A Deep Learning Ensemble Approach for Diabetic Retinopathy Detection
IEEE ACCESS(2019)
摘要
Diabetic Retinopathy (DR) is an ophthalmic disease that damages retinal blood vessels. DR causes impaired vision and may even lead to blindness if it is not diagnosed in early stages. DR has five stages or classes, namely normal, mild, moderate, severe and PDR (Proliferative Diabetic Retinopathy). Normally, highly trained experts examine the colored fundus images to diagnose this fatal disease. This manual diagnosis of this condition (by clinicians) is tedious and error-prone. Therefore, various computer vision-based techniques have been proposed to automatically detect DR and its different stages from retina images. However, these methods are unable to encode the underlying complicated features and can only classify DRs different stages with very low accuracy particularly, for the early stages. In this research, we used the publicly available Kaggle dataset of retina images to train an ensemble of five deep Convolution Neural Network (CNN) models (Resnet50, Inceptionv3, Xception, Dense121, Dense169) to encode the rich features and improve the classification for different stages of DR. The experimental results show that the proposed model detects all the stages of DR unlike the current methods and performs better compared to state-of-the-art methods on the same Kaggle dataset.
更多查看译文
关键词
Diabetes,Retinopathy,Training,Predictive models,Retina,Biomedical imaging,CNN,diabetic retinopathy,deep learning,ensemble model,fundus images,medical image analysis
AI 理解论文
溯源树
样例
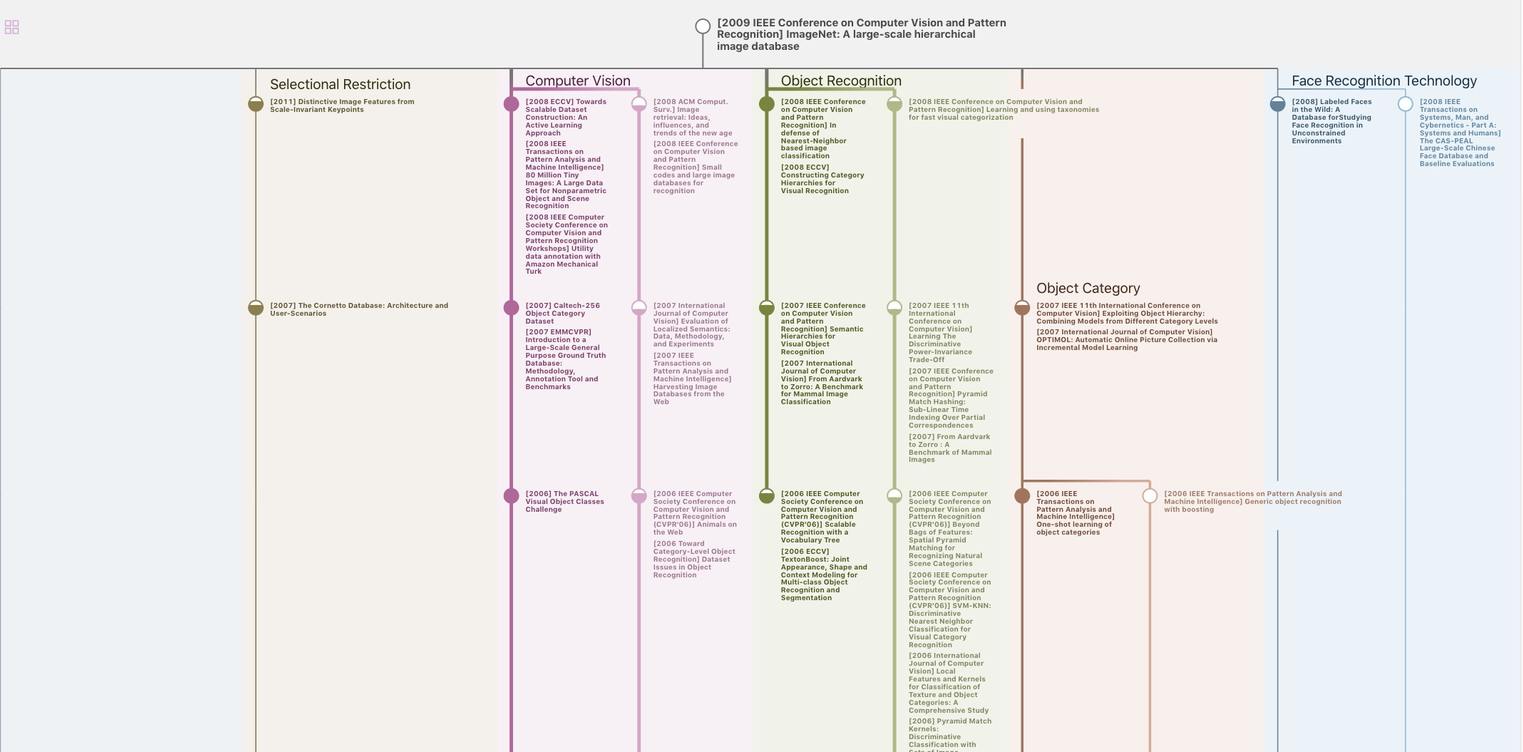
生成溯源树,研究论文发展脉络
Chat Paper
正在生成论文摘要