A learning-based multiscale modelling approach to real-time serial manipulator kinematics simulation
Neurocomputing(2020)
摘要
Kinematics simulation is central to the control of serial manipulators in human machine interaction, which highly depends upon computational efficiency and accuracy for the sake of enabling real-time analysis and interaction. In this paper, a novel learning-based multiscale modelling approach is proposed to effectively address the efficiency and accuracy trade-offs through a combination of models with different levels of fidelity. Specifically, low-fidelity models are formulated using Kernel Ridge Regression (KRR) to achieve much lower computational cost as its kinematic approximation. Additionally, high-fidelity models are created based on the Long Short Term Memory (LSTM) neural network, which can calibrate fidelity by training the significant samples and eliminate position singularity of a serial manupulator. The proposed approach is evaluated both by using numerical tests and by applying it to a collaborative industrial robot arm. The experimental results obtained demonstrate that it can achieve better performance in terms of both accuracy and efficiency compared with the other popular methods.
更多查看译文
关键词
Human Machine Interaction,Serial Manipulator Kinematics,Multiscale Modelling,Kernel Ridge Regression,LSTM Neural Network
AI 理解论文
溯源树
样例
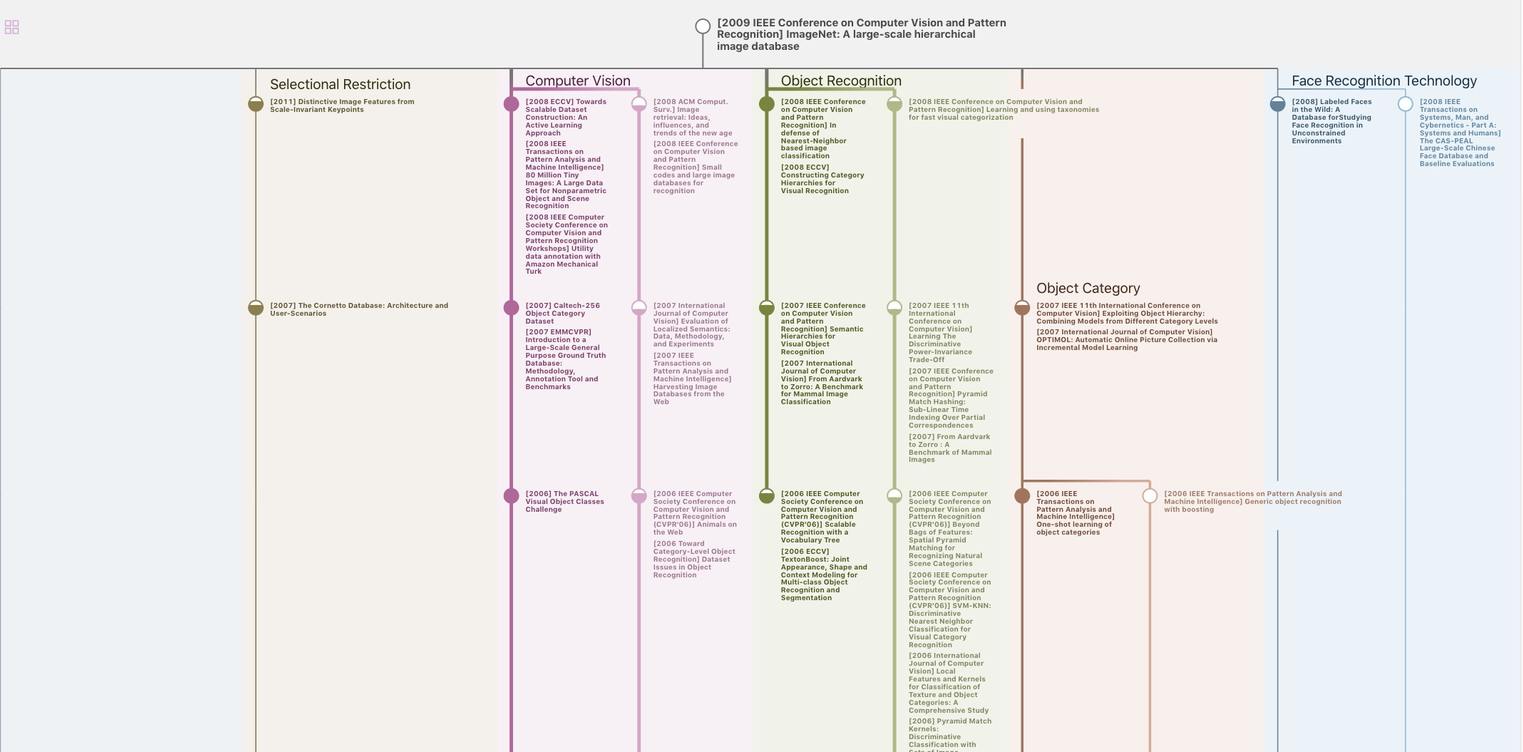
生成溯源树,研究论文发展脉络
Chat Paper
正在生成论文摘要