A novel recommendation method based on general matrix factorization and artificial neural networks
NEURAL COMPUTING & APPLICATIONS(2019)
摘要
Collaborative filtering is a successful approach in relevant item or service recommendation provision to users in rich, online domains. This approach has been widely applied in commercial environments with success, especially in online marketing, similar product suggestion and selection and tailor-made consumer suggestions. However, regardless of its market penetration, there are still considerable limitations in terms of accuracy in the proposed recommendations stemming from the high-frequency low-relevance user-item bias, data specificities and individual user patterns and needs that may be hidden in data. We propose a novel recommendation approach that improves accuracy and requires significantly less maintenance compared to traditional collaborative filtering. For the experimental evaluation, we use two real data sets and well-known metrics with the results validating our method. Our proposed method outperforms all the alternative recommendation methods for each of the two data sets and metrics and seems holistically effective against alternatives since it requires fewer settings to be considered without affecting the output.
更多查看译文
关键词
Recommender systems,Collaborative filtering,Matrix factorization,Neural networks
AI 理解论文
溯源树
样例
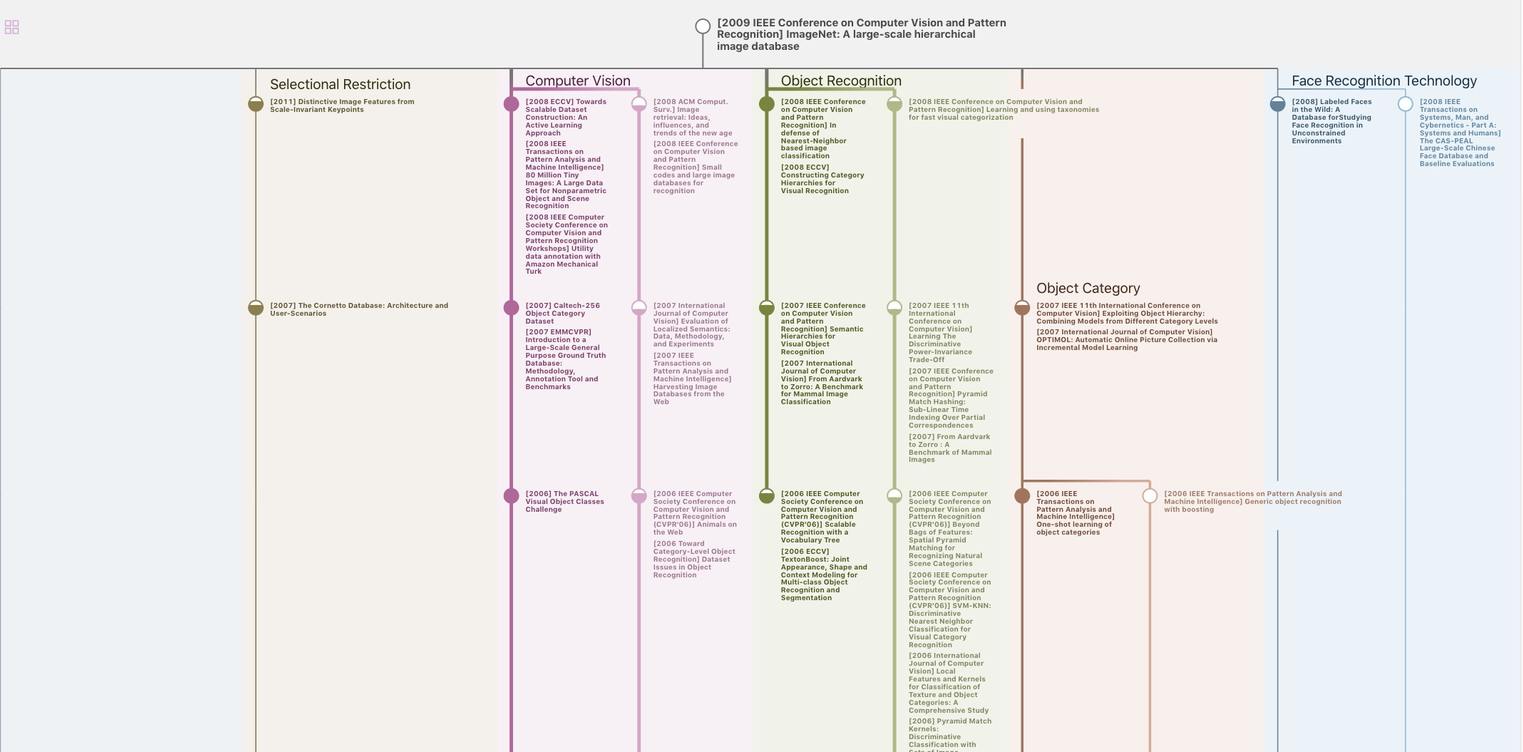
生成溯源树,研究论文发展脉络
Chat Paper
正在生成论文摘要