A feasibility study of deep learning for predicting hemodynamics of human thoracic aorta.
Journal of Biomechanics(2020)
摘要
Numerical analysis methods including finite element analysis (FEA), computational fluid dynamics (CFD), and fluid–structure interaction (FSI) analysis have been used to study the biomechanics of human tissues and organs, as well as tissue-medical device interactions, and treatment strategies. However, for patient-specific computational analysis, complex procedures are usually required to set-up the models, and long computing time is needed to perform the simulation, preventing fast feedback to clinicians in time-sensitive clinical applications. In this study, by using machine learning techniques, we developed deep neural networks (DNNs) to directly estimate the steady-state distributions of pressure and flow velocity inside the thoracic aorta. After training on hemodynamic data from CFD simulations, the DNNs take as input a shape of the aorta and directly output the hemodynamic distributions in one second. The trained DNNs are capable of predicting the velocity magnitude field with an average error of 1.9608% and the pressure field with an average error of 1.4269%. This study demonstrates the feasibility and great potential of using DNNs as a fast and accurate surrogate model for hemodynamic analysis of large blood vessels.
更多查看译文
关键词
Machine learning,Deep neural network,Computational fluid dynamics,Hemodynamic analysis
AI 理解论文
溯源树
样例
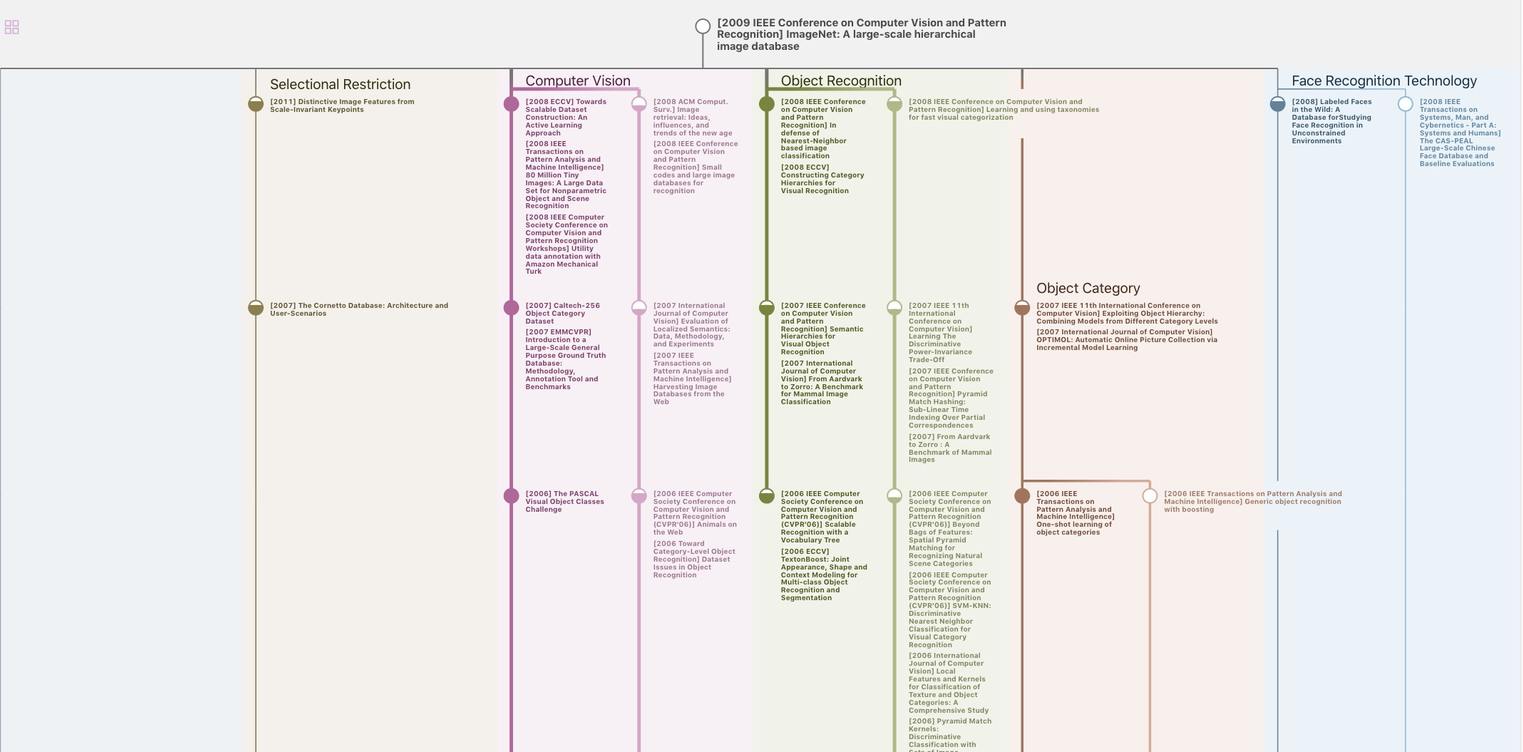
生成溯源树,研究论文发展脉络
Chat Paper
正在生成论文摘要