On the geometry of Stein variational gradient descent
JOURNAL OF MACHINE LEARNING RESEARCH(2023)
摘要
Bayesian inference problems require sampling or approximating high-dimensional probability distributions. The focus of this paper is on the recently introduced Stein variational gradient descent methodology, a class of algorithms that rely on iterated steepest descent steps with respect to a reproducing kernel Hilbert space norm. This construction leads to interacting particle systems, the mean-field limit of which is a gradient flow on the space of probability distributions equipped with a certain geometrical structure. We leverage this viewpoint to shed some light on the convergence properties of the algorithm, in particular addressing the problem of choosing a suitable positive definite kernel function. Our analysis leads us to considering certain nondifferentiable kernels with adjusted tails. We demonstrate significant performance gains of these in various numerical experiments.
更多查看译文
关键词
Bayesian inference,gradient flows,geometry of optimal transport,Stein's method,reproducing kernel Hilbert spaces
AI 理解论文
溯源树
样例
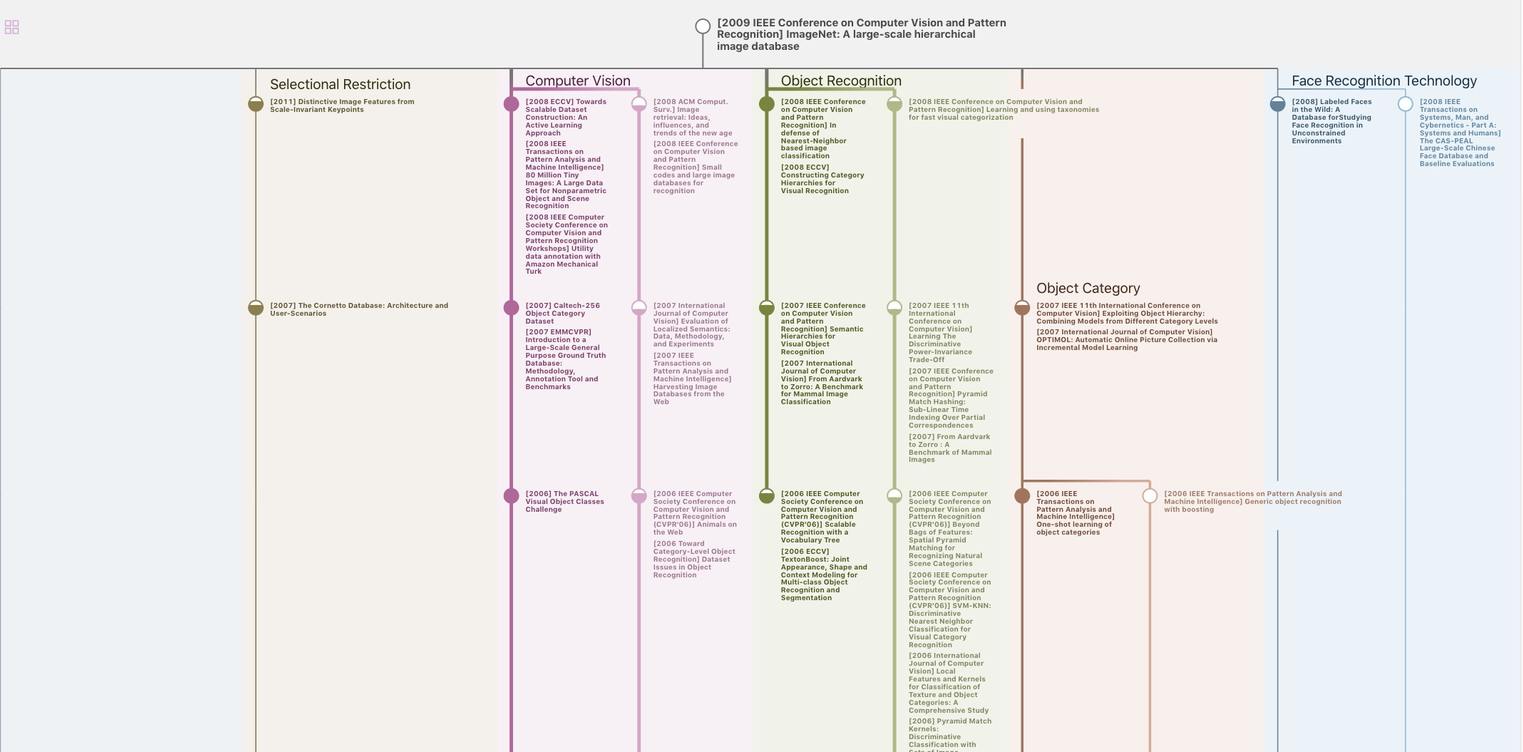
生成溯源树,研究论文发展脉络
Chat Paper
正在生成论文摘要