ReXCam: Resource-Efficient, Cross-Camera Video Analytics at Scale
arxiv(2019)
摘要
Cameras are deployed at scale with the purpose of searching for and tracking objects of interest (e.g. a suspected person) through a live video network. Such cross-camera analytics is compute and data intensive, with cost growing with the number of cameras and time. We present ReXCam, a new low-cost system for efficient cross-camera video analytics. ReXCam exploits spatial and temporal correlations in the dynamics of real camera networks to guide its inference-time search for a query identity. At inference time, ReXCam applies this model (learned from historical videos) to filter frames that are not correlated with the query identity's current position. On rare missed detections, ReXCam performs a fast-replay search on recently filtered video frames and recovers gracefully. ReXCam reduces compute load by 8.3x on an 8-camera dataset, and by 23x to 38x on a simulated 130-camera dataset. We have also implemented and deployed ReXCam on a testbed of 5 AWS DeepLens cameras.
更多查看译文
AI 理解论文
溯源树
样例
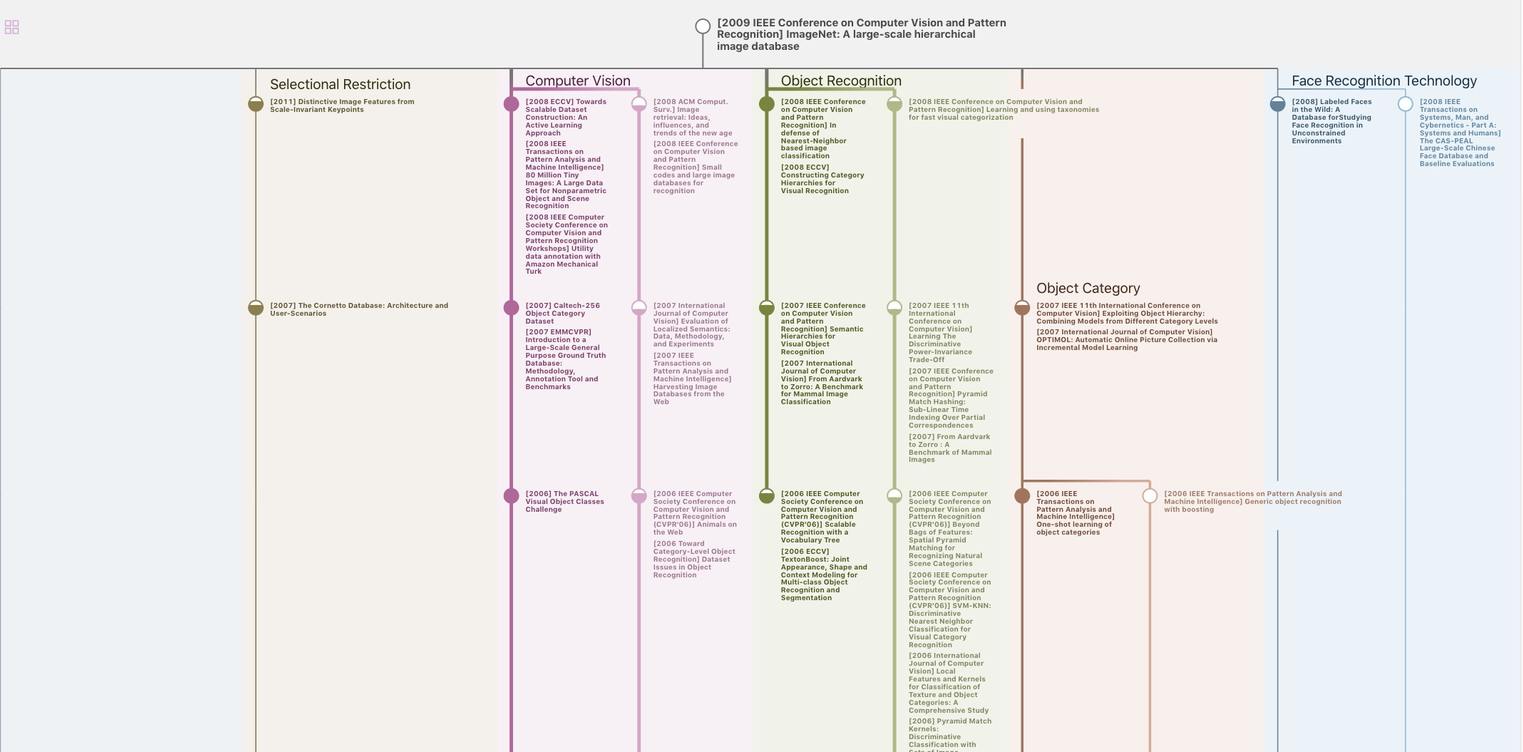
生成溯源树,研究论文发展脉络
Chat Paper
正在生成论文摘要