Rigorous Analysis of Multi-Factorial Evolutionary Algorithm as Multi-Population Evolution Model
INTERNATIONAL JOURNAL OF COMPUTATIONAL INTELLIGENCE SYSTEMS(2019)
摘要
Multi-task optimization algorithm is an emergent paradigm which solves multiple self-contained tasks simultaneously. It is thought that multi-factorial evolutionary algorithm (MFEA) can be seen as a novel multi-population algorithm, wherein each population is represented independently and evolved for the selected task only. However, the theoretical and experimental evidence to this conclusion is not very convincing and especially, the coincidence relation between MFEA and multi-population evolution model is ambiguous and inaccurate. This paper aims to make an in-depth analysis of this relationship, and to provide more theoretical and experimental evidence to support the idea. In this paper, we clarify several key issues unsettled to date, and design a novel across-population crossover approach to avoid population drift. Then MFEA and its variation are reviewed carefully in view of multi-population evolution model, and the coincidence relation between them are concluded. MFEA is completely recoded along with this idea and tested on 25 multi-task optimization problems. Experimental results illustrate its rationality and superiority. Furthermore, we analyze the contribution of each population to algorithm performance, which can help us design more efficient multi-population algorithm for multi-task optimization.
更多查看译文
关键词
Multi-factorial evolutionary algorithm,Multi-population evolution model,Multi-task optimization,Knowledge transfer,Across-population
AI 理解论文
溯源树
样例
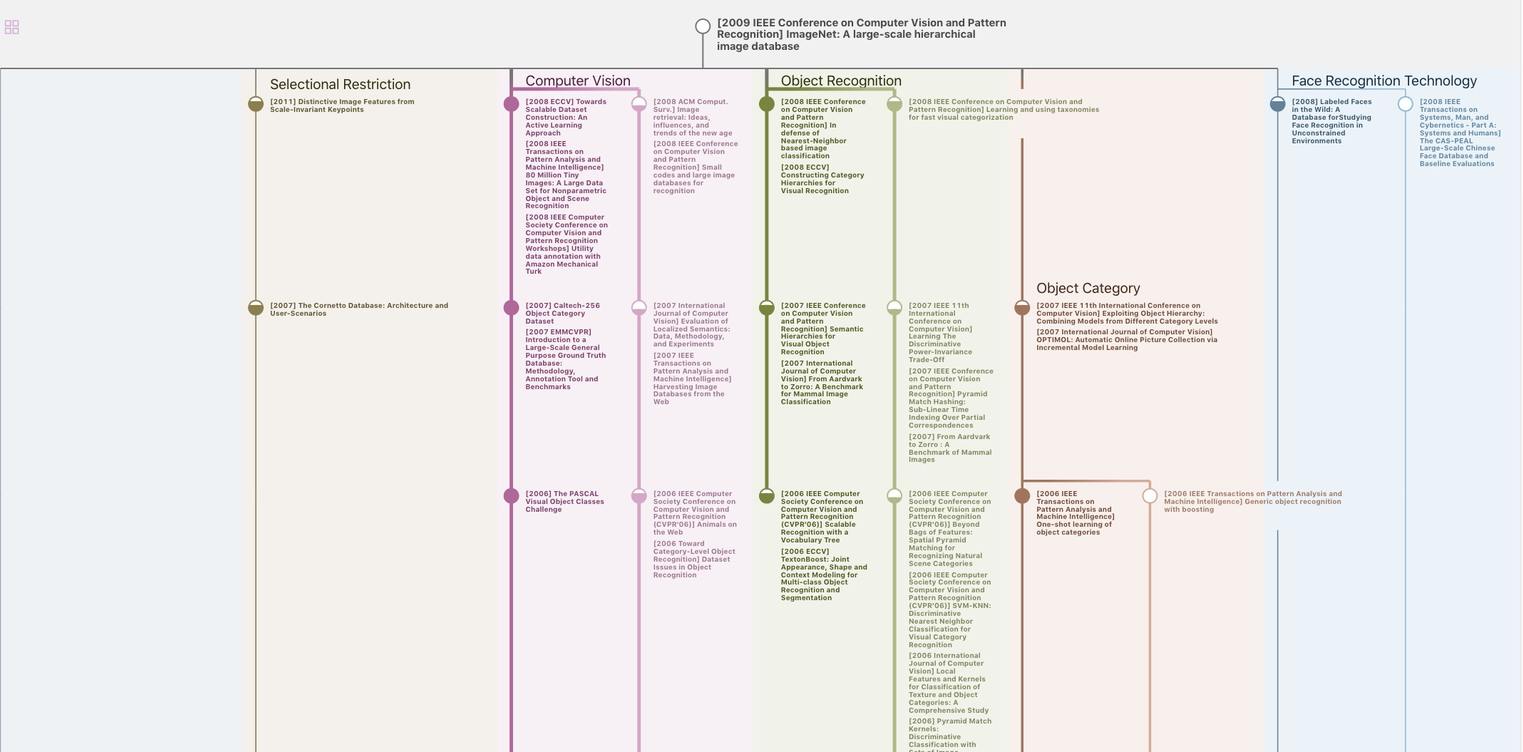
生成溯源树,研究论文发展脉络
Chat Paper
正在生成论文摘要