Challenges in Peptide-Spectrum Matching: a Robust and Reproducible Statistical Framework for Removing Low-Accuracy, High-Scoring Hits.
JOURNAL OF PROTEOME RESEARCH(2020)
摘要
Workflows for large-scale (MS)-based shotgun proteomics can potentially lead to costly errors in the form of incorrect peptide-spectrum matches (PSMs). To improve the robustness of these workflows, we have investigated the use of the precursor mass discrepancy (PMD) to detect and filter potentially false PSMs that have, nonetheless, a high confidence score. We identified and addressed three cases of unexpected bias in PMD results: time of acquisition within a liquid chromatography-mass spectrometry (LC-MS) run, decoy PSMs, and length of the peptide. We created a postanalysis Bayesian confidence measure based on score and PMD, called PMD-false discovery rate (FDR). We tested PMD-FDR on four data sets across three types of MS -based proteomics projects: standard (single organism; reference database), proteogenomics (single organism; customized genomic-based database plus reference), and metaproteomics (microorganism community; customized conglomerate database). On a ground-truth data set and other representative data, PMD-FDR was able to detect 60-80% of likely incorrect PSMs (false-hits) while losing only 5% of correct PSMs (true-hits). PMD-FDR can also be used to evaluate data quality for results generated within different experimental PSM-generating workflows, assisting in method development. Going forward, PMD-FDR should provide detection of high scoring but likely false-hits, aiding applications that rely heavily on accurate PSMs, such as proteogenomics and metaproteomics.
更多查看译文
关键词
peptide-spectrum match,tandem mass spectrometry,precursor mass discrepancy,false discovery rate,statistical analysis,proteogenomics,metaproteomics
AI 理解论文
溯源树
样例
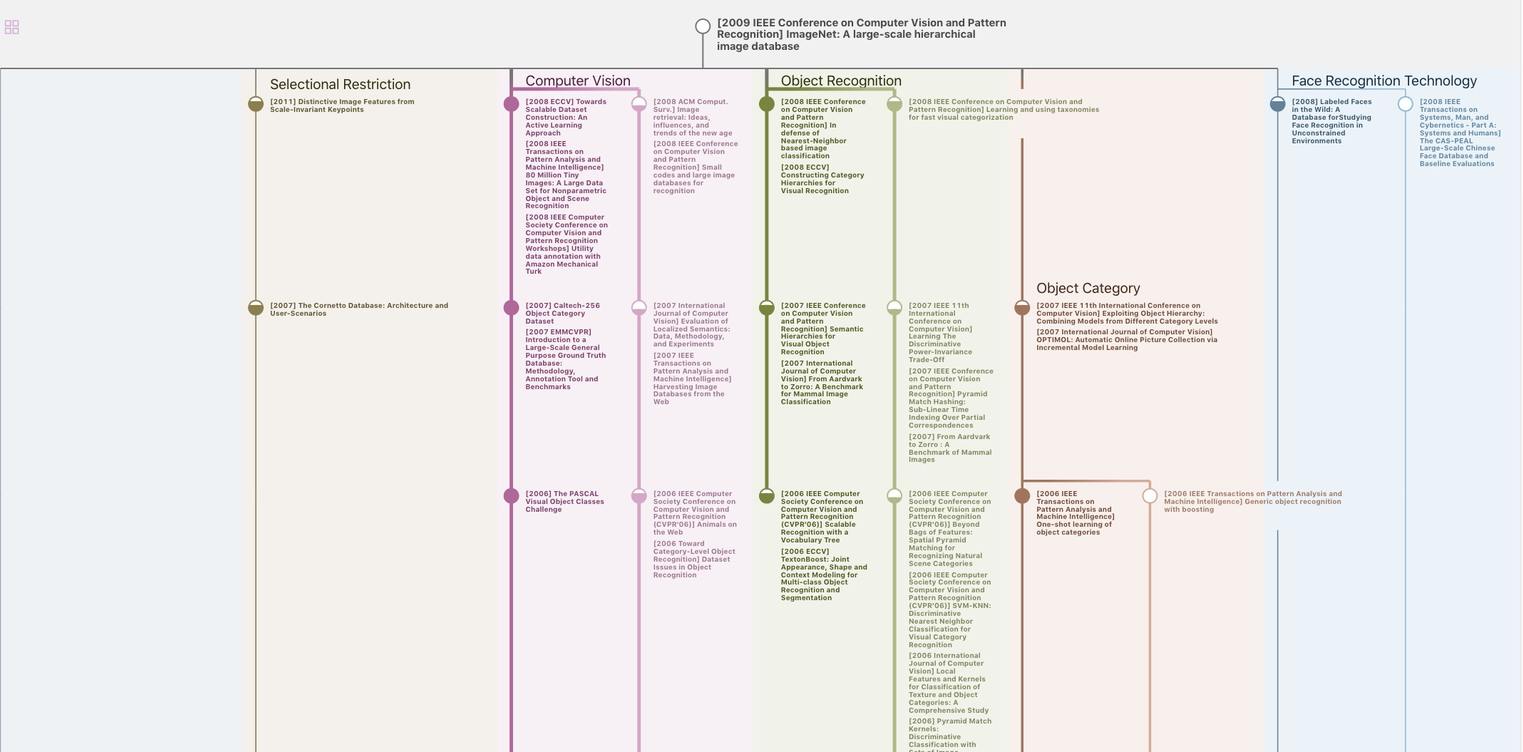
生成溯源树,研究论文发展脉络
Chat Paper
正在生成论文摘要