Long-Term Impacts Of Fair Machine Learning
ERGONOMICS IN DESIGN(2020)
摘要
Machine learning models developed from real-world data can inherit potential, preexisting bias in the dataset. When these models are used to inform decisions involving human beings, fairness concerns inevitably arise. Imposing certain fairness constraints in the training of models can be effective only if appropriate criteria are applied. However, a fairness criterion can be defined/assessed only when the interaction between the decisions and the underlying population is well understood. We introduce two feedback models describing how people react when receiving machine-aided decisions and illustrate that some commonly used fairness criteria can end with undesirable consequences while reinforcing discrimination.
更多查看译文
关键词
fairness,machine learning,sequential decision making,group representation
AI 理解论文
溯源树
样例
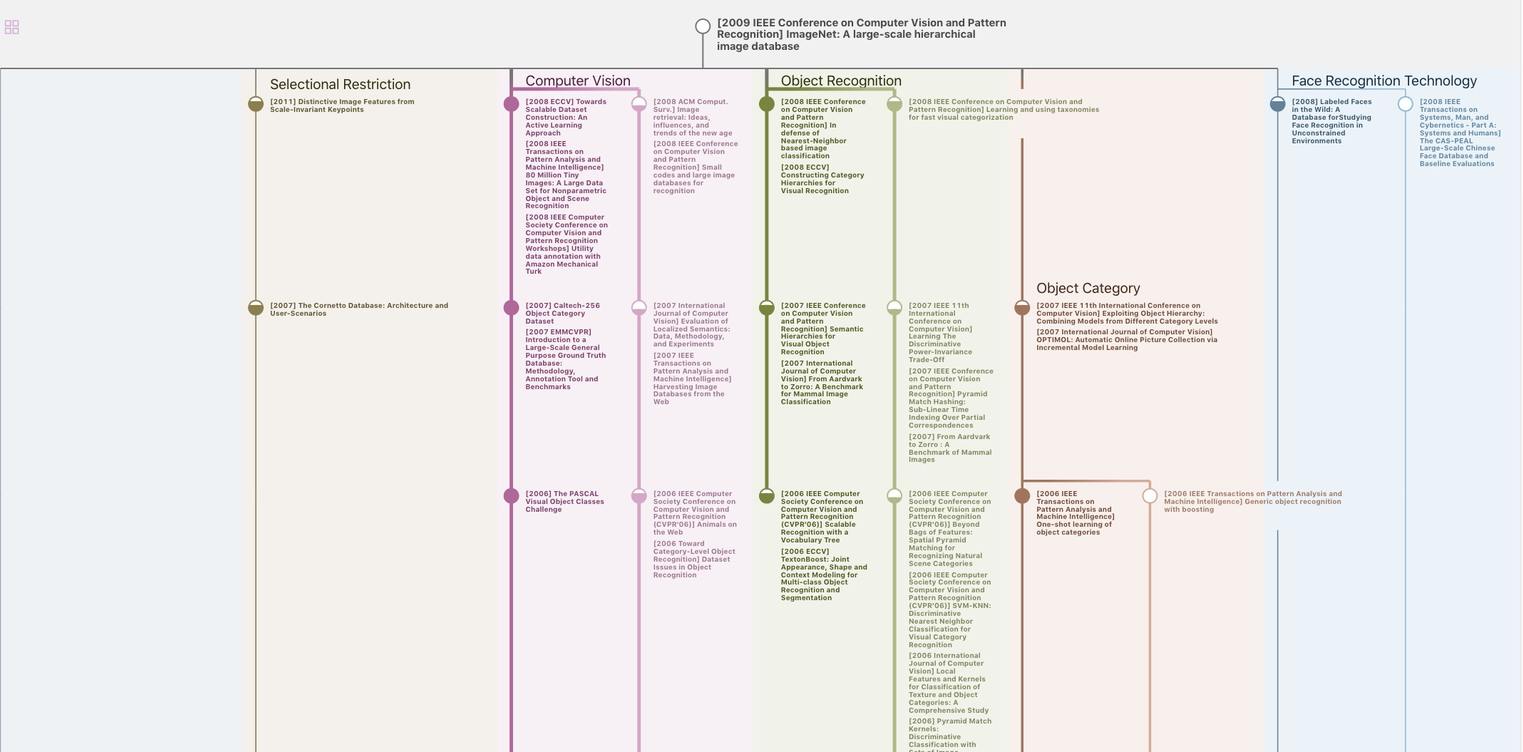
生成溯源树,研究论文发展脉络
Chat Paper
正在生成论文摘要