Prediction Of Acute Care Service Utilization For Patients With Hematologic Malignancies.
JOURNAL OF CLINICAL ONCOLOGY(2019)
摘要
312 Background: Improving the ability to predict which patients are at increased risk for acute care services subsequent to hospitalization can lead to more effective interventions and quality care. This study evaluated the performance of risk models to predict 30-day acute service utilization among adult inpatients with newly diagnosed hematologic malignancies. The study explored the impact of medical complications on potentially preventable service utilization, aligned with specifications for proposed measure OP-35 from the CMS Hospital Outpatient Quality Reporting Program. Methods: The study included 933 unique adult patients admitted to four acute care inpatient facilities within a non-profit community based healthcare system during calendar years 2012 to 2017. Risk models integrated a comprehensive set of data elements using clinical information from electronic medical records and facility oncology registries. Predictive models were constructed using a multivariable logistic regression, with calculation of standardized coefficients to rank order variables with the greatest impact on unplanned readmissions. Exploratory data mining techniques were conducted to augment classification of at-risk patients. Results: The mean age of study participants was 65 years and 55.1% were male. In order of prevalence, the types of hematologic malignancy were lymphoma (48.7%), leukemia (35.2%), myeloma (14.0%), and mixed types (2.1%). Approximately one fifth of patients received unplanned acute care services within 30 days after the index hospitalization (lymphoma = 23.1%, myeloma = 22.1%, leukemia = 18.6%). Among the risk models, the most influential predictors included initial length of stay, severity of illness, and consult for social work. Overall, 56.6% of patients who were readmitted within 30 days of hospitalization presented with one or more symptoms associated with the CMS medical complication measure, including cytopenia, infection, negative hydration, and/or pain. Conclusions: Optimizing quality care in a value-based health care environment requires proactive approaches such as risk prediction models for identifying patients who can benefit most from care transition interventions.
更多查看译文
关键词
acute care service utilization,hematologic malignancies,patients
AI 理解论文
溯源树
样例
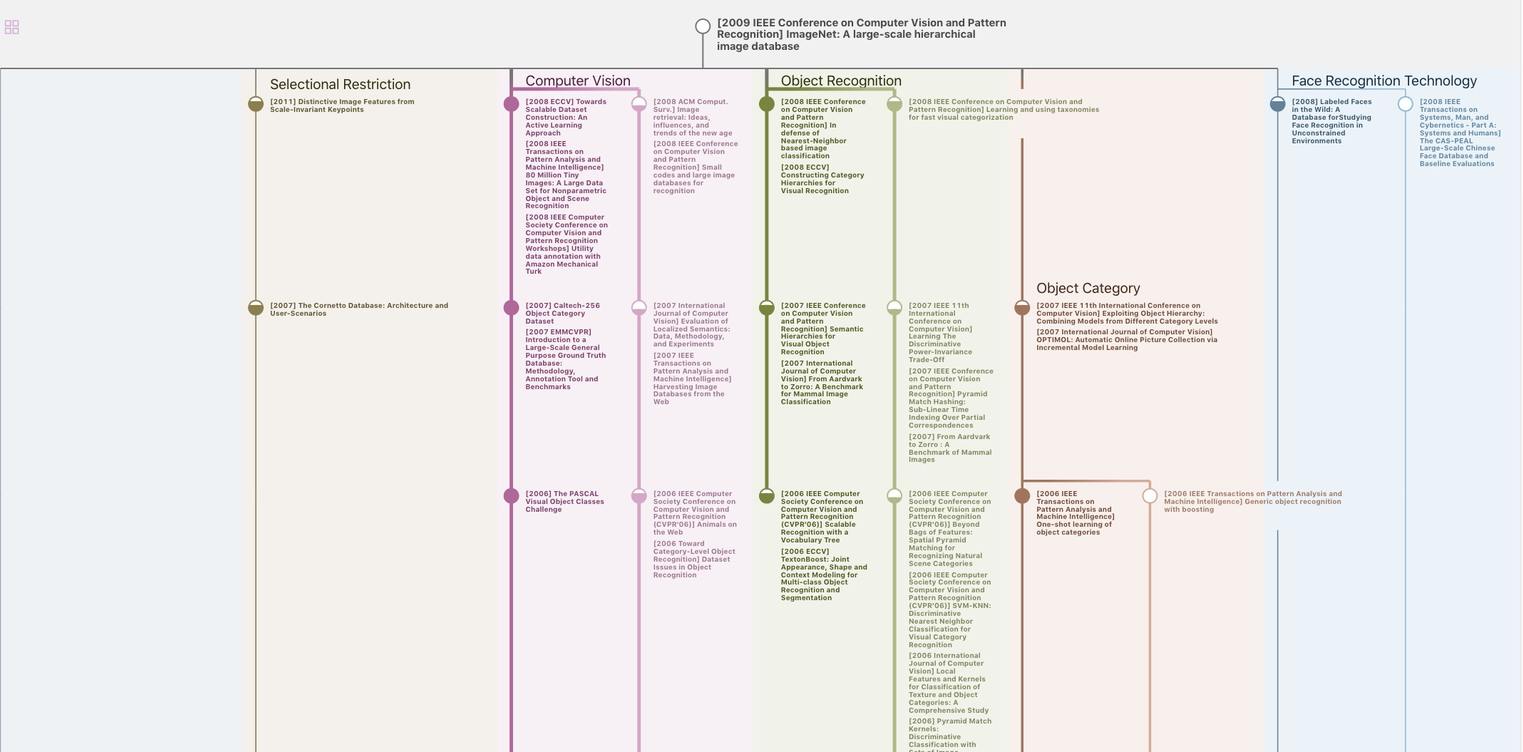
生成溯源树,研究论文发展脉络
Chat Paper
正在生成论文摘要