Road Extraction of High-Resolution Remote Sensing Images Derived from DenseUNet
REMOTE SENSING(2019)
摘要
Road network extraction is one of the significant assignments for disaster emergency response, intelligent transportation systems, and real-time updating road network. Road extraction base on high-resolution remote sensing images has become a hot topic. Presently, most of the researches are based on traditional machine learning algorithms, which are complex and computational because of impervious surfaces such as roads and buildings that are discernible in the images. Given the above problems, we propose a new method to extract the road network from remote sensing images using a DenseUNet model with few parameters and robust characteristics. DenseUNet consists of dense connection units and skips connections, which strengthens the fusion of different scales by connections at various network layers. The performance of the advanced method is validated on two datasets of high-resolution images by comparison with three classical semantic segmentation methods. The experimental results show that the method can be used for road extraction in complex scenes.
更多查看译文
关键词
high-resolution remote sensing imagery,multi-scale,road extraction,machine learning,DenseUNet
AI 理解论文
溯源树
样例
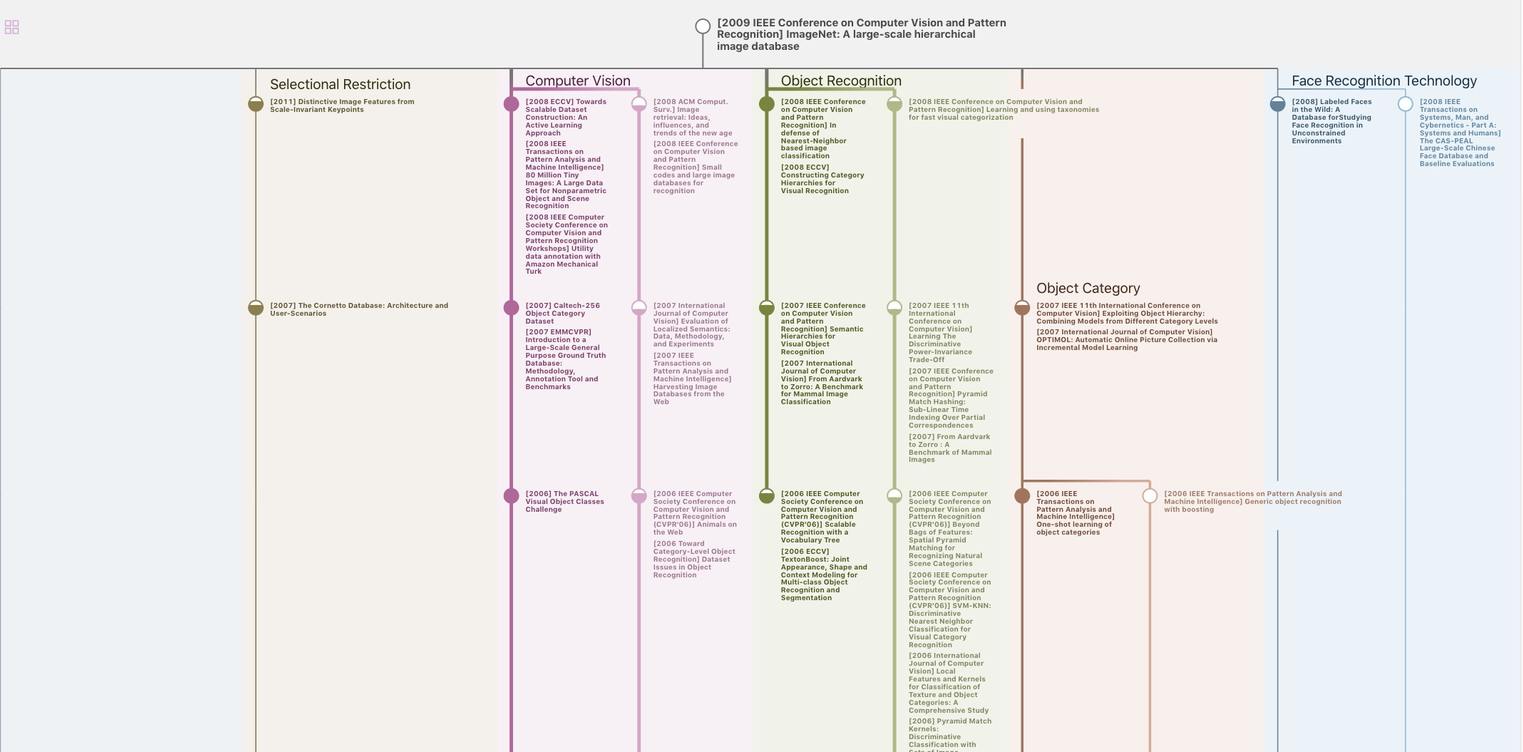
生成溯源树,研究论文发展脉络
Chat Paper
正在生成论文摘要