Small-scale moving target detection in aerial image by deep inverse reinforcement learning
Soft Computing(2019)
摘要
It proposes a deep inverse reinforcement learning method for slow and weak moving targets detection in aerial video. Differential gray images of adjacent frames are used as the network model input, and the feature network layer extracts the candidate moving target regions through the multi-layer convolution. The candidate target information is used as the initial layer of the policy network. The expert trajectory is used to adjust and optimize the feature convolution network model and the policy fully connected network model to realize the training the reward return function and the expert policy. In the stage of autonomous improvement policy, the policy model is re-optimized by unmarked aerial video, and deep inverse reinforcement learning and nonlinear policy network are used to make decision on moving target position and size information. The target size of the multi-group aerial video test set is 10 * 10 pixels. Experimental results show that the proposed algorithm has the advantage of the nonlinear policy of the neural network compared with the traditional moving target detection algorithm, and the detection result is more accurate. At the same time, compared with the traditional marginal programming (MMP) method and the structured classification based (SCIRL) method, the proposed algorithm shows obvious advantages in the accuracy of aerial video moving target detection.
更多查看译文
关键词
Aerial image, Deep inverse reinforcement, Small-scale target detection
AI 理解论文
溯源树
样例
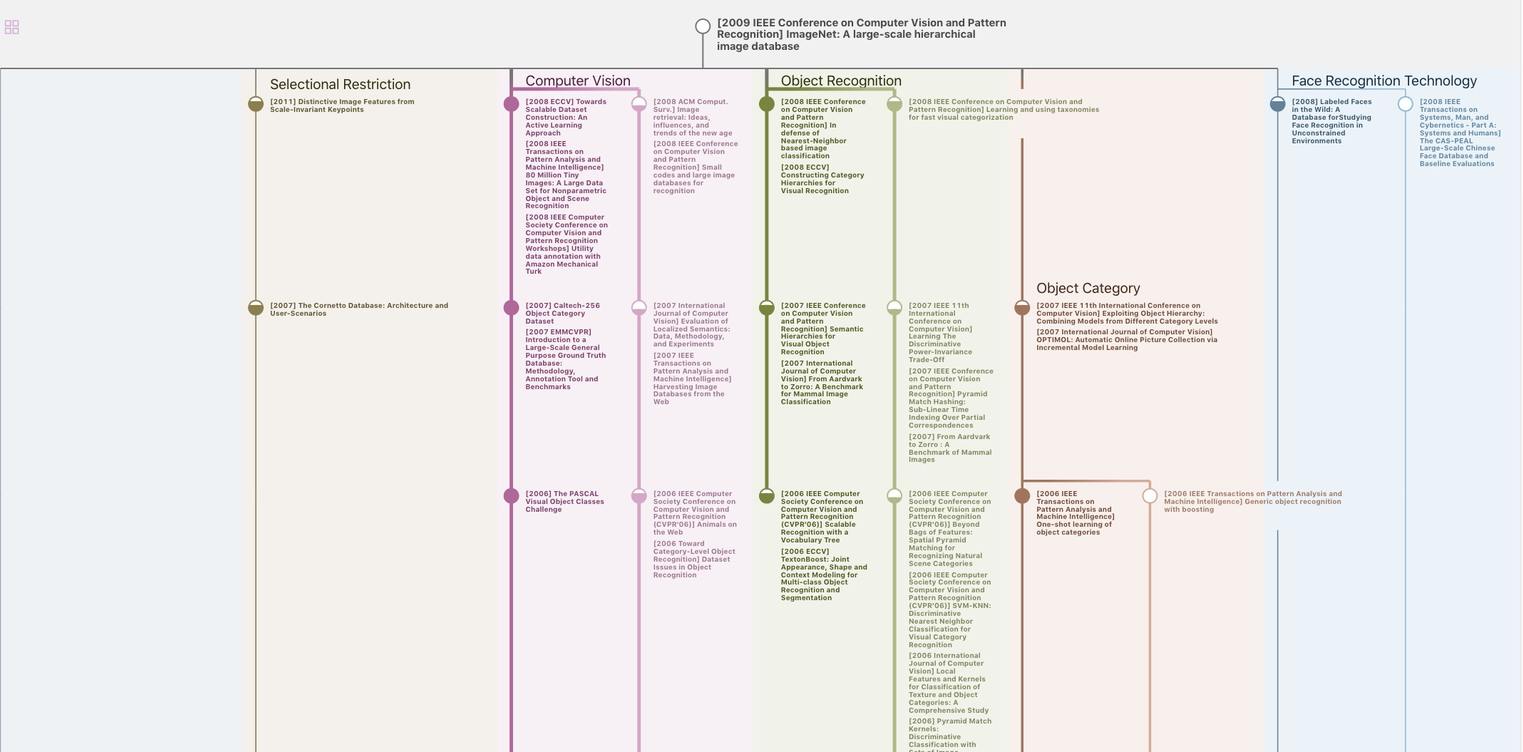
生成溯源树,研究论文发展脉络
Chat Paper
正在生成论文摘要