Active Learning of SVDD Hyperparameter Values
2020 IEEE 7th International Conference on Data Science and Advanced Analytics (DSAA)(2020)
摘要
Support Vector Data Description (SVDD) is a popular one-class classifier, and well-suited for outlier detection. However, the effectiveness of SVDD depends on selecting good hyperparameter values - a difficult problem that has received significant attention in the literature. Since SVDD is an unsupervised classifier, tuning of hyperparameter values is difficult. This has motivated several methods to estimate hyperparameter values based on data characteristics. But existing methods are purely heuristic, and the conditions under which they work well are largely unclear. This has created a situation where instead of selecting hyperparameter values, one has to choose among several, equally plausible heuristics.In this article, we make some strides towards a principled approach to estimate SVDD hyperparameter values. We propose LAMA (Local Active Min-Max Alignment), the first method to select SVDD hyperparameter values by active learning. The core idea is based on kernel alignment, which we adapt to active learning with small sample sizes. LAMA provides estimates for both of the SVDD hyperparameters. These estimates are evidence-based, i.e., rely on actual class labels, and come with a quality score. This eliminates the need for manual validation, an issue with current heuristics. LAMA outperforms state-of-theart competitors in extensive experiments on real-world data. In several cases, LAMA even yields results close to the empirical upper bound.
更多查看译文
关键词
Support Vector Data Description,Active Learning,Outlier Detection
AI 理解论文
溯源树
样例
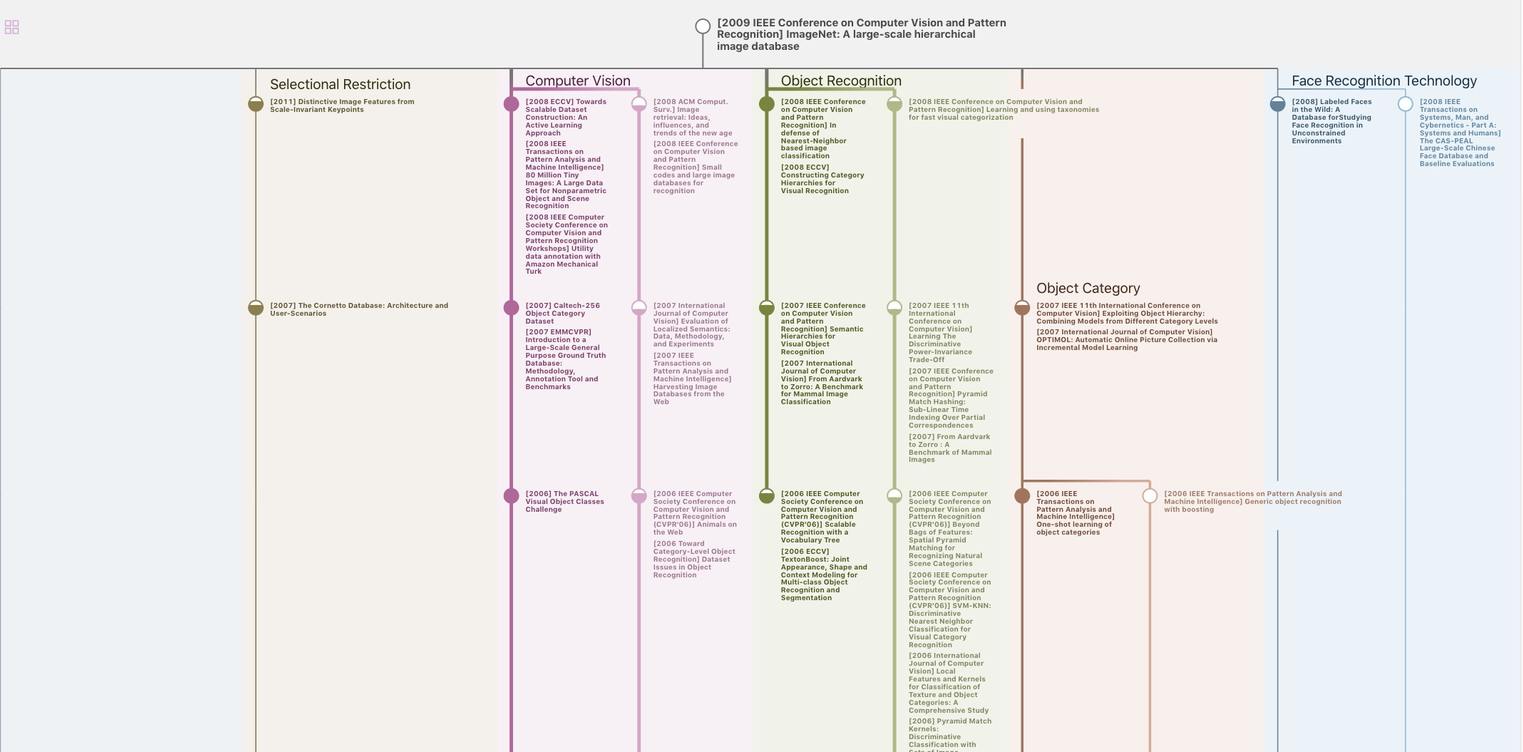
生成溯源树,研究论文发展脉络
Chat Paper
正在生成论文摘要