Spatio-Temporal Fusion Based Convolutional Sequence Learning for Lip Reading
2019 IEEE/CVF International Conference on Computer Vision (ICCV)(2019)
摘要
Current state-of-the-art approaches for lip reading are based on sequence-to-sequence architectures that are designed for natural machine translation and audio speech recognition. Hence, these methods do not fully exploit the characteristics of the lip dynamics, causing two main drawbacks. First, the short-range temporal dependencies, which are critical to the mapping from lip images to visemes, receives no extra attention. Second, local spatial information is discarded in the existing sequence models due to the use of global average pooling (GAP). To well solve these drawbacks, we propose a Temporal Focal block to sufficiently describe short-range dependencies and a Spatio-Temporal Fusion Module (STFM) to maintain the local spatial information and to reduce the feature dimensions as well. From the experiment results, it is demonstrated that our method achieves comparable performance with the state-of-the-art approach using much less training data and much lighter Convolutional Feature Extractor. The training time is reduced by 12 days due to the convolutional structure and the local self-attention mechanism.
更多查看译文
关键词
convolutional sequence learning,temporal focal block,local self-attention mechanism,convolutional structure,convolutional feature extractor,Spatio-Temporal Fusion Module,short-range dependencies,global average pooling,sequence models,local spatial information,lip images,short-range temporal dependencies,lip dynamics,audio speech recognition,natural machine translation,sequence-to-sequence architectures,lip reading,time 12.0 d
AI 理解论文
溯源树
样例
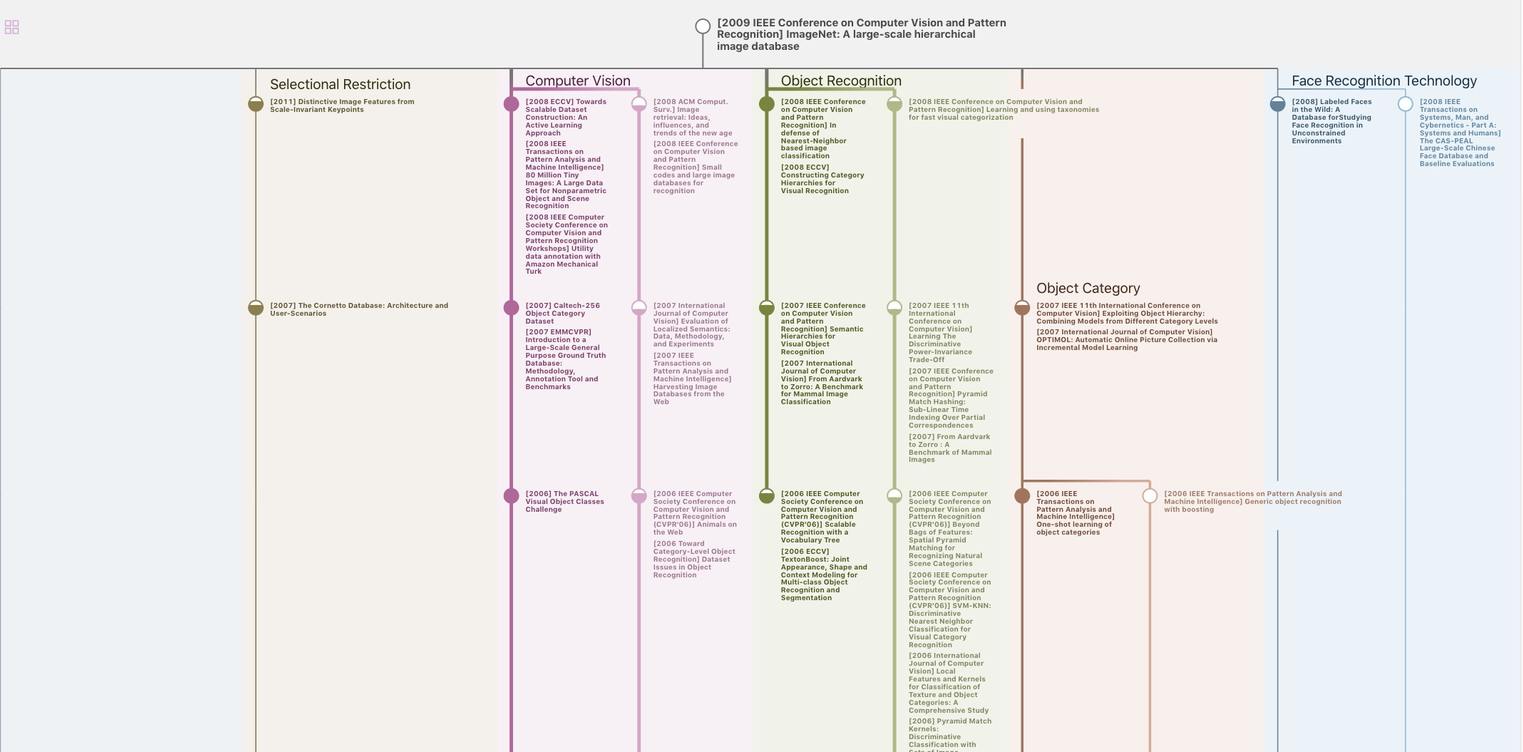
生成溯源树,研究论文发展脉络
Chat Paper
正在生成论文摘要