A Correntropy-Based Classifier for Motor Imagery Brain-Computer Interfaces
Biomedical physics & engineering express(2019)
摘要
Objective. This work aims to present a deeper investigation of the classification performance achieved by a motor imagery (MI) EEG-based brain-computer interface (BCI) using functional connectivity (FC) measures as features. The analysis is performed for two different datasets and analytical setups, including an information-theoretic based FC estimator (correntropy). Approach. In the first setup, using data acquired by our group, correntropy was compared to Pearson and Spearman correlations for FC estimation followed by graph-based feature extraction and two different classification strategies?linear discriminant analysis (LDA) and extreme learning machines (ELMs) - coupled with a wrapper for feature selection in the mu (7-13 Hz) and beta (13-30 Hz) frequency bands. In the second setup, the BCI competition IV dataset 2a was considered for a broader comparison. Main results. For our own database the correntropy / degree centrality / ELM approach resulted in the most solid framework, with overall classification error as low as 5%. When using the BCI competition dataset, our best result provided a performance comparable to those of the top three competitors. Significance. Correntropy was shown to be the best FC estimator in all analyzed situations in the first experimental setup, capturing the signal temporal behavior and being less sensitive to outliers. The second experimental setup showed that the inclusion of different frequency bands can bring more information and improve the classification performance. Finally, our results pointed towards the importance of the joint use of different graph measures for the classification.
更多查看译文
关键词
brain-computer interfaces,information-theoretic learning,correntropy,graph metrics,motor imagery,functional connectivity,graph signal processing
AI 理解论文
溯源树
样例
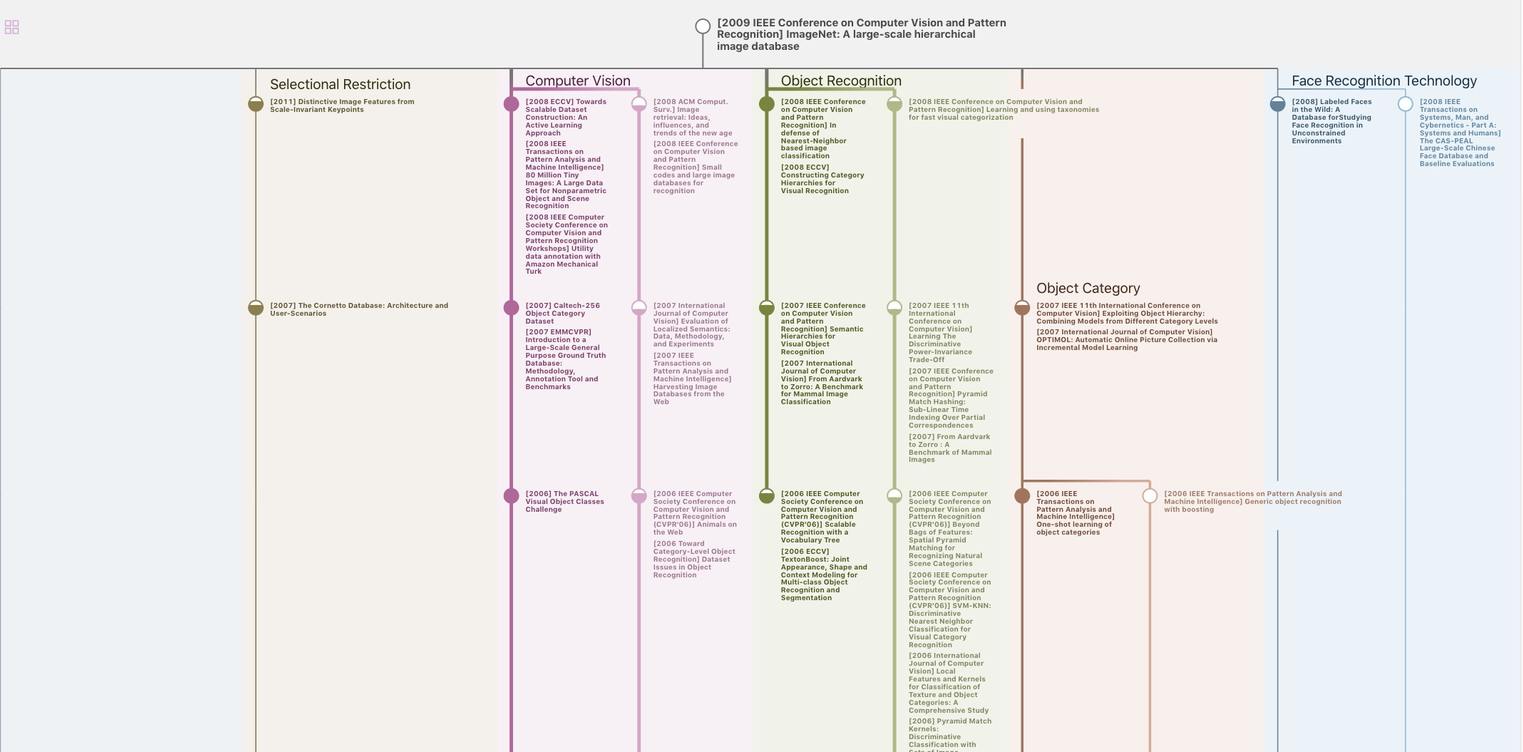
生成溯源树,研究论文发展脉络
Chat Paper
正在生成论文摘要