Intrinsic Topological Transforms via the Distance Kernel Embedding
SoCG(2020)
摘要
Topological transforms are parametrized families of topological invariants, which, by analogy with transforms in signal processing, are much more discriminative than single measurements. The first two topological transforms to be defined were the Persistent Homology Transform and Euler Characteristic Transform, both of which apply to shapes embedded in Euclidean space. The contribution of this paper is to define topological transforms that depend only on the intrinsic geometry of a shape, and hence are invariant to the choice of embedding. To that end, given an abstract metric measure space, we define an integral operator whose eigenfunctions are used to compute sublevel set persistent homology. We demonstrate that this operator, which we call the distance kernel operator, enjoys desirable stability properties, and that its spectrum and eigenfunctions concisely encode the large-scale geometry of our metric measure space. We then define a number of topological transforms using the eigenfunctions of this operator, and observe that these transforms inherit many of the stability and injectivity properties of the distance kernel operator.
更多查看译文
关键词
intrinsic topological transforms,distance kernel
AI 理解论文
溯源树
样例
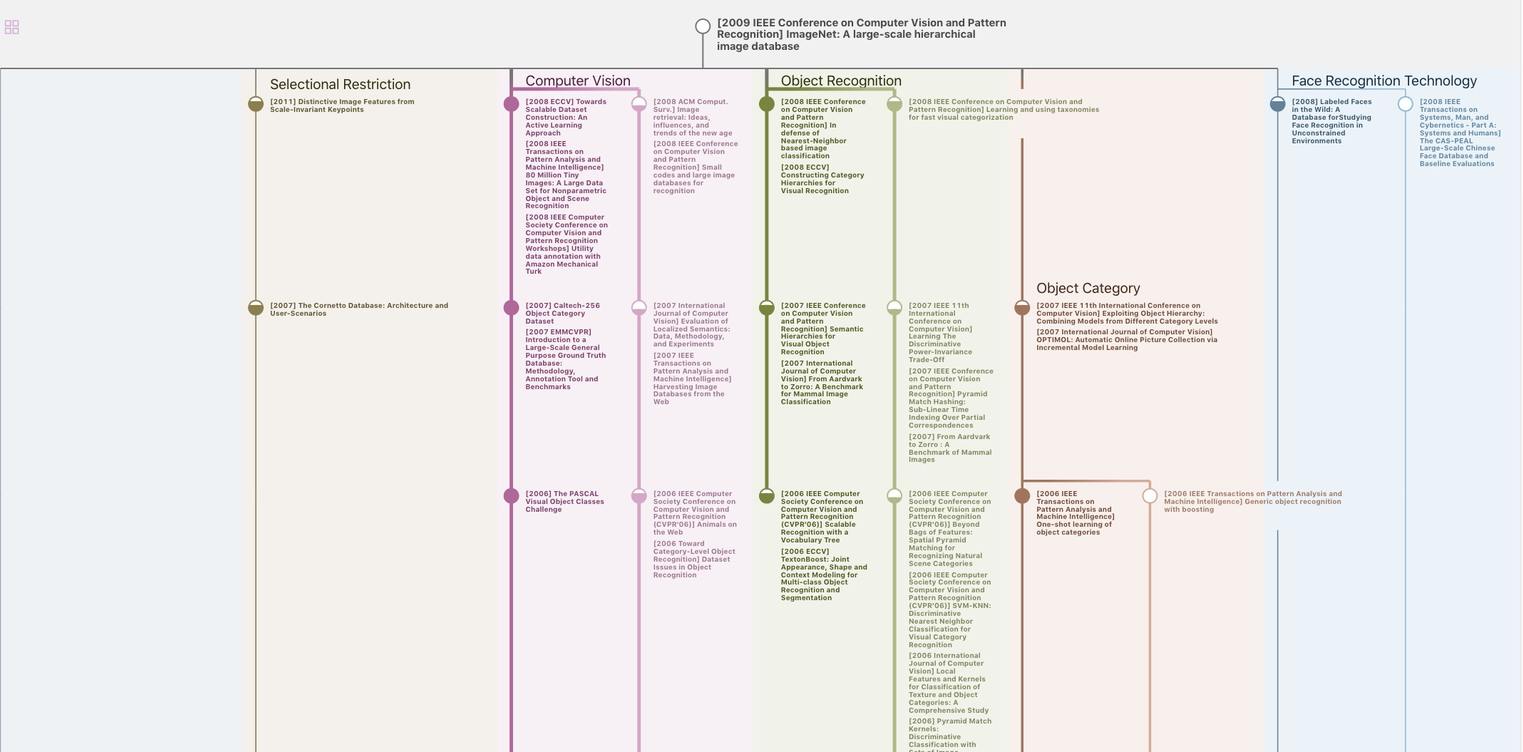
生成溯源树,研究论文发展脉络
Chat Paper
正在生成论文摘要