Cnn Mode For Screen Content Image Quality Assessment Based On Region Difference
2019 IEEE 4TH INTERNATIONAL CONFERENCE ON SIGNAL AND IMAGE PROCESSING (ICSIP 2019)(2019)
摘要
Deep learning methods for image quality assessment (IQA) is becoming more and more popular, and a variety of convolutional neural network(CNN) models for natural image quality assessment (NI-QA) have been proposed. Screen content image quality assessment (SCI-QA) is a relatively new topic compared to natural image quality assessments. Screen content. image is different from natural images. It usually consists of pictorial regions and textual regions, which results in different visual perceptions of different regions in a SCI. In the CNN models for IQA, they usually take image patches as input of the network. Therefore, we propose a method to segment the distorted image into image patches and then distinguish them into pictorial patches and textual patches. Due to the different characteristics of those two regions, the quality scores of pictorial and textual regions are separately predicted by the CNN model, and then are fused to indicate the whole quality degree of distorted SCIs. The scores are fused according to different weights that are calculated by the local activity degree of SCIs. The experimental results in the SIQAI) database validate the effectiveness of the proposed CNN-based method compared to several advanced IQA methods.
更多查看译文
关键词
image quality assessment, screen content image, image patch, convolutional neural network
AI 理解论文
溯源树
样例
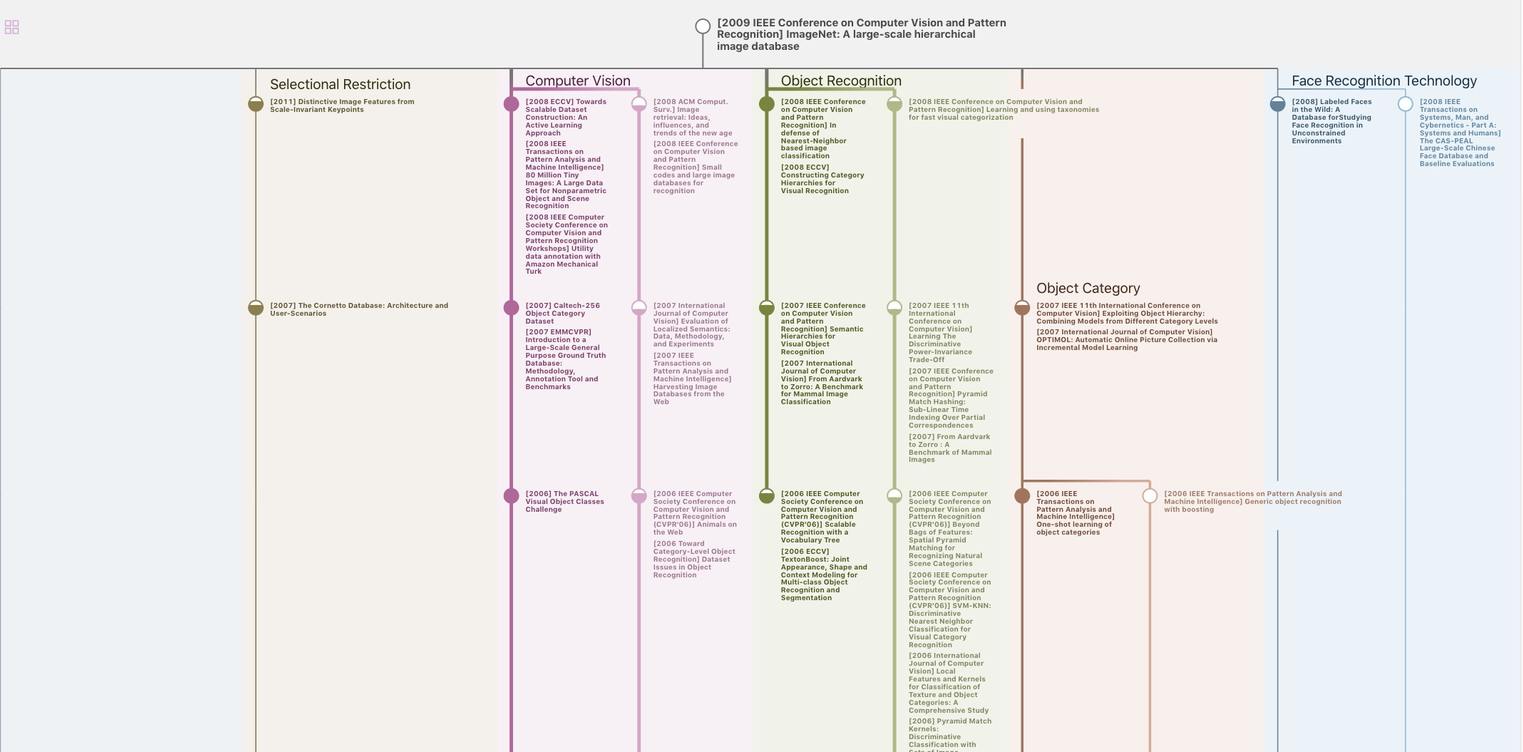
生成溯源树,研究论文发展脉络
Chat Paper
正在生成论文摘要