Diagnosing PTSD using electronic medical records from canadian primary care data
Proceedings of the 6th International Conference on Networking, Systems and Security(2019)
摘要
Post-traumatic stress disorder (PTSD) can be a debilitating condition and early intervention can be instrumental in preventing patients' suffering. Identifying patients at risk for PTSD is challenging because of the limitations of the available data set, variations in the symptoms of PTSD for different patients, and misdiagnosis due to symptoms being shared with other conditions. In this preliminary study, we explore a small set of structured primary care data extracted from patients' electronic medical records (EMR) from Manitoba, Canada. This data has a small subset of PTSD positive cases, and is used to assess the feasibility of applying machine learning algorithms to diagnose PTSD. We developed three supervised machine learning models, a multi-layered perceptron artificial neural network (ANN) model, a support vector machine (SVM), and a random forest classifier (RF) to identify PTSD patients using 890 patients' records. These methods obtained 0.79, 0.78, and 0.83 AUC respectively, which are better than all of the previous work that used EMR data having comparable size as our data. This study is geared towards understanding the primary care standard for PTSD patients in Canada in general and military-veteran population and developing a case definition for PTSD. This initial result demonstrates that an automated PTSD screening tool can be developed based on historical medical data for further study. In our ongoing work, we are exploring the providers' chart notes from the EMR data, which is unstructured text data, to improve the model accuracy and understand the progression of PTSD.
更多查看译文
关键词
PTSD detection, SVM, artificial neural networks, bioinformatics, machine learning, psychology, random forests
AI 理解论文
溯源树
样例
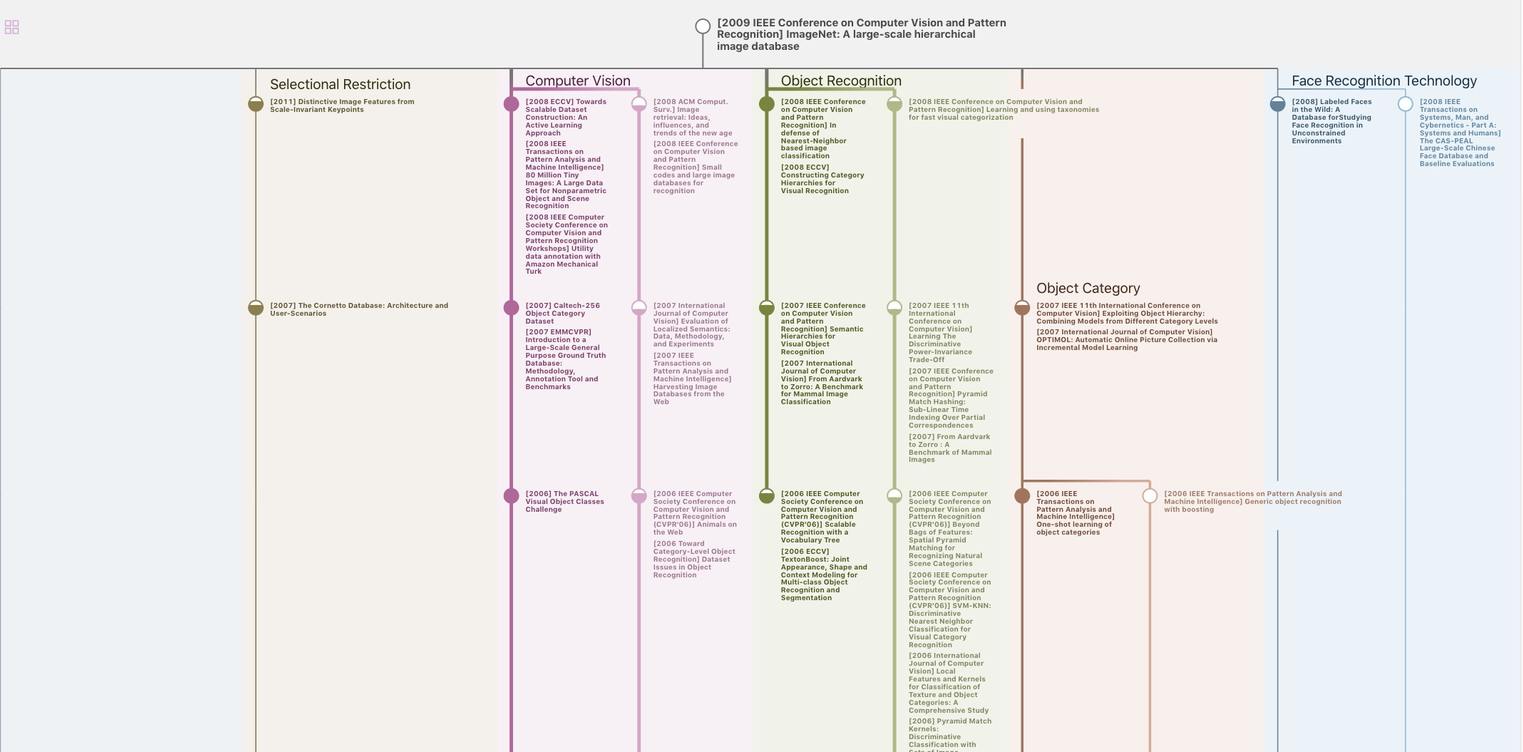
生成溯源树,研究论文发展脉络
Chat Paper
正在生成论文摘要