Semi-Supervised Classification-Based Local Vertex Ranking Via Dual Generative Adversarial Nets
2019 IEEE INTERNATIONAL CONFERENCE ON BIG DATA (BIG DATA)(2019)
摘要
Real-world graphs are usually very sparse in terms of inadequate edges and labels as well as have poor quality due to a large amount of noisy data. In this paper, we propose a classification-based local vertex ranking architecture through dual generative adversarial networks in the semi-supervised setting, DQGAN, for analyzing sparse noisy graphs with rarely labeled data. First, we develop a quadruple generative adversarial ClassNet model to address the noisy data and data sparsity issues as well as to classify each vertex into K classes by automatically creating imaginary/real-looking supplementary labeled vertices with the quite different/similar distributions as real vertices, without the high cost of multi-step graph propagation, heterogeneous graph mining, and iterative weight learning. In addition, the vertex label vicinity is incorporated into the classification model to capture the pairwise vertex closeness based on the labeling and align the vertex label vicinity with the well-known vertex homophily for preserving the original structural semantics in the classification space. Second, we present a quintuple generative adversarial RankNet framework to locally rank each vertex on each of K classes by designing the game of multiple competitors utilizing the mix of real and noisy data to fight against each other, for improving the robustness of local vertex ranking to noisy data with few help from human efforts. The cycle ranking consistency strategy is designed to make the ranking quality verifiable through the bidirectional information-lossless translations between the original features and the ranking features. We propose to utilize the relaxed local PageRank property to produce high-quality local vertex ranking results in the context of information networks. Third but last, extensive evaluation on real graph datasets demonstrates that DQGAN outperforms existing representative methods in terms of both classification and ranking in the semi-supervised setting.
更多查看译文
关键词
noisy data,cycle ranking consistency strategy,ranking features,relaxed local PageRank property,high-quality local vertex,graph datasets,semisupervised classification-based local vertex ranking,real-world graphs,classification-based local vertex ranking architecture,dual generative adversarial networks,sparse noisy graphs,rarely labeled data,quadruple generative adversarial ClassNet model,data sparsity issues,supplementary labeled vertices,multistep graph propagation,heterogeneous graph mining,vertex label vicinity,classification model,pairwise vertex closeness,classification space,quintuple generative adversarial RankNet framework,DQGAN,bidirectional information-lossless translations,information networks
AI 理解论文
溯源树
样例
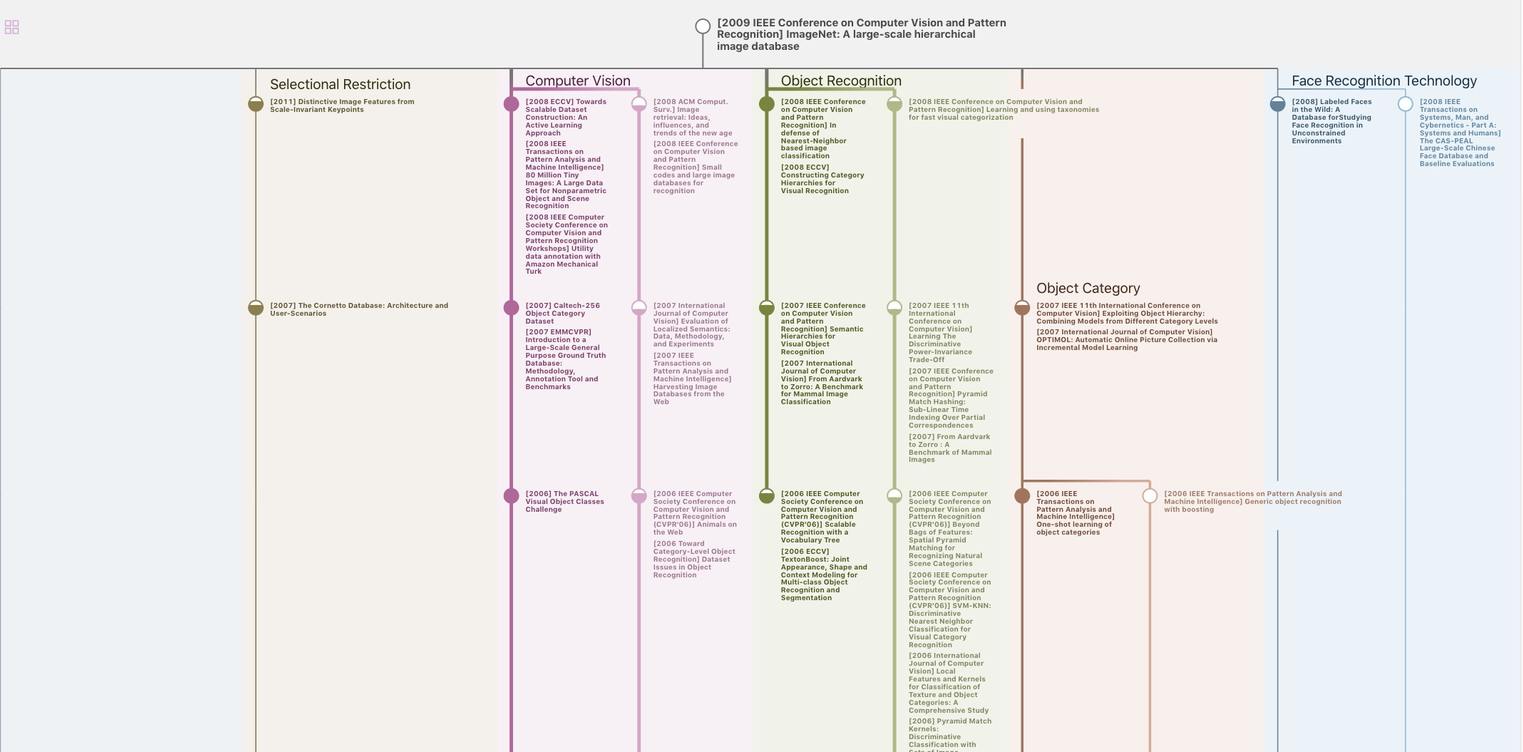
生成溯源树,研究论文发展脉络
Chat Paper
正在生成论文摘要