QuEST: Quantized Embedding Space for Transferring Knowledge
European Conference on Computer Vision(2020)
摘要
Knowledge distillation refers to the process of training a student network to achieve better accuracy by learning from a pre-trained teacher network. Most of the existing knowledge distillation methods direct the student to follow the teacher by matching the teacher’s output, feature maps or their distribution. In this work, we propose a novel way to achieve this goal: by distilling the knowledge through a quantized visual words space. According to our method, the teacher’s feature maps are first quantized to represent the main visual concepts (i.e., visual words) encompassed in these maps and then the student is asked to predict those visual word representations. Despite its simplicity, we show that our approach is able to yield results that improve the state of the art on knowledge distillation for model compression and transfer learning scenarios. To that end, we provide an extensive evaluation across several network architectures and most commonly used benchmark datasets.
更多查看译文
关键词
Knowledge distillation,Transfer learning,Model compression
AI 理解论文
溯源树
样例
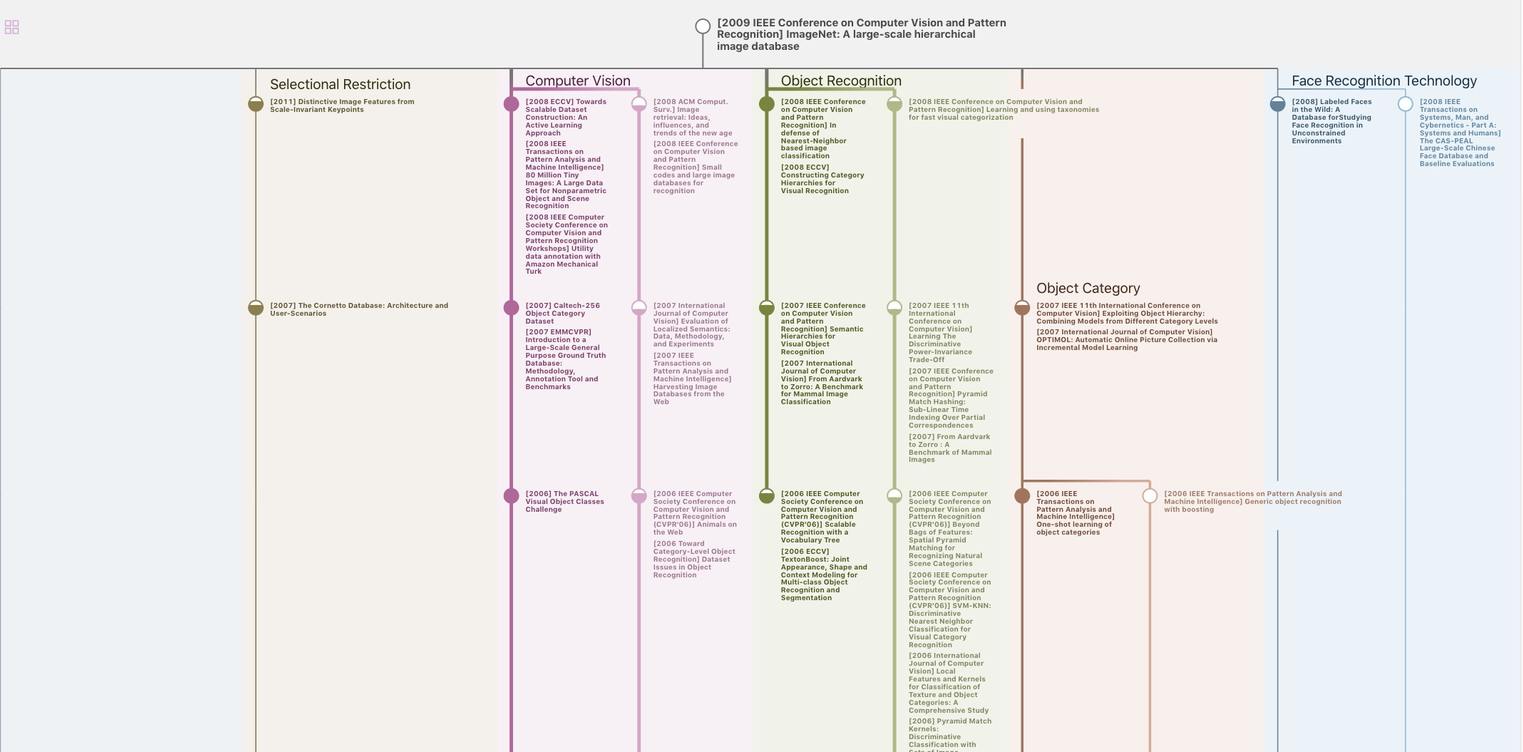
生成溯源树,研究论文发展脉络
Chat Paper
正在生成论文摘要