Knowledge extraction from the learning of sequences in a long short term memory (LSTM) architecture
Knowledge-Based Systems(2022)
摘要
Transparency and trust in machine learning algorithms have been deemed to be fundamental and yet, from a practical point of view, they remain difficult to implement. Particularly, explainability and interpretability are certainly among the most difficult capabilities to be addressed and imply to be able to understand a decision in terms of simple cues and rules. In this article, we address this specific problem in the context of sequence learning by recurrent neuronal models (and more specifically Long Short Term Memory model). We introduce a general method to extract knowledge from the latent space based on the clustering of the internal states. From these hidden states, we explain how to build and validate an automaton that corresponds to the underlying (unknown) grammar, and allows to predict if a given sequence is valid or not. Finally, we show that it is possible for such complex recurrent model, to extract the knowledge that is implicitly encoded in the sequences and we report a high rate of recognition of the sequences extracted from the original grammar. This method is illustrated on artificial grammars (Reber grammar variants) as well as on a real use-case in the electrical domain, whose underlying grammar is unknown.
更多查看译文
关键词
Sequence learning,Knowledge extraction,Long Short Term Memory,Latent space,Implicit learning,Recurrent Neural Networks
AI 理解论文
溯源树
样例
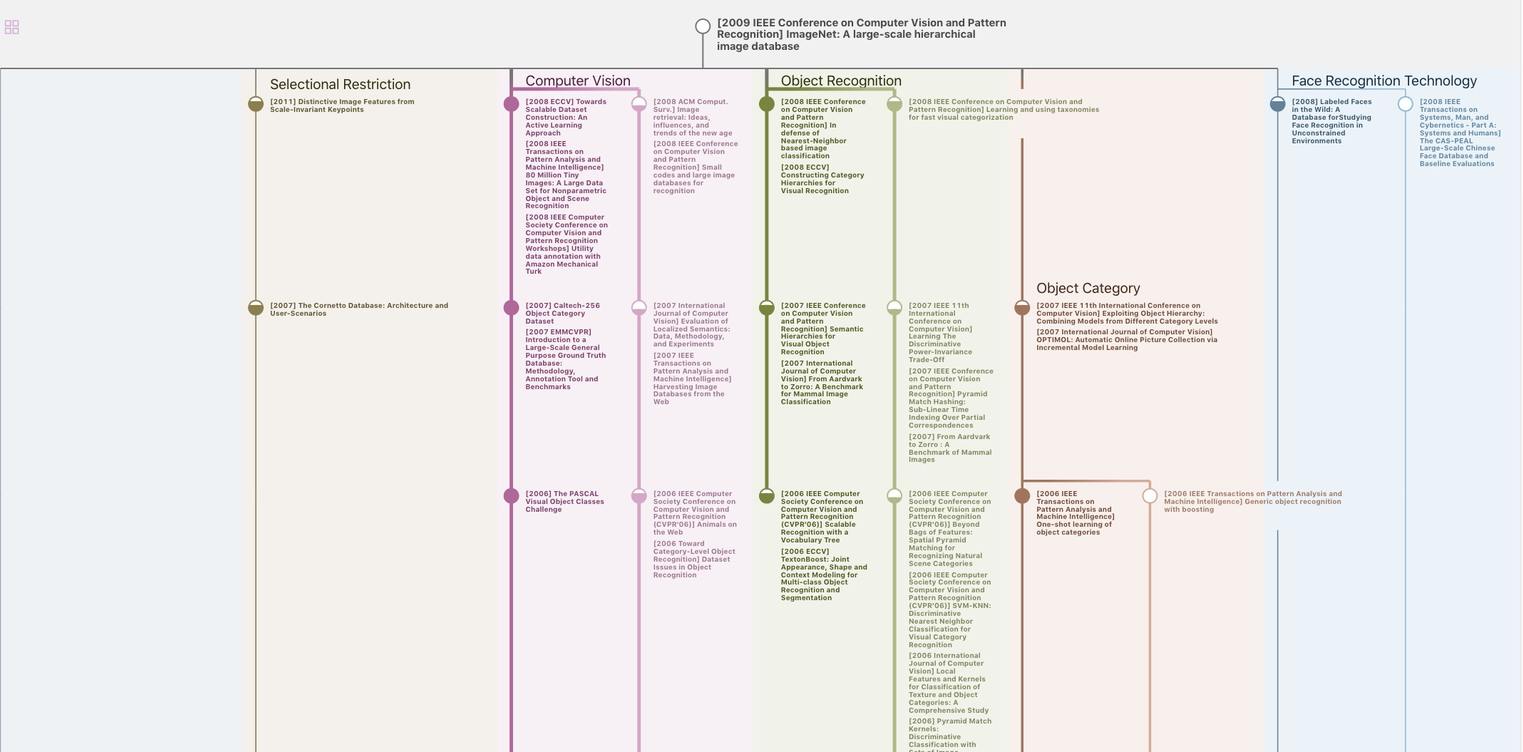
生成溯源树,研究论文发展脉络
Chat Paper
正在生成论文摘要