Effective person re-identification by self-attention model guided feature learning.
Knowledge-Based Systems(2020)
摘要
Person re-identification (re-ID), of which the goal is to recognize person identities of images captured by non-overlapping cameras, is a challenging topic in computer vision. Most existing person re-ID methods conduct directly on detected objects, which ignore the space misalignment caused by detectors, human pose variation, and occlusion problems. To tackle the above mentioned difficulties, we propose a self-attention model guided deep convolutional neural network(DCNN) to learn robust features from image shots. Kernels of the self-attention model evaluate weights for the importance of different person regions. To solve the local feature dependence problem of feature extraction, the non-local feature map generated by the self-attention model is fused with the original feature map generated from the resnet-50. Furthermore, the loss function considers both the cross-entropy loss and the triplet loss in the training process, which enables the network to capture common characteristics within the same individuals and significant differences between distinct persons. Extensive experiments and comparative evaluations show that our proposed strategy outperforms most of the state-of-the-art methods on standard datasets: Market-1501, DukeMTMC-reID, and CUHK03.
更多查看译文
关键词
Person re-identification,Feature extraction,Self-attention,Cross-entropy loss,Triplet loss
AI 理解论文
溯源树
样例
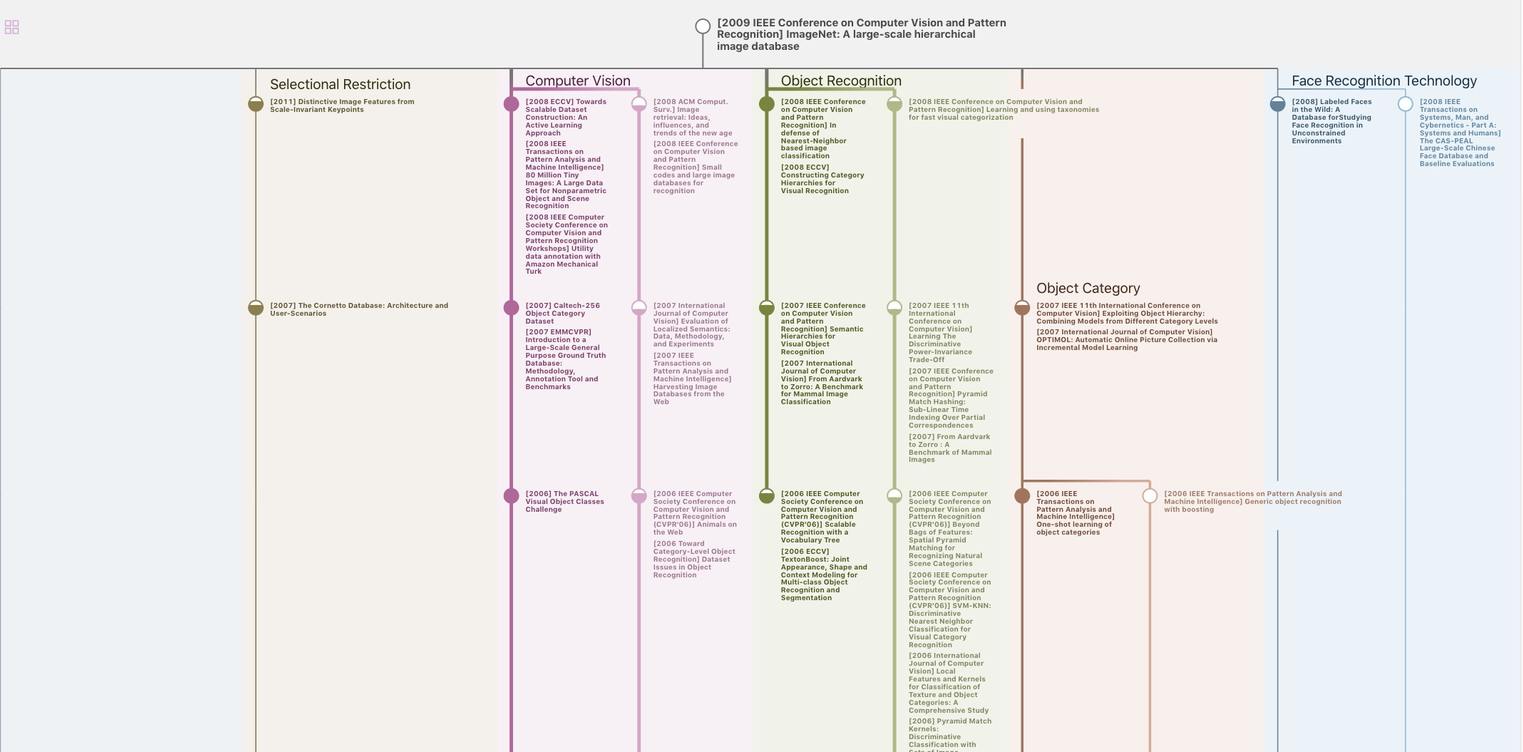
生成溯源树,研究论文发展脉络
Chat Paper
正在生成论文摘要