Realistic Peer-To-Peer Energy Trading Model For Microgrids Using Deep Reinforcement Learning
PROCEEDINGS OF 2019 IEEE PES INNOVATIVE SMART GRID TECHNOLOGIES EUROPE (ISGT-EUROPE)(2019)
摘要
In this paper, we integrate deep reinforcement learning with our realistic peer-to-peer (P2P) energy trading model to address a decision-making problem for microgrids (MGs) in the local energy market. First, an hour-ahead P2P energy trading model with a set of critical physical constraints is formed. Then, the decision-making process of energy trading is built as a Markov decision process, which is used to find the optimal strategies for MGs using a deep reinforcement learning (DRL) algorithm. Specifically, a modified deep Q-network (DQN) algorithm helps the MGs to utilise their resources and make better strategies. Finally, we choose several real-world electricity data sets to perform the simulations. The DQN-based energy trading strategies improve the utilities of the MGs and significantly reduce the power plant schedule with a virtual penalty function. Moreover, the model can determine the best battery for the selected MG. The results show that this P2P energy trading model can be applied to real-world situations.
更多查看译文
关键词
deep Q-network, deep reinforcement learning, P2P energy trading, smart grids
AI 理解论文
溯源树
样例
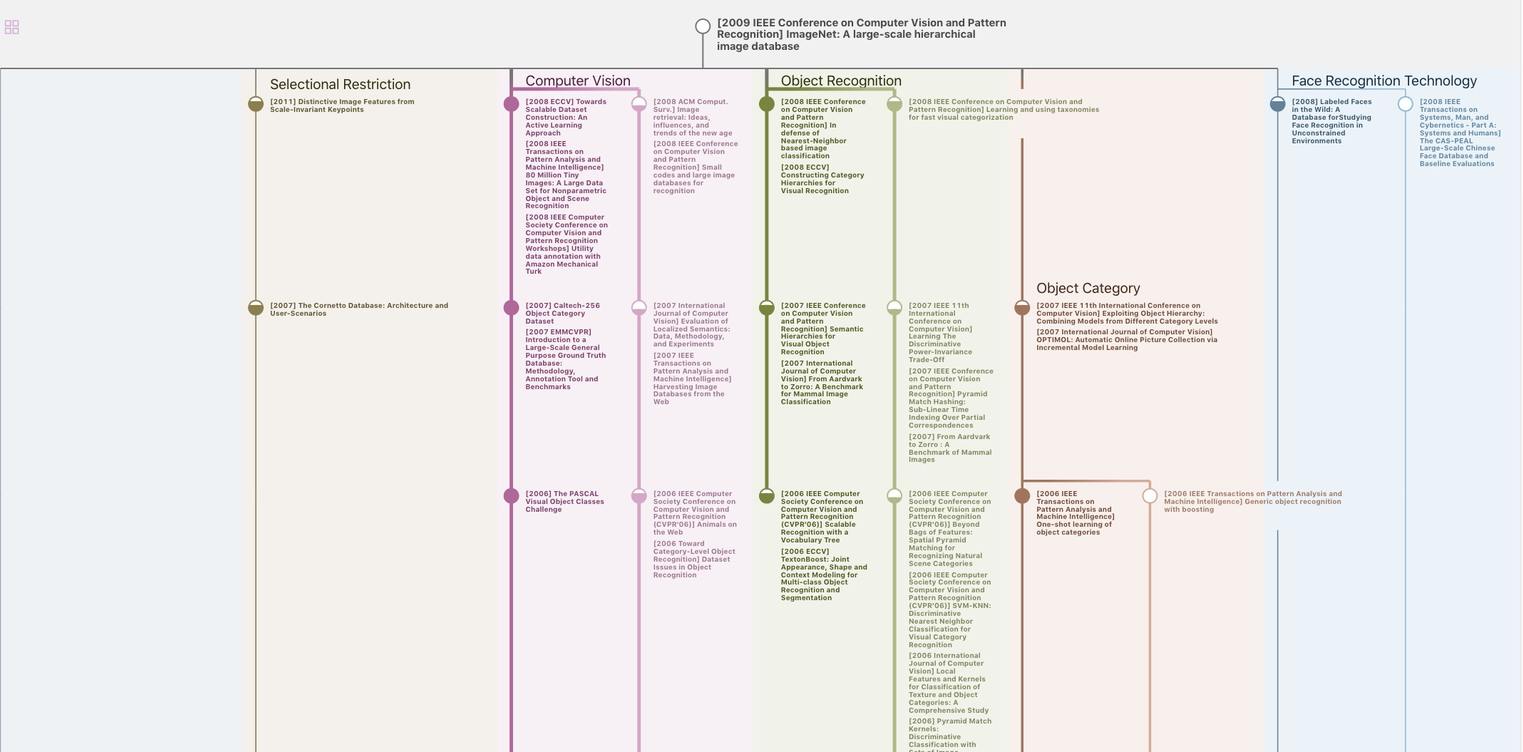
生成溯源树,研究论文发展脉络
Chat Paper
正在生成论文摘要