Face identification with top-push constrained generalized low-rank approximation of matrices
IEEE Access(2019)
摘要
We present a novel top-push constrained feature learning (TFL) method for face identification. The idea is to learn low-rank approximations from raw intensity face images such that the squared distance between all faces of the same identity should be smaller than that of different identities by a margin. To this end, we formulate the learning process under the framework of generalized low-rank approximation of matrices (GLRAM) supervised with a top-push constraint. GLRAM operates on the matrix representation of images to seek low-dimensional and compact features, which improves the discriminative power of the features while reducing the time and space costs. The top-push constraint aims to optimize the face identification accuracy of the top-rank matching list, which makes the learned features more discriminative and compact, thus reducing the variability and ambiguity. Once the optimization is finished, the features are vectorized and used for similarity measurement. The effectiveness of TFL is verified on four publicly available face datasets.
更多查看译文
关键词
Face,Measurement,Information science,Training data,Principal component analysis,Optimization,Feature extraction,Feature learning,generalized low-rank approximation,face identification,top-push
AI 理解论文
溯源树
样例
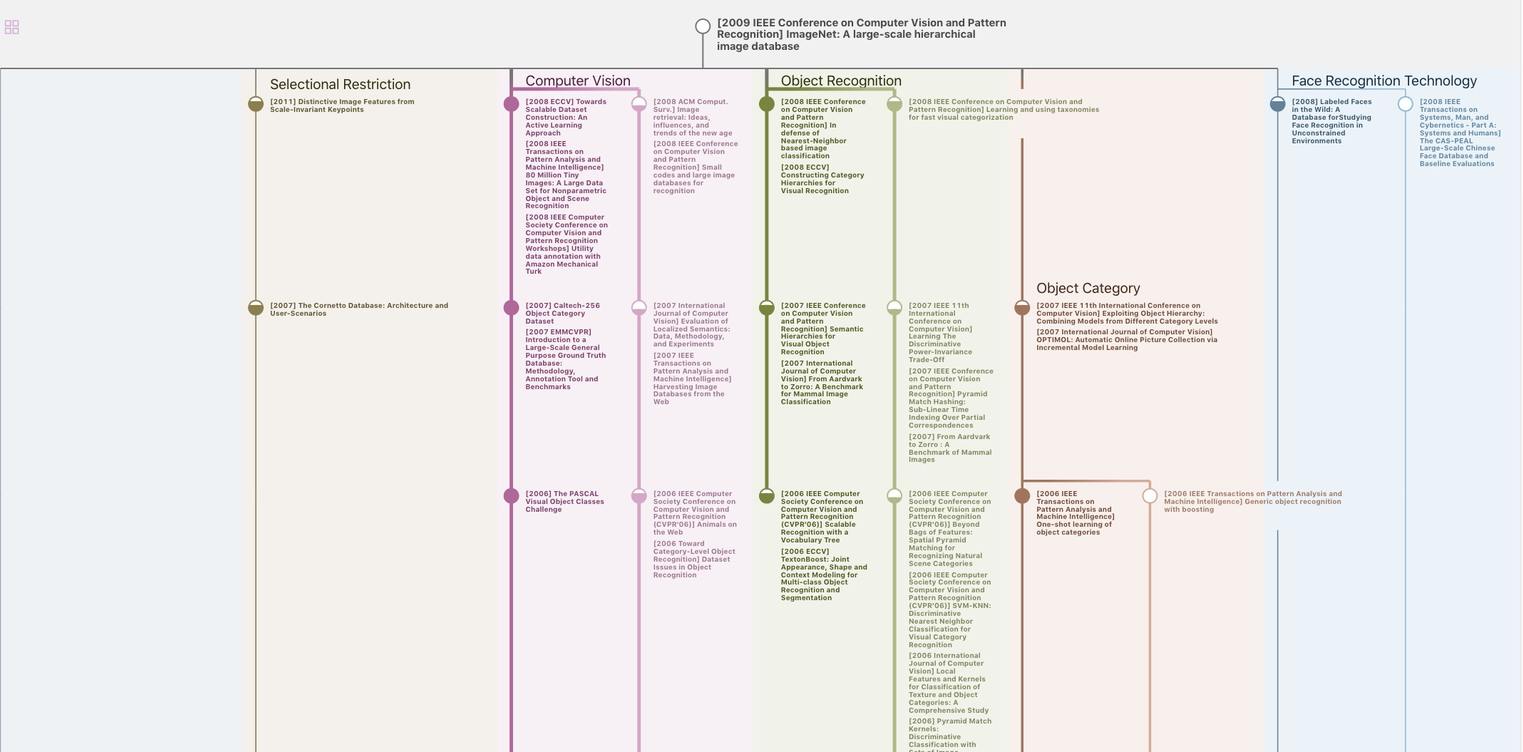
生成溯源树,研究论文发展脉络
Chat Paper
正在生成论文摘要