Real-Time Panoptic Segmentation from Dense Detections
CVPR(2020)
摘要
Panoptic segmentation is a complex full scene parsing task requiring simultaneous instance and semantic segmentation at high resolution. Current state-of-the-art approaches cannot run in real-time, and simplifying these architectures to improve efficiency severely degrades their accuracy. In this paper, we propose a new single-shot panoptic segmentation network that leverages dense detections and a global self-attention mechanism to operate in real-time with performance approaching the state of the art. We introduce a novel parameter-free mask construction method that substantially reduces computational complexity by efficiently reusing information from the object detection and semantic segmentation sub-tasks. The resulting network has a simple data flow that does not require feature map re-sampling or clustering post-processing, enabling significant hardware acceleration. Our experiments on the Cityscapes and COCO benchmarks show that our network works at 30 FPS on 1024x2048 resolution, trading a 3% relative performance degradation from the current state of the art for up to 440% faster inference.
更多查看译文
关键词
time panoptic segmentation,complex full scene parsing task,simultaneous instance,semantic segmentation,single-shot panoptic segmentation network,leverages dense detections,global self-attention mechanism,computational complexity,object detection,parameter-free mask construction method,relative performance degradation,COCO benchmarks,Cityscapes
AI 理解论文
溯源树
样例
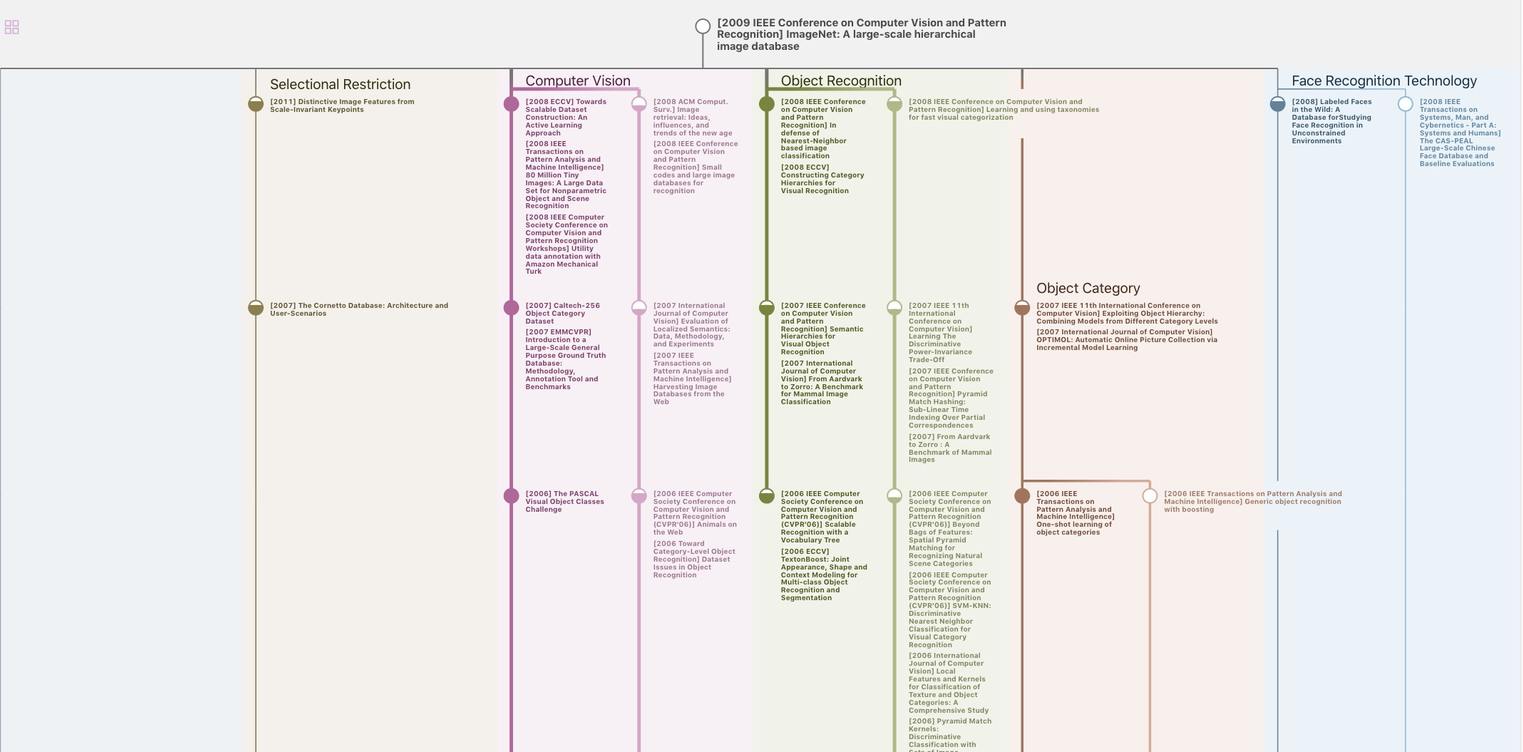
生成溯源树,研究论文发展脉络
Chat Paper
正在生成论文摘要