MACHINE LEARNING TO FACILITATE ASSESSMENT OF DIASTOLIC FUNCTION BY ECHOCARDIOGRAPHY
Canadian Journal of Cardiology(2019)
摘要
Echocardiographic assessment of diastolic function is complex but has important diagnostic and prognostic implications in the management of heart failure, particularly with preserved ejection fraction. The updated 2016 ASE/EACVI Recommendations for the Evaluation of Left Ventricular Diastolic Function by Echocardiography have proposed a less complex diagnostic algorithm. However, it continues to rely on grading of diastolic function based on category (normal, mild, moderate, severe, indeterminate), which provides modest correlation with hemodynamic measurements and remains echocardiographer-dependent. Whether machine learning can be used to derive a more precise diastolic function score has not been previously demonstrated. We screened 224,026 consecutive studies performed at a tertiary care centre from Dec 30, 1999 to Dec 31, 2015. We excluded studies containing features that preclude or complicate diastolic function assessment (stenotic, rheumatic or prosthetic mitral valves; more than moderate mitral regurgitation or MAC; non-sinus rhythm; confirmed or probable tamponade or constriction; more than trivial pericardial effusion; congenital heart disease; hypertrophic cardiomyopathy). We further excluded studies in which one or more parameters deemed essential for diastolic function assessment (age, EF, LAVi, TR velocity, E, lateral E’, septal E’, E/A, E/e') were not reported. We graded diastolic function for the remaining studies based on the ASE/EACVI 2016 diagnostic algorithm. Based on this grading, we trained a fully-connected 4-layer neural network (Figure 1A), blinded to the ASE/EACVI algorithm, to classify these studies by diastolic dysfunction severity. We then changed the final layer to create a neural network that would output a single continuous diastolic function score rather than a discrete categorization into diastolic function severities (Figure 1B). A total of 7,728 studies fulfilled inclusion and exclusion criteria. This was split into training (80%) and validation (20%) datasets. Our neural network reclassified the studies with 99.0% agreement to the ASE/EACVI diastolic function grading when tested on the validation dataset (Figure 1C). We generated a novel continuous score corresponding to overall diastolic function that corresponded well with diastolic function grading using the ASE/EACVI guidelines (Figure 1D). To our knowledge, this is the first study to use machine learning to generate a continuous diastolic function score that corresponds well with current recommendations of diastolic function grading.
更多查看译文
关键词
diastolic function,echocardiography,machine learning
AI 理解论文
溯源树
样例
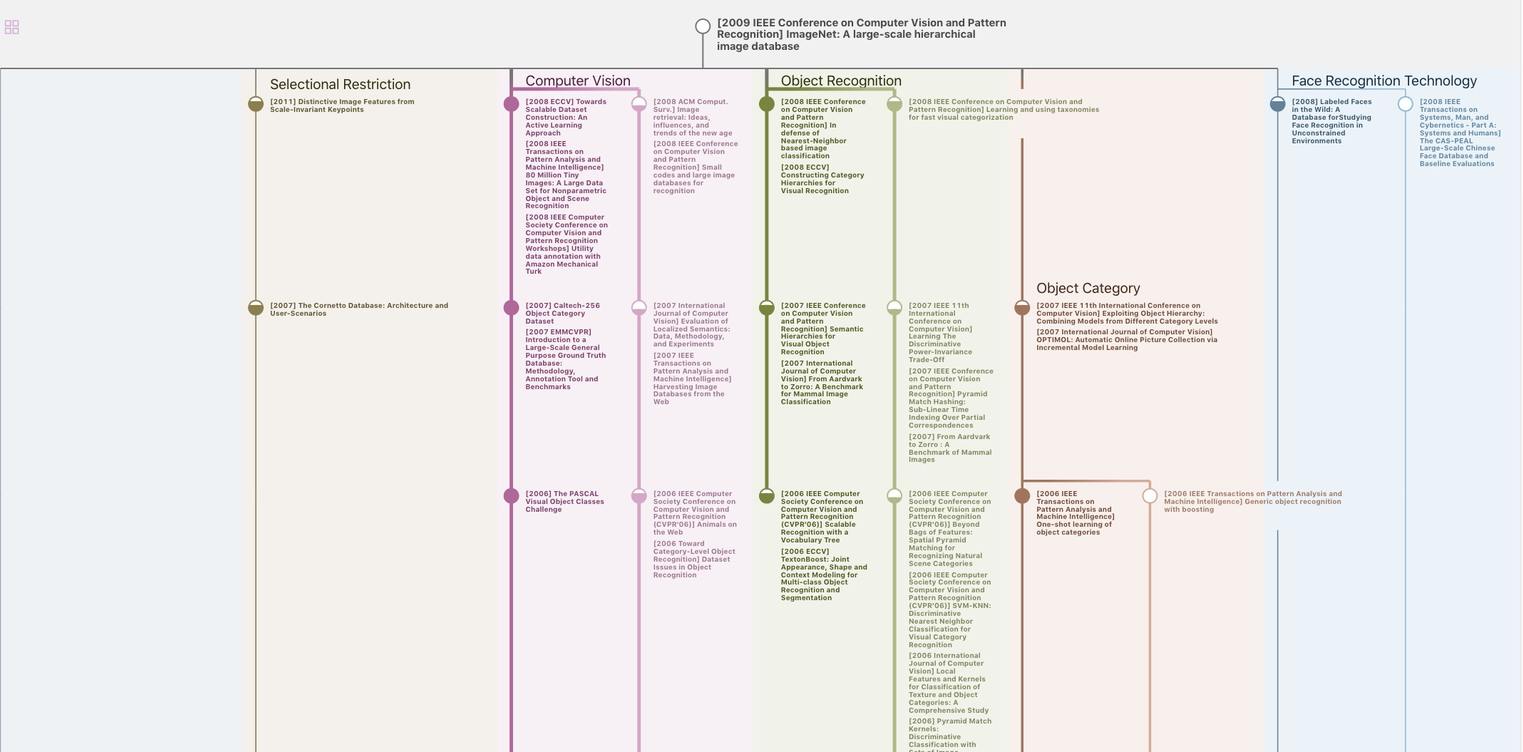
生成溯源树,研究论文发展脉络
Chat Paper
正在生成论文摘要