A novel test-cost-sensitive attribute reduction approach using the binary bat algorithm.
Knowledge-Based Systems(2019)
摘要
Attribute reductions are essential pre-processing steps in such as data mining, machine learning, pattern recognition and many other fields. Moreover, test-cost-sensitive attribute reductions are often used when we have to deal with cost-sensitive data. The main result of this paper is a new meta-heuristic optimization method for finding optimal test-cost-sensitive attribute reduction that is based on binary bat algorithm that originally was designed to model the echolocation behavior of bats when they search their prey. First we provide a 0-1 integer programming algorithm that can calculate optimal reduct but is inefficient for large data sets. We will use it to evaluate other algorithms. Next, a new fitness function that utilizes the pairs of inconsistent objects and does not have any uncertain parameter is design and an efficient algorithm for counting inconsistent pairs is provided. Then, an efficient test-cost-sensitive attribute reduction technique that uses binary bat algorithm is provided. Finally, a new evaluation model with four different evaluation metrics has been proposed and used to evaluate algorithms that only provide sub-optimal solutions. Several experiments were carried out on broadly used benchmark data sets and the results have shown the superiority of our new algorithm, in terms of various metrics, computational time, and classification accuracy, especially for high-dimensional data sets.
更多查看译文
关键词
Rough set,Attribute reduction,Test-cost-sensitive,Binary bat algorithm
AI 理解论文
溯源树
样例
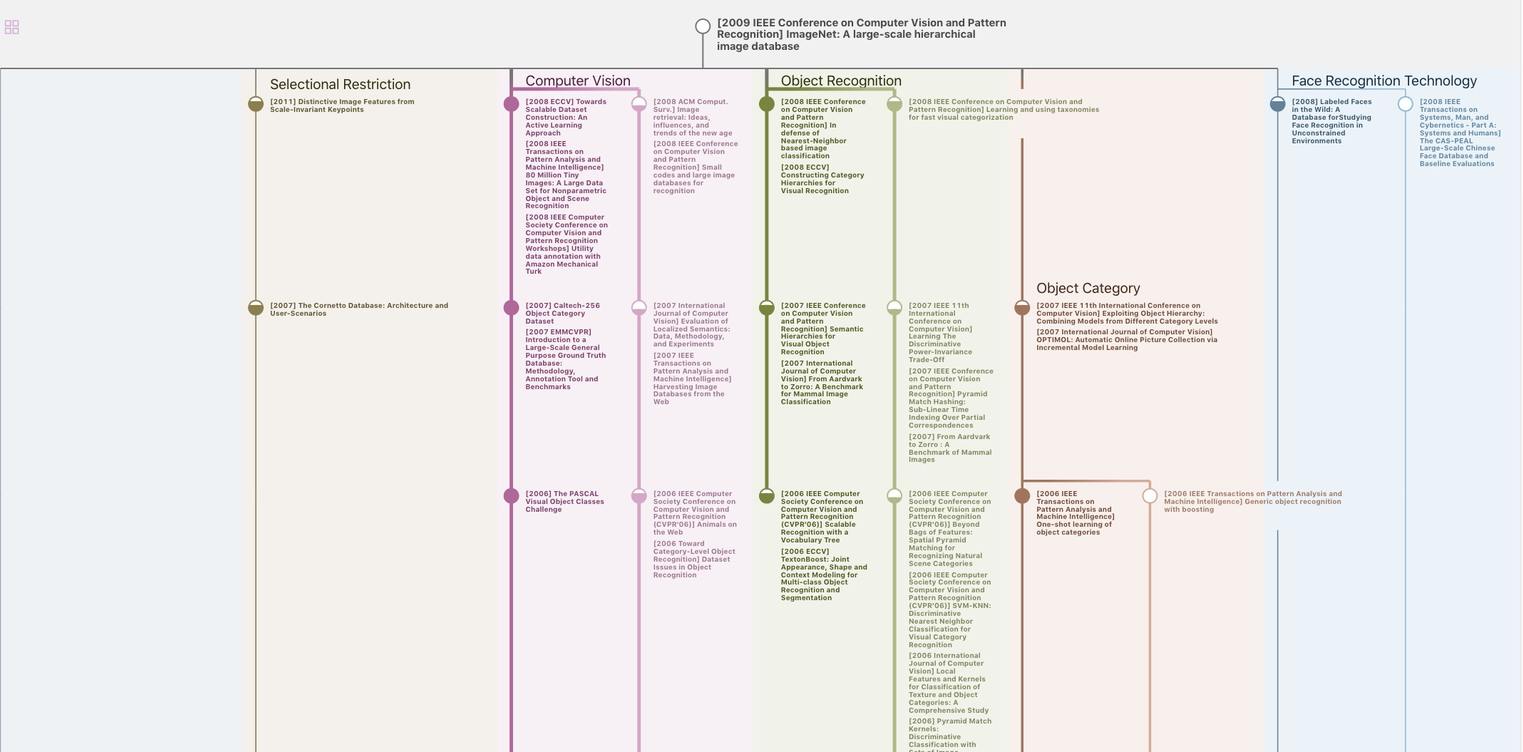
生成溯源树,研究论文发展脉络
Chat Paper
正在生成论文摘要