Learning Multi-Object Tracking And Segmentation From Automatic Annotations
2020 IEEE/CVF CONFERENCE ON COMPUTER VISION AND PATTERN RECOGNITION (CVPR)(2020)
摘要
In this work we contribute a novel pipeline to automatically generate training data, and to improve over state-of-the-art multi-object tracking and segmentation (MOTS) methods. Our proposed track mining algorithm turns raw street-level videos into high-fidelity MOTS training data, is scalable and overcomes the need of expensive and time-consuming manual annotation approaches. We leverage state-of-the-art instance segmentation results in combination with optical flow predictions, also trained on automatically harvested training data. Our second major contribution is MOTSNet- a deep learning, tracking-by-detection architecture for MOTS - deploying a novel mask-pooling layer for improved object association over time. Training MOTSNet with our automatically extracted data leads to significantly improved sMOTSA scores on the novel KITTI MOTS dataset (+1.9%/+7.5% on cars/pedestrians), and MOTSNet improves by +4.1% over previously best methods on the MOTSChallenge dataset. Our most impressive finding is that we can improve over previous best-performing works, even in complete absence of manually annotated MOTS training data.
更多查看译文
关键词
optical flow predictions,deep learning,tracking-by-detection architecture,automatic annotations,track mining algorithm,street-level videos,KITTI MOTS dataset,MOTSNet,object association,instance segmentation,multiobject tracking and segmentation,high-fidelity MOTS training data,mask-pooling layer,sMOTSA scores
AI 理解论文
溯源树
样例
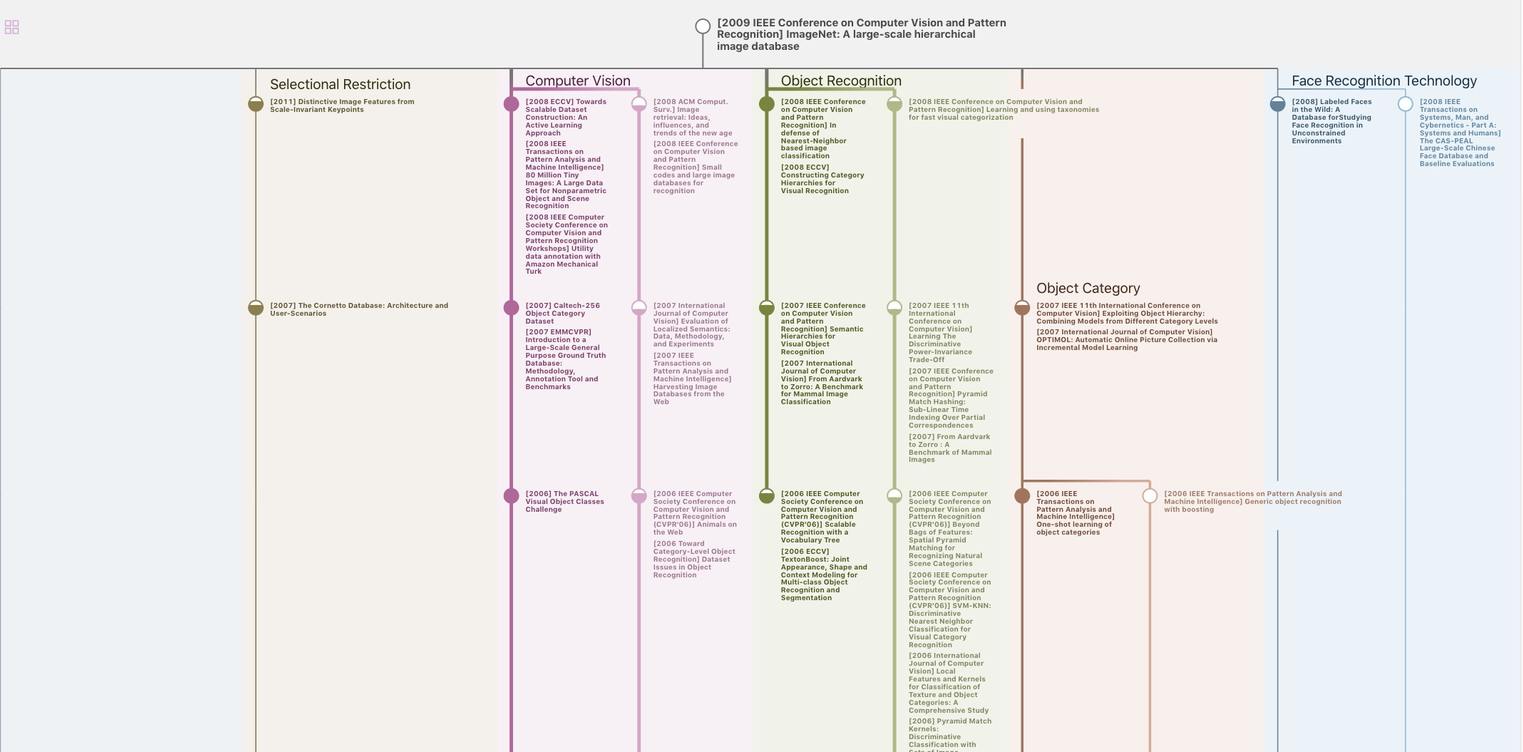
生成溯源树,研究论文发展脉络
Chat Paper
正在生成论文摘要