,intelligent control systems,network management,QoS value,Wi-Fi readings
AI 理解论文
溯源树
样例
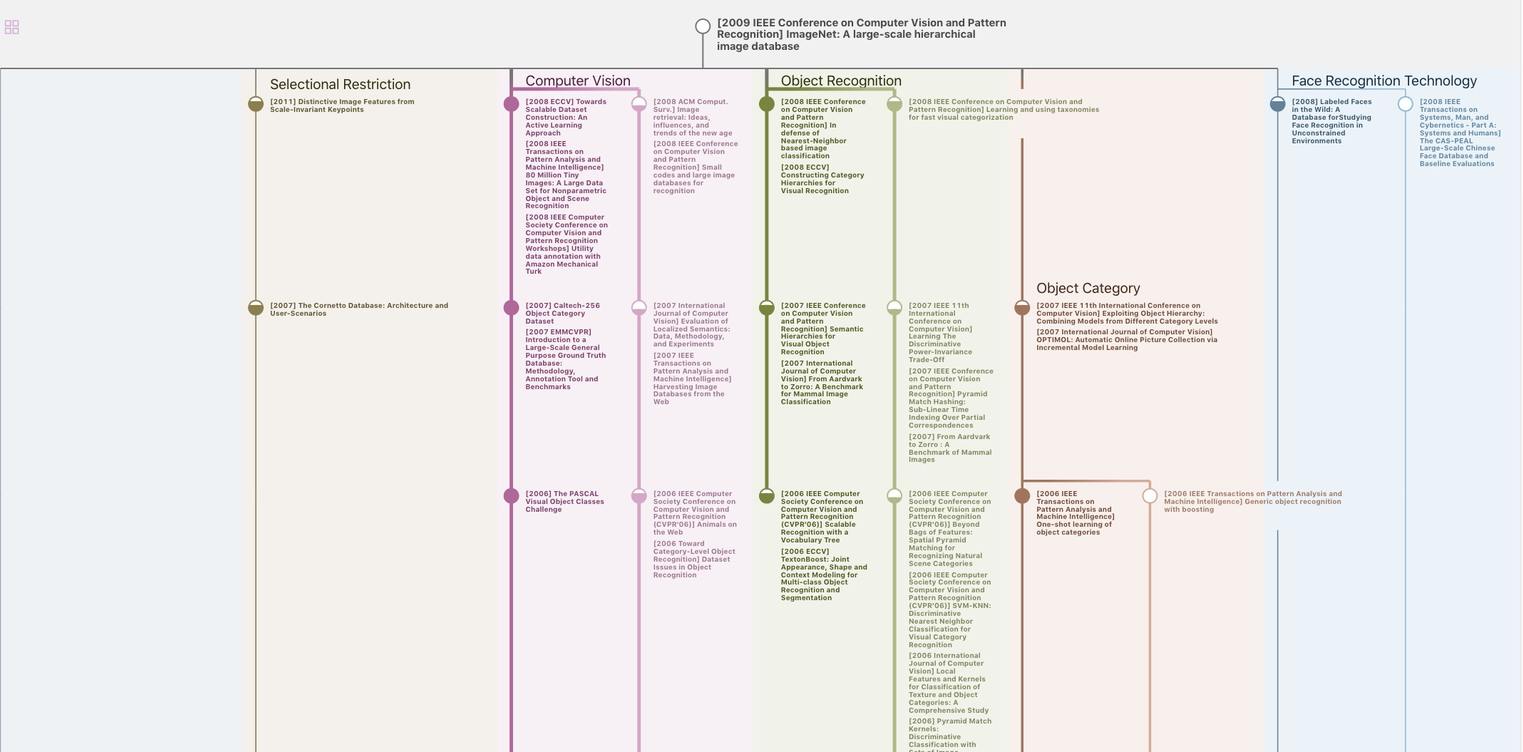
生成溯源树,研究论文发展脉络
Chat Paper
正在生成论文摘要