Assessment of Imputation Methods for Missing Gene Expression Data in Meta-Analysis of Distinct Cohorts of Tuberculosis Patients.
Pacific Symposium on Biocomputing. Pacific Symposium on Biocomputing(2020)
摘要
The growth of publicly available repositories, such as the Gene Expression Omnibus, has allowed researchers to conduct meta-analysis of gene expression data across distinct cohorts. In this work, we assess eight imputation methods for their ability to impute gene expression data when values are missing across an entire cohort of Tuberculosis (TB) patients. We investigate how varying proportions of missing data (across 10%, 20%, and 30% of patient samples) influence the imputation results, and test for significantly differentially expressed genes and enriched pathways in patients with active TB. Our results indicate that truncating to common genes observed across cohorts, which is the current method used by researchers, results in the exclusion of important biology and suggest that LASSO and LLS imputation methodologies can reasonably impute genes across cohorts when total missingness rates are below 20%.
更多查看译文
关键词
meta-analysis, imputation, multi-cohort analysis, cohort-wide imputation
AI 理解论文
溯源树
样例
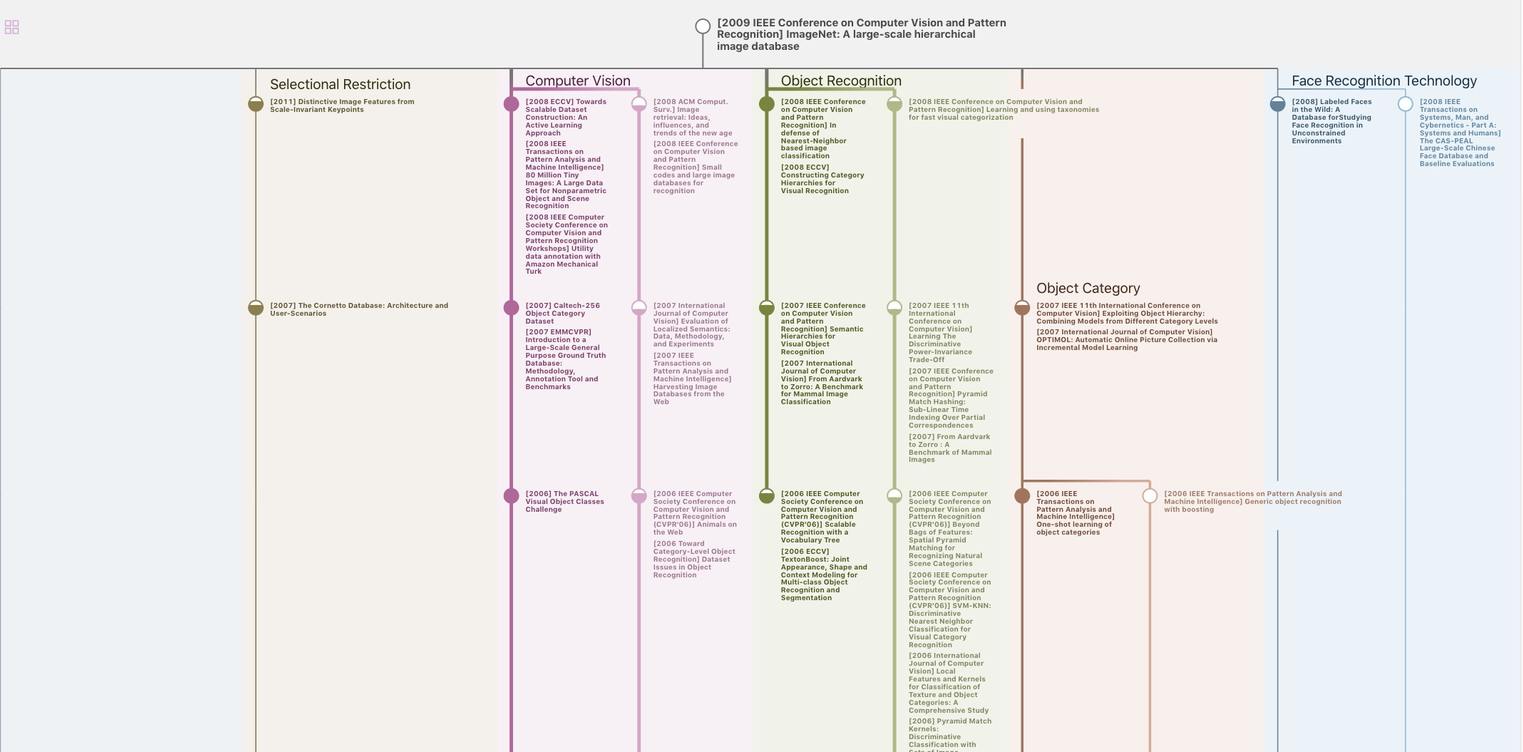
生成溯源树,研究论文发展脉络
Chat Paper
正在生成论文摘要