Task Adaptive Metric Space for Medium-Shot Medical Image Classification.
Lecture Notes in Computer Science(2019)
摘要
In the medical domain, one challenge of deep learning is to build sample-efficient models from a small number of labeled data. In recent years, meta-learning has become an important approach to few-shot image classification. However, current research on meta-learning focuses on learning from a few examples; we propose to extend few-shot learning to medium-shot to evaluate medical classification tasks in a more realistic setup. We build a baseline evaluation procedure by analyzing two representative meta-learning methods through the lens of bias-variance tradeoff, and propose to fuse the two techniques for better bias-variance equilibrium. The proposed method, Task Adaptive Metric Space (TAMS), fine-tunes parameters of a metric space to represent medical data in a more semantically meaningful way. Our empirical studies suggest that TAMS outperforms other baselines. Visualizations on the metric space show TAMS leads to better-separated clusters. Our baselines and evaluation procedure of the proposed TAMS opens the door to more research on medium-shot medical image classification.
更多查看译文
关键词
Meta-learning,Image classification,Medium-shot learning
AI 理解论文
溯源树
样例
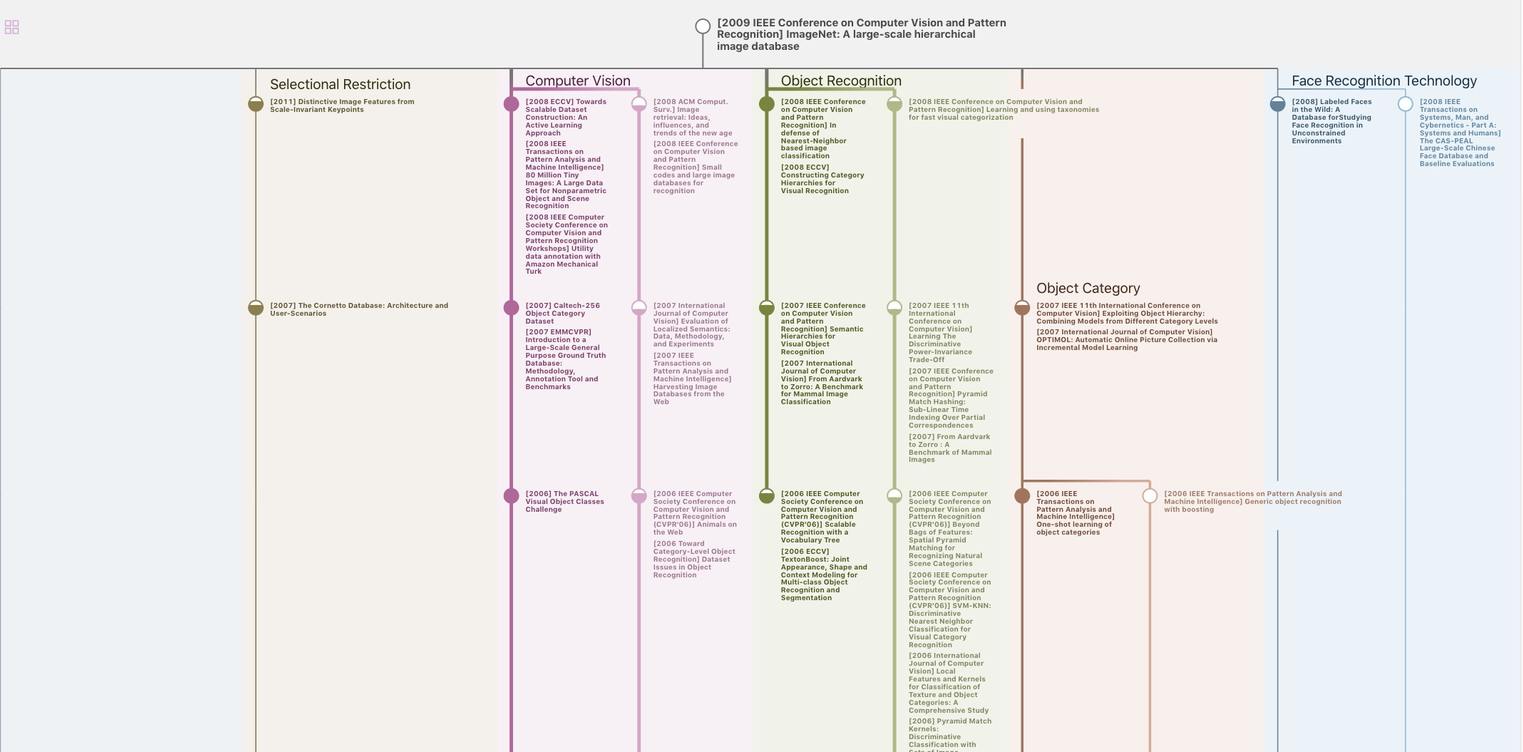
生成溯源树,研究论文发展脉络
Chat Paper
正在生成论文摘要