Personalizing Many Decisions with High-Dimensional Covariates
ADVANCES IN NEURAL INFORMATION PROCESSING SYSTEMS 32 (NIPS 2019)(2019)
摘要
We consider the k-armed stochastic contextual bandit problem with d dimensional features, when both k and d can be large. To the best of our knowledge, all existing algorithms for this problem have regret bounds that scale as polynomials of degree at least two, in k and d. The main contribution of this paper is to introduce and theoretically analyse a new algorithm (REAL-Bandit) with a regret that scales by r(2)(k + d) when r is the rank of the k x d matrix of unknown parameters. REAL-Bandit relies on ideas from low-rank matrix estimation literature and a new row-enhancement subroutine that yields sharper bounds for estimating each row of the parameter matrix that may be of independent interest. We also show via simulations that REAL-Bandit algorithm outperforms existing algorithms that do not leverage the low-rank structure of the problem.
更多查看译文
关键词
many decisions,high-dimensional
AI 理解论文
溯源树
样例
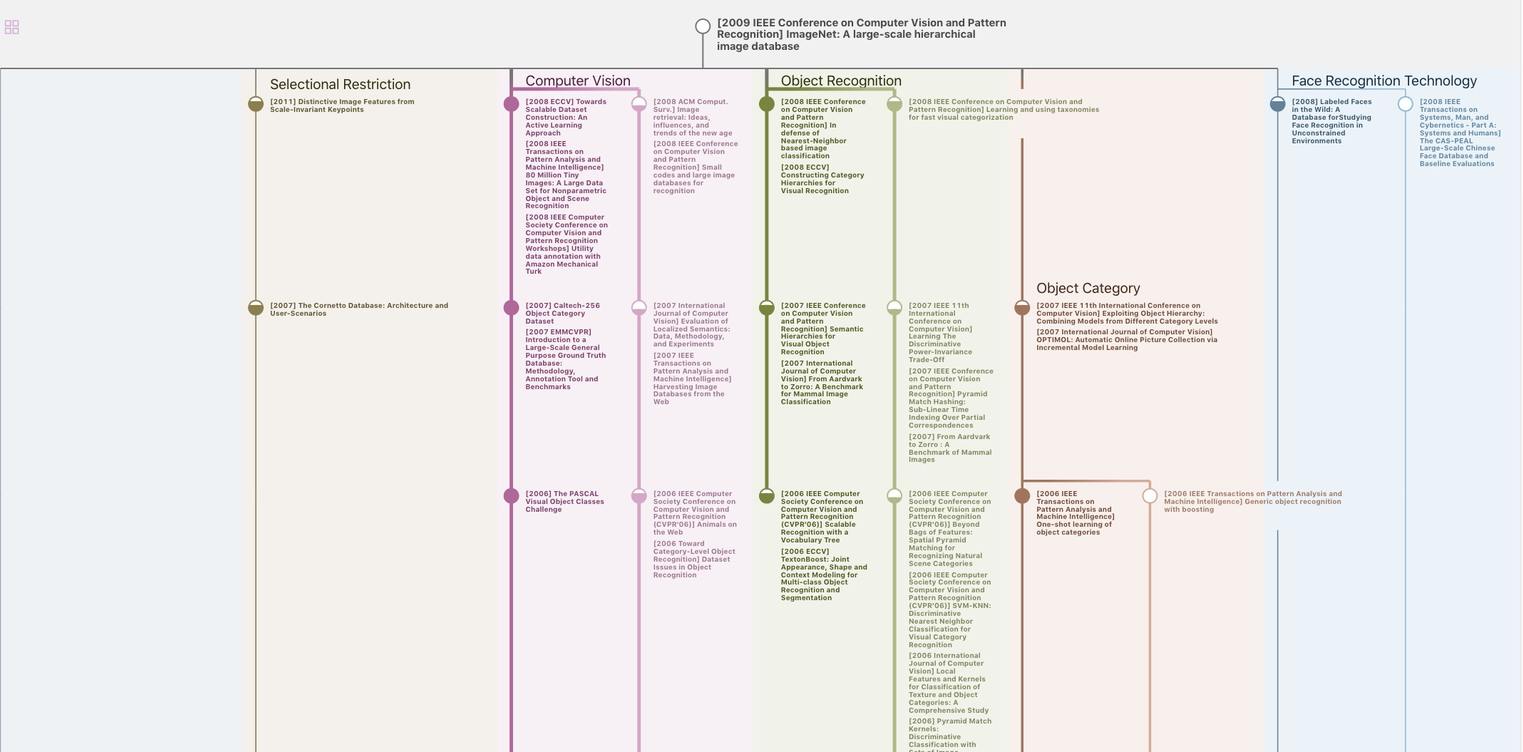
生成溯源树,研究论文发展脉络
Chat Paper
正在生成论文摘要