From Whole Slide Imaging to Microscopy: Deep Microscopy Adaptation Network for Histopathology Cancer Image Classification
Lecture Notes in Computer Science(2019)
摘要
Deep learning (DL) has achieved remarkable performance on digital pathology image classification with whole slide images (WSIs). Unfortunately, high acquisition costs of WSIs hinder the applications in practical scenarios, and most pathologists still use microscopy images (MSIs) in their workflows. However, it is especially challenging to train DL models on MSIs, given limited image qualities and high annotation costs. Alternatively, directly applying a WSI-trained DL model on MSIs usually performs poorly due to huge gaps between WSIs and MSIs. To address these issues, we propose to exploit deep unsupervised domain adaptation to adapt DL models trained on the labeled WSI domain to the unlabeled MSI domain. Specifically, we propose a novel Deep Microscopy Adaptation Network (DMAN). By reducing domain discrepancies via adversarial learning and entropy minimization, and alleviating class imbalance with sample reweighting, DMAN can classify MSIs effectively even without MSI annotations. Extensive experiments on colon cancer diagnosis demonstrate the effectiveness of DMAN and its potential in customizing models for each pathologist’s microscope.
更多查看译文
关键词
Histopathology image classification,Unsupervised domain adaptation,Deep learning,Microscopy image,While slide image
AI 理解论文
溯源树
样例
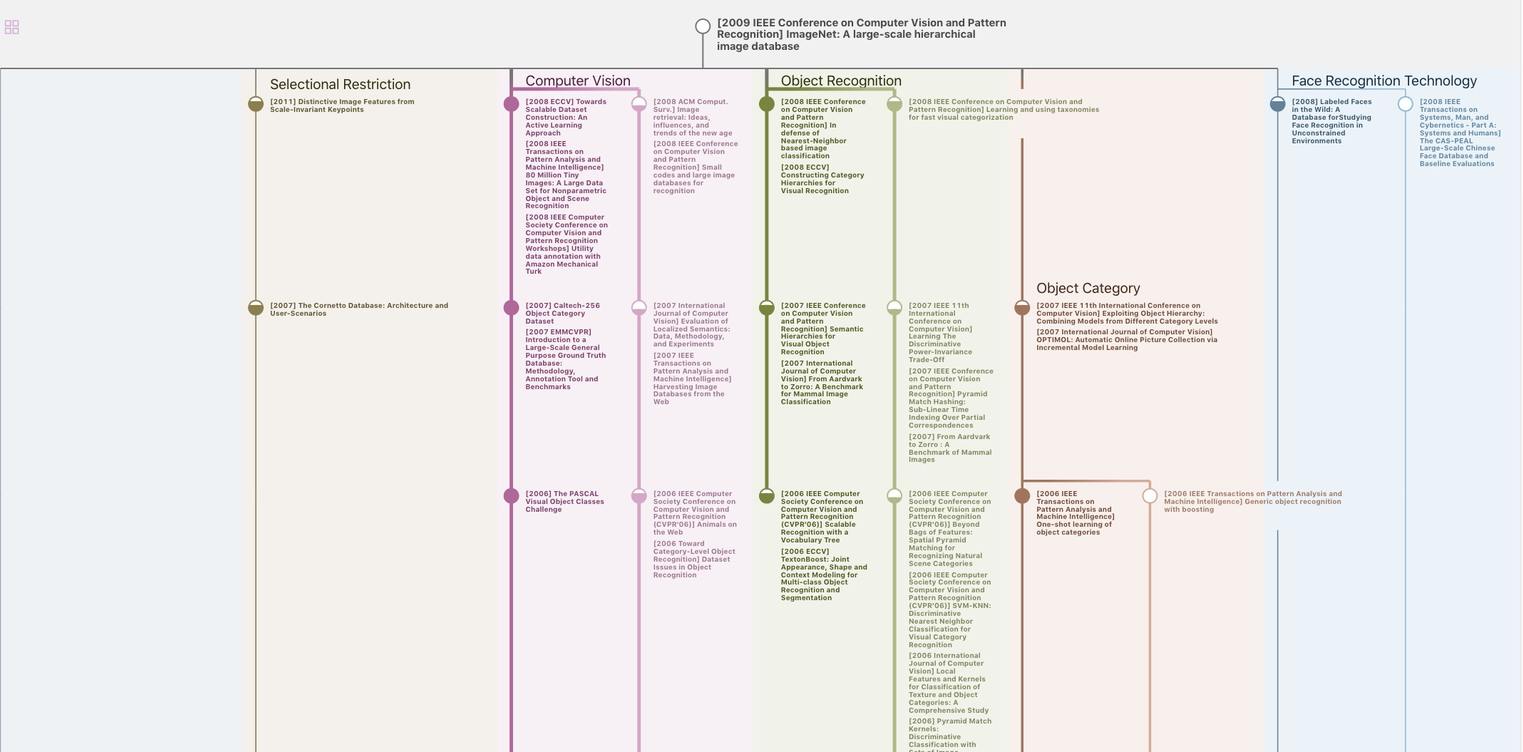
生成溯源树,研究论文发展脉络
Chat Paper
正在生成论文摘要