Deep Learning For Organic Clutter Detection In Radar Cross Section Data
international conference on electromagnetics in advanced applications(2019)
摘要
To date, radar cross section (RCS) measurements at outdoor ranges require manual inspection by test engineers to check for contamination from natural causes. This is an expensive, time-consuming operation that acts as a bottleneck for collecting RCS measurements at these ranges. Advancements in deep learning (DL) have allowed automation of similar types of manual labor. Unfortunately, such techniques normally require enormous training datasets which are impractical to collect at outdoor RCS ranges. We present a novel deep learning method for detecting organic contamination in RCS data that is robust to differing target geometries and performs well even with as little as one contamination measurement for training. Our test case also demonstrates that this method is even capable of outperforming manual human inspection, by uncovering difficult to find mistakes in the original ground truthing.
更多查看译文
关键词
Radar Cross Section, Deep Learning Error Detection, Data-starved Deep Learning
AI 理解论文
溯源树
样例
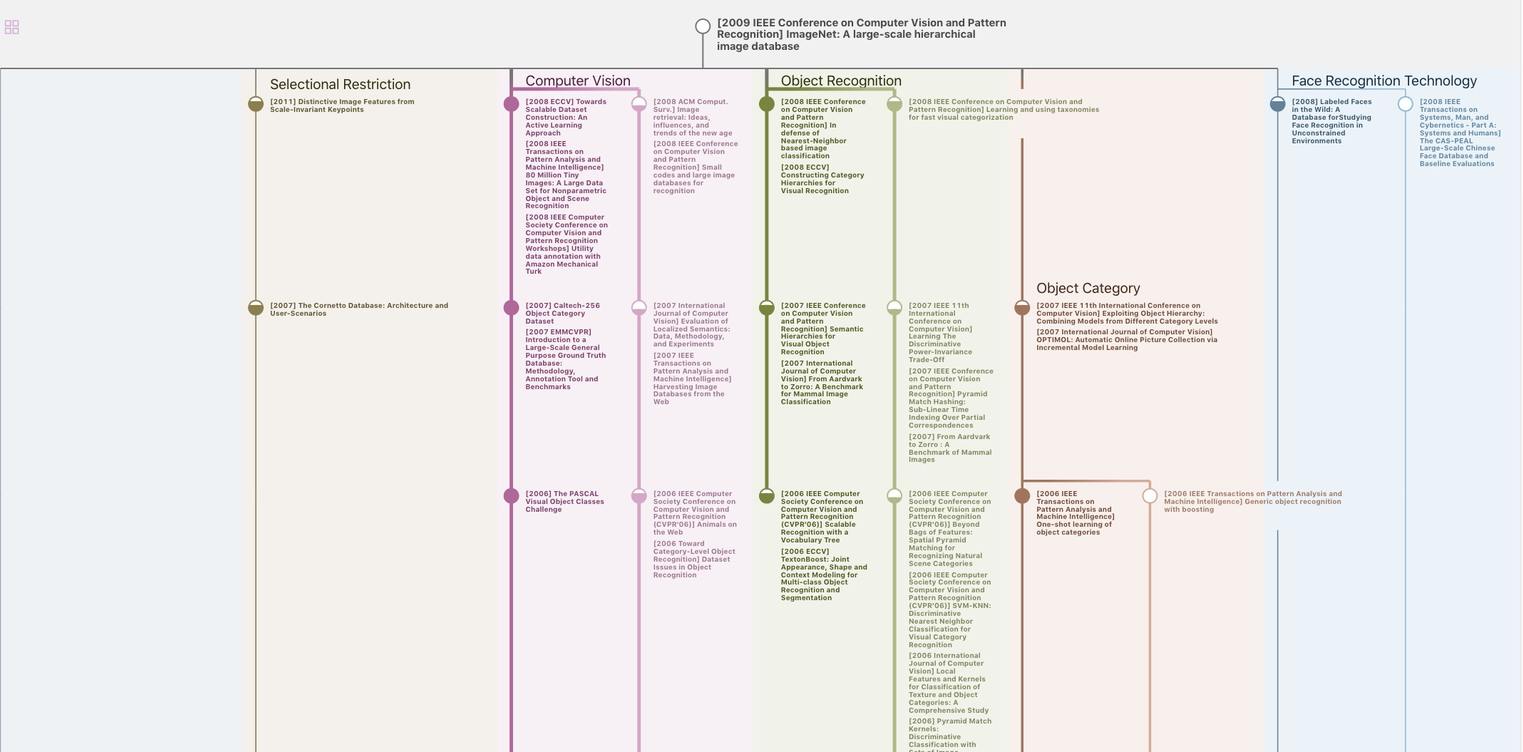
生成溯源树,研究论文发展脉络
Chat Paper
正在生成论文摘要