Effects of prediction accuracy of the proportion of vegetation cover on land surface emissivity and temperature using the NDVI threshold method
International Journal of Applied Earth Observations and Geoinformation(2020)
摘要
Predicting land surface energy budgets requires precise information of land surface emissivity (LSE) and land surface temperature (LST). LST is one of the essential climate variables as well as an important parameter in the physics of land surface processes at local and global scales, while LSE is an indicator of the material composition. Despite the fact that there are numerous publications on methods and algorithms for computing LST and LSE using remotely sensed data, accurate prediction of these variables is still a challenging task. Among the existing approaches for calculating LSE and LST, particular attention has been paid to the normalised difference vegetation index threshold method (NDVITHM), especially for agriculture and forest ecosystems. To apply NDVITHM, knowledge of the proportion of vegetation cover (PV) is essential. The objective of this study is to investigate the effect of the prediction accuracy of the PV on the estimation of LSE and LST when using NDVITHM. In August 2015, a field campaign was carried out in mixed temperate forest of the Bavarian Forest National Park, in southeastern Germany, coinciding with a Landsat-8 overpass. The PV was measured in the field for 37 plots. Four different vegetation indices, as well as artificial neural network approaches, were used to estimate PV and to compute LSE and LST. The results showed that the prediction accuracy of PV improved using an artificial neural network (R2CV = 0.64, RMSECV = 0.05) over classic vegetation indices (R2CV = 0.42, RMSECV = 0.06). The results of this study also revealed that variation in the accuracy of the estimated PV affected calculation results of the LSE. In addition, our findings revealed that, though LST depends on LSE, other parameters should also be taken into account when predicting LST, as more accurate LSE results did not increase the prediction accuracy of LST.
更多查看译文
关键词
Proportion of vegetation cover,Thermal infrared remote sensing,Land surface emissivity,Land surface temperature,Vegetation index,Landsat-8
AI 理解论文
溯源树
样例
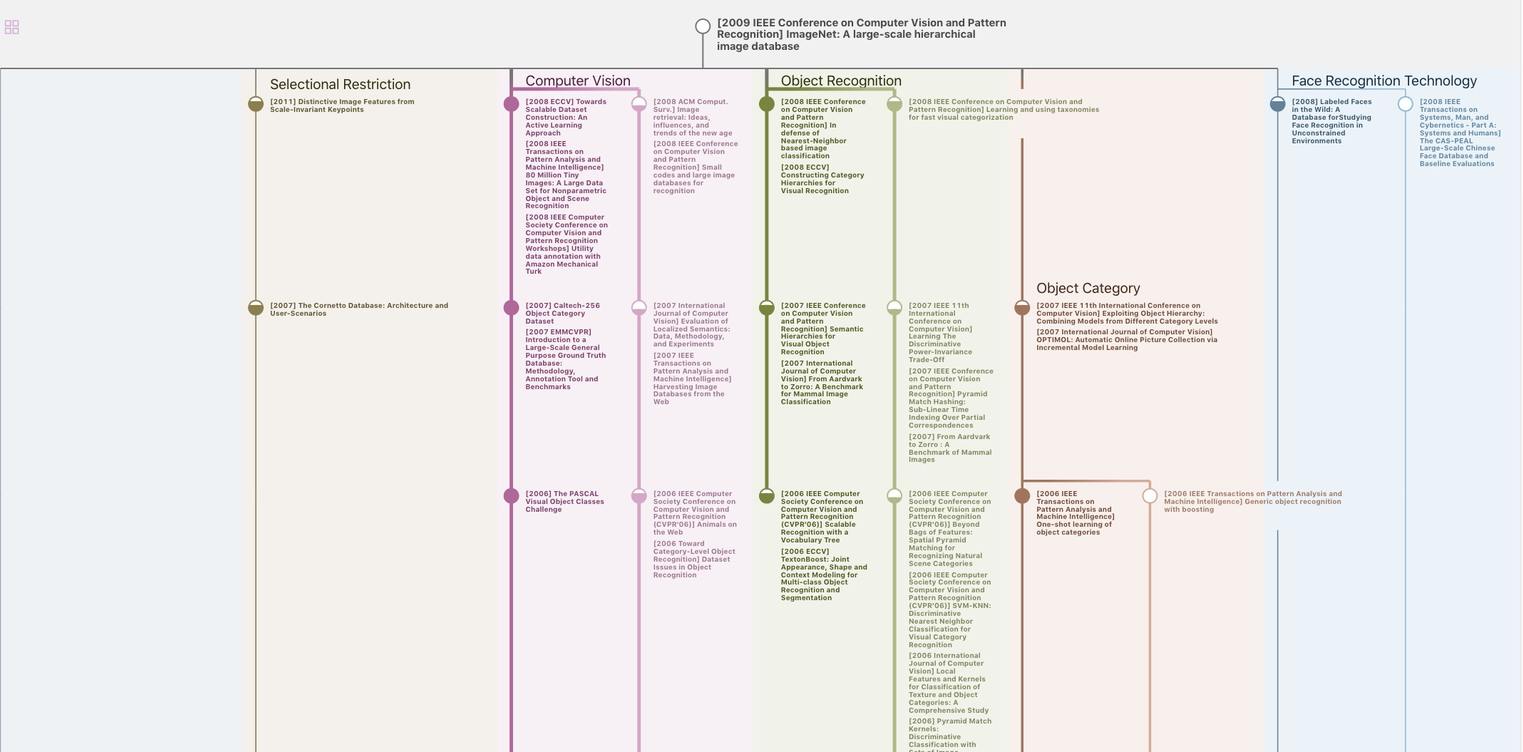
生成溯源树,研究论文发展脉络
Chat Paper
正在生成论文摘要