Transformer Fault Diagnosis Based On Deep Brief Sparse Autoencoder
PROCEEDINGS OF THE 38TH CHINESE CONTROL CONFERENCE (CCC)(2019)
摘要
Dissolved gas analysis (DGA) is an effective way to diagnose the internal faults of transformer. This paper proposes a deep belief sparse autoencoder (DBSAE), which can be performed on DGA data to detect the transformer fault. The proposed method is constructed by two layers of sparse autoencoder and one layer of back propagation network. DBSAE adopts the unsupervised training to collect the features of DGA raw data, and the back propagation network with the supervised training could realize the transformer faults diagnosis. The experiments are performed on a real DGA dataset. The experimental results verify the effectiveness of the proposed method, which can extract features from the original data, and achieve a superior correct differentiation rate which reached 95.4% in average accuracy on transformer fault diagnosis.
更多查看译文
关键词
Transformer fault diagnosis, dissolved gas analysis, sparse autoencoder, deep belief network
AI 理解论文
溯源树
样例
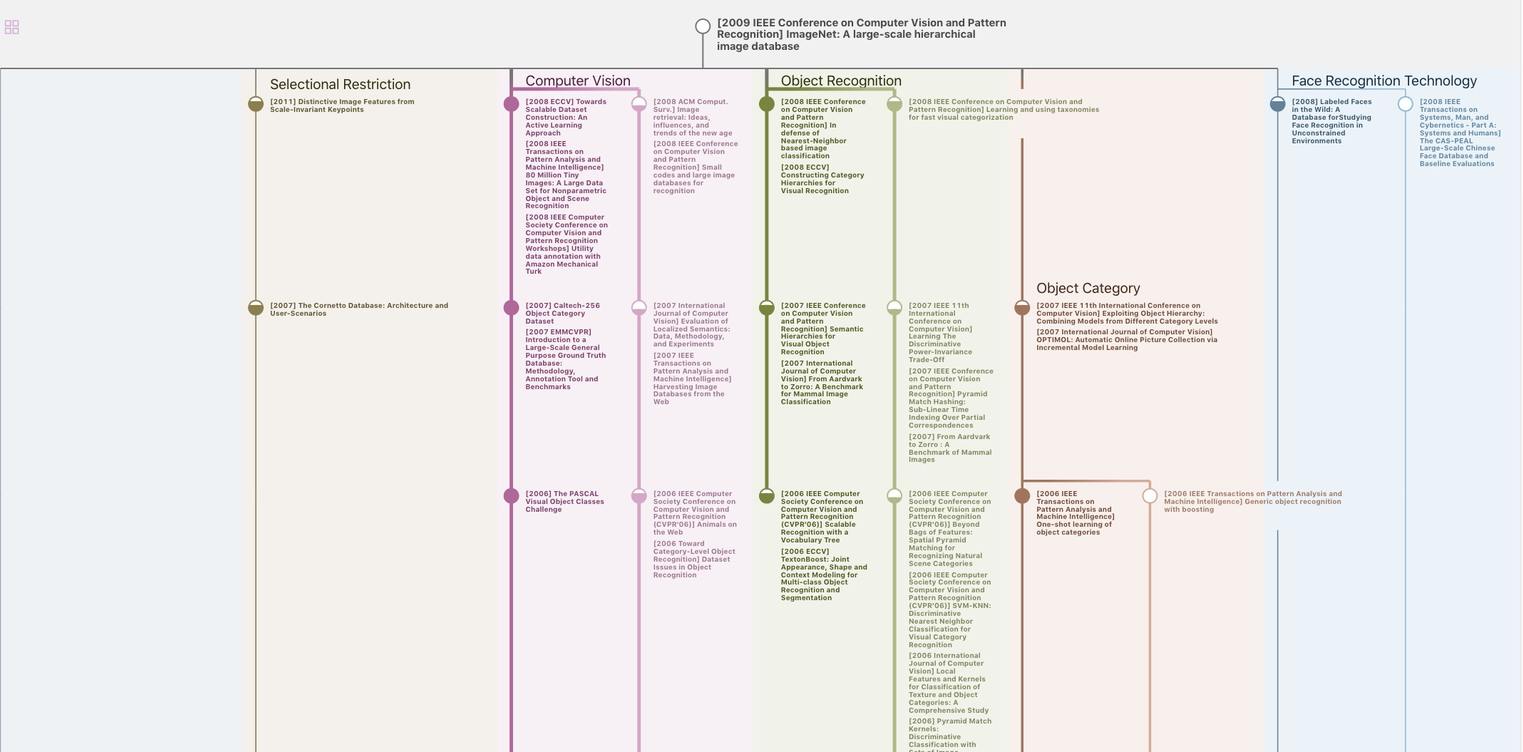
生成溯源树,研究论文发展脉络
Chat Paper
正在生成论文摘要