A Study on the use of State-of-the-Art CNNs with Fine Tuning for Spatial Stream Generation for Activity Recognition
2019 IEEE International Conference on Electrical, Computer and Communication Technologies (ICECCT)(2019)
摘要
Recurrent neural network (RNN) models have been proven successful in modeling the temporal dynamics in videos of which Long Short-Term Memory (LSTM) networks have been specifically successful as it does not suffer from the vanishing gradient problem. They along with Convolutional Neural Networks (CNN) for visual feature extraction are popularly referred as the Long-term Recurrent Convolutional Networks (LRCN) and have been widely accepted in the recent times for activities like video activity classification, video captioning and video description. The features for these models may be generated using single spatial stream or dual streams, both spatial and motion streams from the video frames. The paper is a study on how the State-of-the-Art networks like ResNet50, InceptionV3 and MobileNet perform with fine tuning for spatial feature extraction in the task of activity recognition in videos using LRCN with stacked LSTM. The fine-tuning approach and optimization settings for the extraction of the visual features from the State-of-the-Art pretrained networks is also discussed in this paper.
更多查看译文
关键词
Activity Recognition,Transfer Learning,Fine Tuning,Deep Learning,Convolutional Neural Network,LSTM,LRCN,ResNet50,InceptionV3,MobileNet
AI 理解论文
溯源树
样例
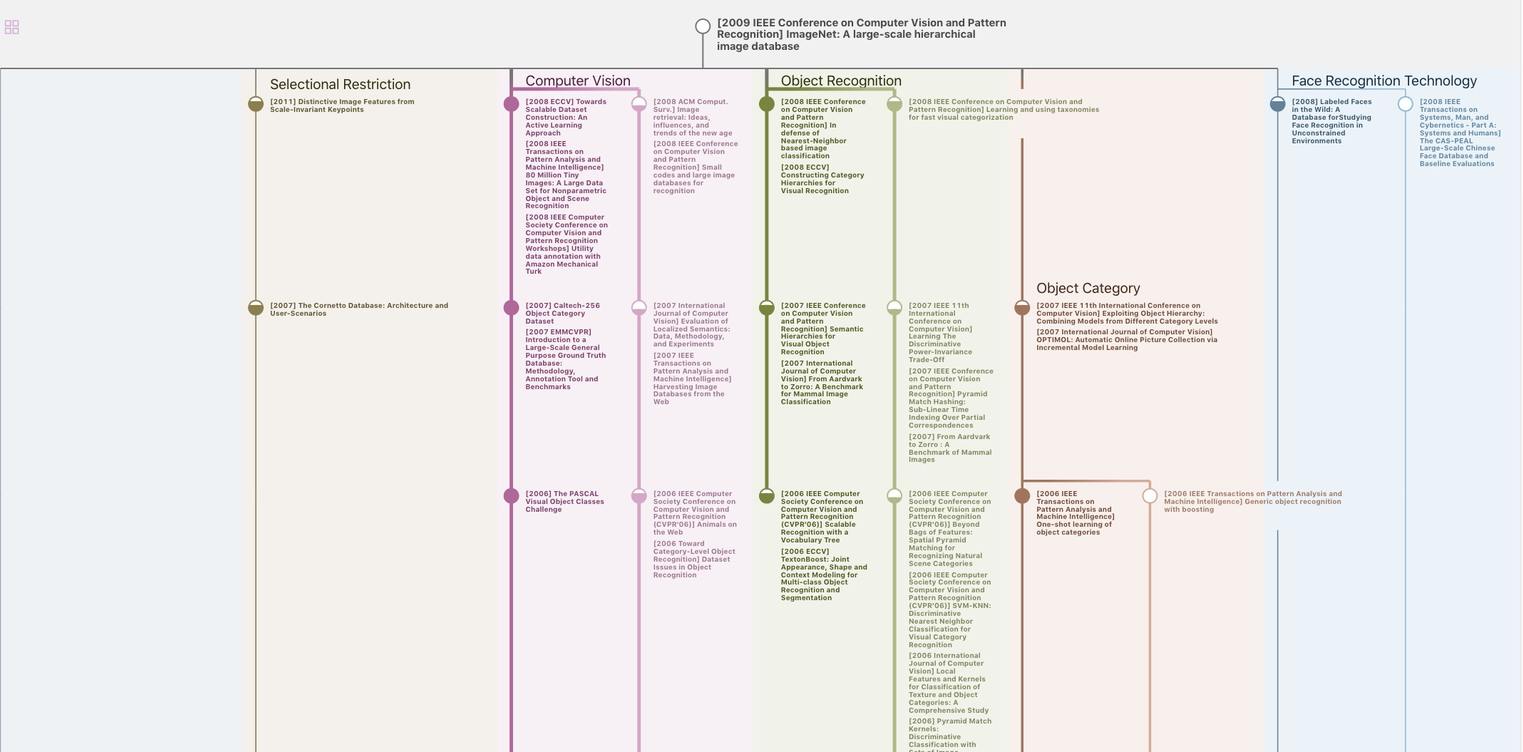
生成溯源树,研究论文发展脉络
Chat Paper
正在生成论文摘要