Unsupervised learning of monocular depth and ego-motion with space–temporal-centroid loss
International Journal of Machine Learning and Cybernetics(2019)
摘要
We propose DPCNN (Depth and Pose Convolutional Network), a novel framework for monocular depth with absolute scale and camera motion estimation from videos. DPCNN uses our proposed stereo training examples, in which the spatial and temporal images can be combined more closely, thus providing more priori constraint relationships. In addition, there are two significant features existing in DPCNN: One is that the entire space–temporal-centroid model is established to independently constrain the rotation matrix and the translation vector, so that the spatial and temporal images are collectively limited in a common, real-world scale. The other is to use the triangulation principle to establish a two-channel depth consistency loss, which penalizes inconsistency of the depths estimated from the spatial images and inconsecutive temporal images, respectively. Experiments on the KITTI datasets show that DPCNN achieves the most advanced results in both tasks and outperforms the current monocular methods.
更多查看译文
关键词
Deep learning,Depth estimation,Visual odometry (VO),3D feature transformation loss,Centroid transformation loss
AI 理解论文
溯源树
样例
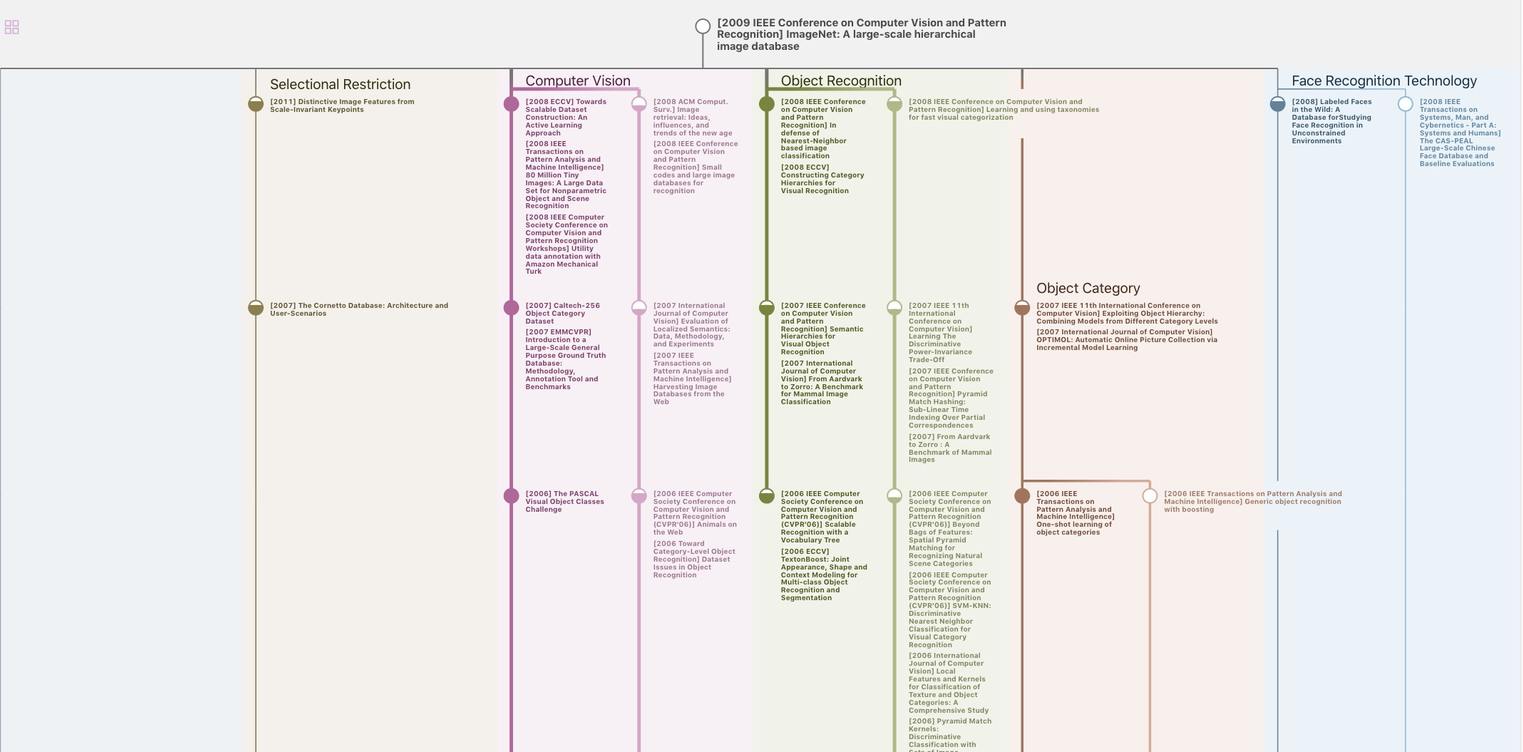
生成溯源树,研究论文发展脉络
Chat Paper
正在生成论文摘要