CURL: Neural Curve Layers for Global Image Enhancement
2020 25TH INTERNATIONAL CONFERENCE ON PATTERN RECOGNITION (ICPR)(2021)
摘要
We present a novel approach to adjust global image properties such as colour, saturation, and luminance using human-interpretable image enhancement curves, inspired by the Photoshop curves tool. Our method, dubbed neural CURve Layers (CURL), is designed as a multi-colour space neural retouching block trained jointly in three different colour spaces (HSV, CIELab, RGB) guided by a novel multi-colour space loss. The curves are fully differentiable and are trained end-to-end for different computer vision problems including photo enhancement (RGB-to-RGB) and as part of the image signal processing pipeline for image formation (RAW-to-RGB). To demonstrate the effectiveness of CURL we combine this global image transformation block with a pixel-level (local) image multi-scale encoder-decoder backbone network. In an extensive experimental evaluation we show that CURL produces state-of-the-art image quality versus recently proposed deep learning approaches in both objective and perceptual metrics, setting new state-of-the-art performance on multiple public datasets. Our code is publicly available at: https://github.com/sjmoran/CURL.
更多查看译文
关键词
global image enhancement,global image properties,human-interpretable image enhancement curves,Photoshop curves tool,multicolour space neural retouching block,computer vision problems,RGB-to-RGB,image signal,RAW-to-RGB,global image transformation block,pixel-level image multiscale encoder-decoder backbone network,image quality,deep learning,multicolour space loss,colour spaces,neural CURve Layers
AI 理解论文
溯源树
样例
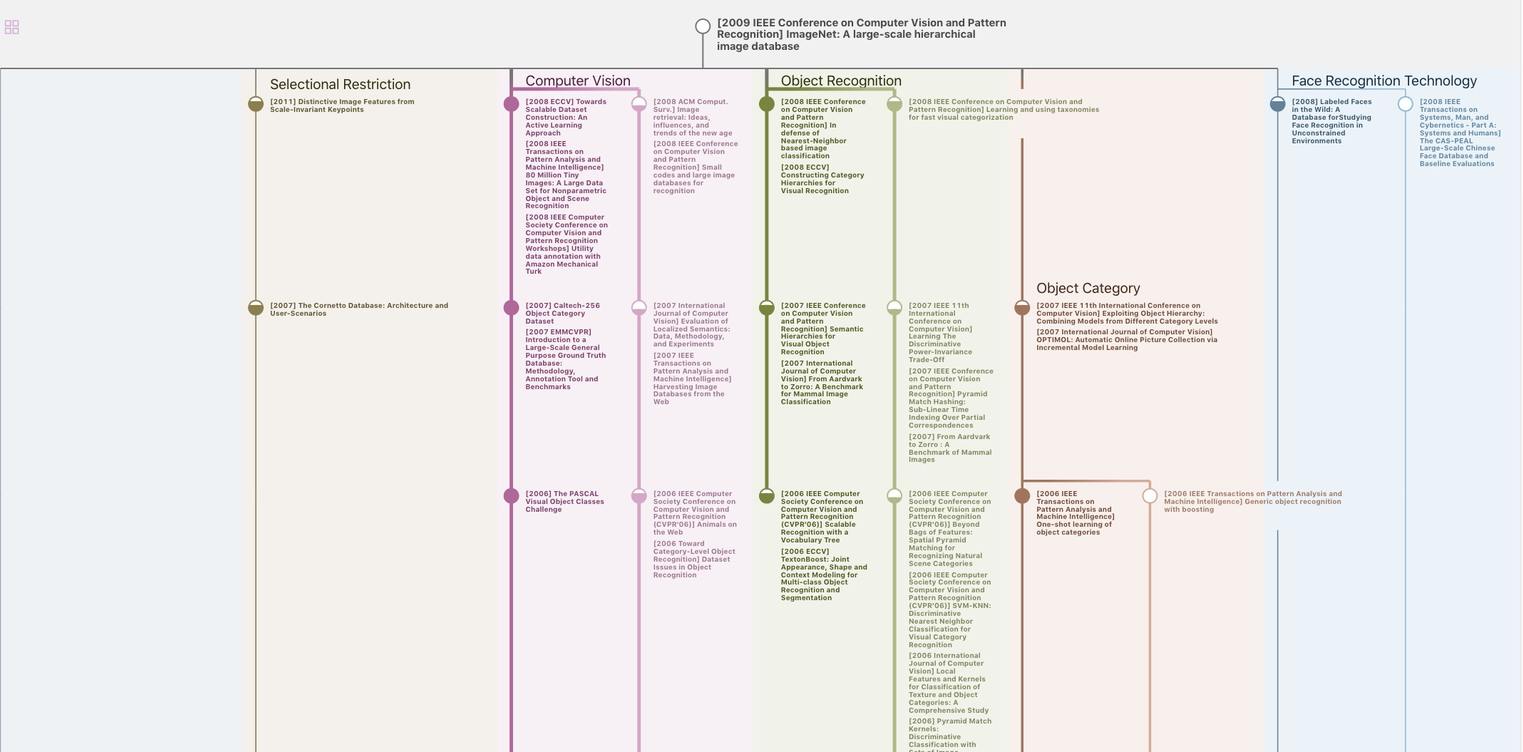
生成溯源树,研究论文发展脉络
Chat Paper
正在生成论文摘要